Triple Factorization-Like Symmetric NLF Models With Latent Item–Item Relationship
IEEE Transactions on Systems, Man, and Cybernetics: Systems(2022)
摘要
Undirected, high-dimension and sparse (SHiDS) networks which are often encountered in industrial applications usually contain numerous useful information; thus, it is practically important to develop effective collaborative filtering methods to rigidly extract the latent information. In order to improve the performance of nonnegative latent factor (NLF) models based on the double factorization while reducing the computation burden of NLF models in terms of the triple factorization (TF) technique, a novel symmetric NLF (SNLF) model, i.e., TF-like SNLF model, is proposed in this article. A latent factor (LF) matrix that reflects the relationship of items is introduced into the objective function so as to offer more freedom for solutions. Then, a fixed weighting matrix designed by sensitivity experiments is used to replace the aforementioned LF matrix dependent on the update, which greatly helps save the time cost without sacrificing any accuracy. Furthermore, the alteration direction (ADI) method is adopted to design the algorithm, which effectively helps reduce the occurrence of the local minimum while guaranteeing convergence. Finally, experimental results on four real industrial data sets demonstrate that the proposed TF-like SNLF model makes a good compromise between the model performance and the time efficiency.
更多查看译文
关键词
Alteration direction (ADI),fixed weighting matrix,high-dimension and sparse networks,latent factor (LF),triple factorization (TF)-like symmetric NLF (SNLF) model,undirected
AI 理解论文
溯源树
样例
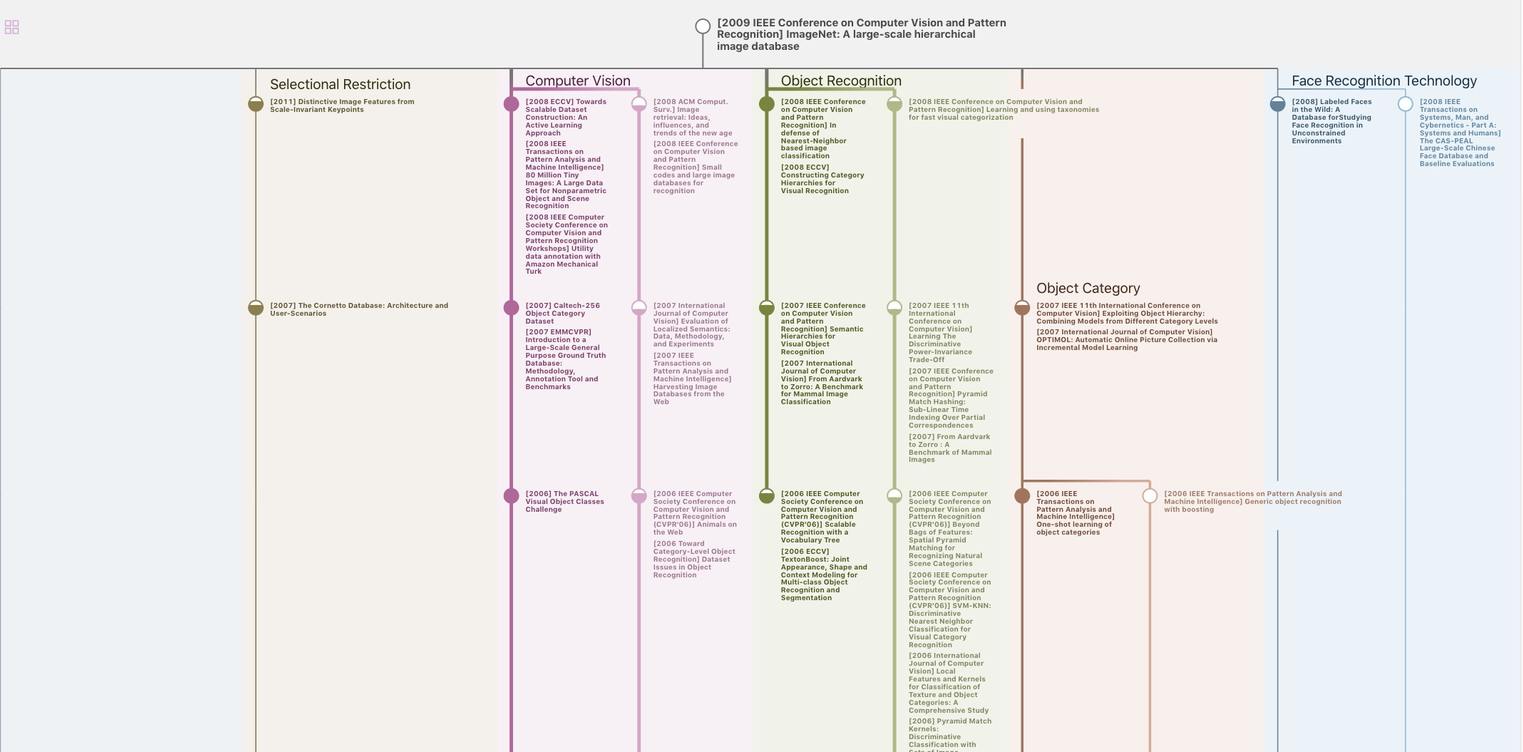
生成溯源树,研究论文发展脉络
Chat Paper
正在生成论文摘要