Non-subsampled Shearlet Entropy and Logistic Regression Based Multiple Sclerosis Detection
2020 2nd International Conference on Advanced Information and Communication Technology (ICAICT)(2020)
摘要
For detection of multiple sclerosis (MS) disease at an early stage in MRI scanning, we introduce a new improved approach based on non-subsampled shearlet entropy (NSSE) and logistic regression (LR) in this paper. Our used dataset includes 676 MRI brain slices of 38 MS affected patients and 880 slices of 34 healthy subjects. In order to balance the grey-level intensity, we applied histogram matching at pre-processing. Non-subsampled shearlet transform (NSST) was performed up to 5 levels for decomposition and non-subsampled shearlet entropy (NSSE) was then implemented for feature extraction from the NSST coefficients sub-bands. Logistic regression (LR) was employed for detection using these NSSE features, conducting k-fold cross validation. Experimental outcomes exhibit that our proposed method obtains 99.12%, 99.77%, 99.50% and 99.41%, sensitivity, specificity, accuracy and f1 score, respectively. Beside comparative analysis proves the supremacy of our proposed approach over five state-of-the-art approaches.
更多查看译文
关键词
Multiple Sclerosis (MS),Non-subsampled Shear-let Transform (NSST),Non-subsampled Shearlet Entropy (NSSE),Logistic Regression (LR),Histogram Matching (HM)
AI 理解论文
溯源树
样例
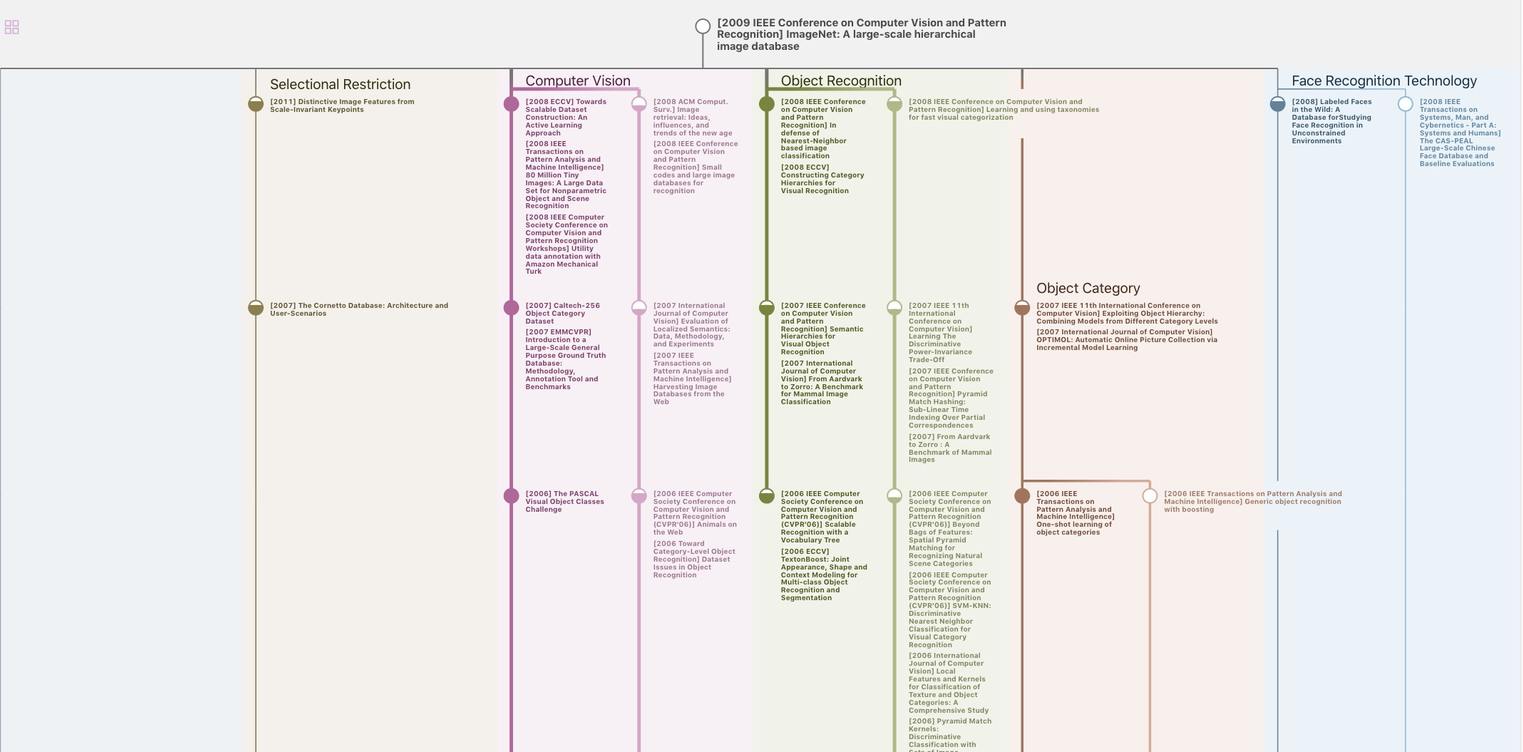
生成溯源树,研究论文发展脉络
Chat Paper
正在生成论文摘要