Short term Traffic Speed Prediction Based on Fundamental and Cointegration Relationship of Speed Density in Non congested and Congested States
IEEE open journal of intelligent transportation systems(2021)
摘要
Short-term traffic state prediction has been an integral part of intelligent transportation systems. Currently, many prediction methods that model traffic dynamics have been proposed. However, only a few of them utilize the relationship among traffic variables represented on the fundamental diagram of traffic flows for prediction. This study proposes traffic speed prediction models based on a threshold error correction model. The proposed model expresses long-run fundamental relationships between speed and density, which differ in non-congested and congested states separated by a speed or density threshold, and the short-run fluctuation of traffic variables that are cointegrated. Using traffic sensor data for more than 300 days on 96 links of arterial roads in Naha City, Japan, the feasibility of the proposed method is evaluated through the analysis of estimated parameters and prediction accuracy. The results confirmed that the proposed method estimates the fundamental speed–density relationship in non-congested and congested states and improves the prediction accuracy using simple threshold error correction models, feed-forward neural networks. The proposed method supports vector regression for most of the links.
更多查看译文
关键词
Cointegration,error correction model,regime-switching model,speed–density relationship,traffic speed prediction
AI 理解论文
溯源树
样例
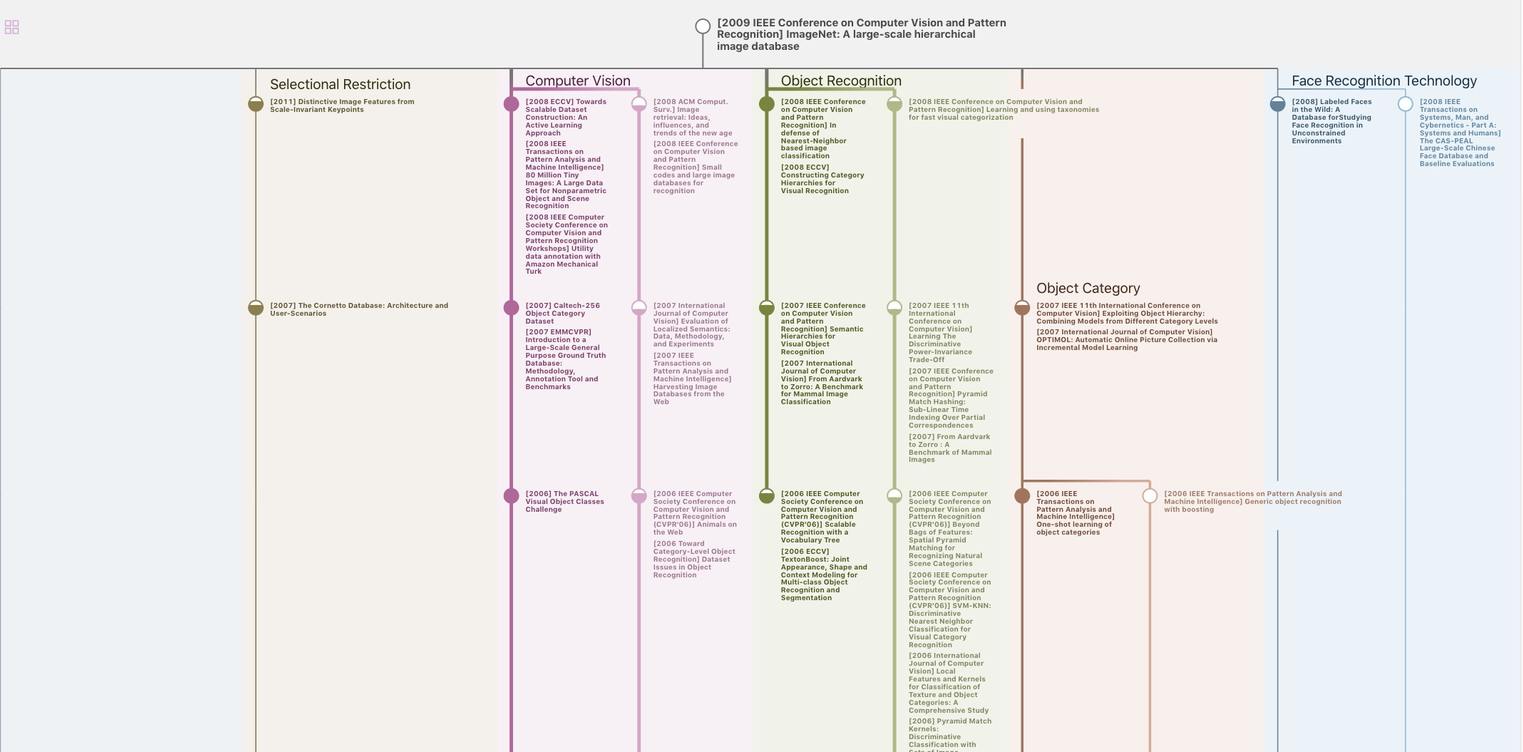
生成溯源树,研究论文发展脉络
Chat Paper
正在生成论文摘要