TNE: A General Time-Aware Network Representation Learning Framework for Temporal Applications
Knowledge-based systems(2022)
Abstract
Temporal dynamics such as short term and long term effects, recency effects, periodic and seasonal temporal factors in information networks are of great importance for many real-world applications. However, existing network embedding learning approaches mainly focus on semantic information or temporal phenomenon such as recency or dynamic process. They failed to have the capability of incorporating multiple temporal factors/phenomenon in information networks. To bridge the gap, this paper proposes a general time-aware network representation learning framework TNE for temporal applications. TNE contains a temporally annotated network TAN, a temporally annotated meta-path based random walk method, and a self-supervised embedding learning approach. We introduce temporal nodes and relations to existing information networks to construct TAN that can incorporate multiple temporal factors. We propose a temporally annotated meta-path based random walk approach to form a time-aware hybrid neighbourhood context that considers both semantic and temporal factors. Based on the time-aware context, self-supervised representation learning approaches are used to simultaneously preserve both semantic and temporal factors in embeddings. Extensive experiments of two large scale real-life datasets show that the proposed framework is effective in various temporal applications such as temporal similarity search and temporal recommendations.
MoreTranslated text
Key words
Representation learning,Heterogeneous information network,User profiling,Network embeddings,Temporal dynamics
AI Read Science
Must-Reading Tree
Example
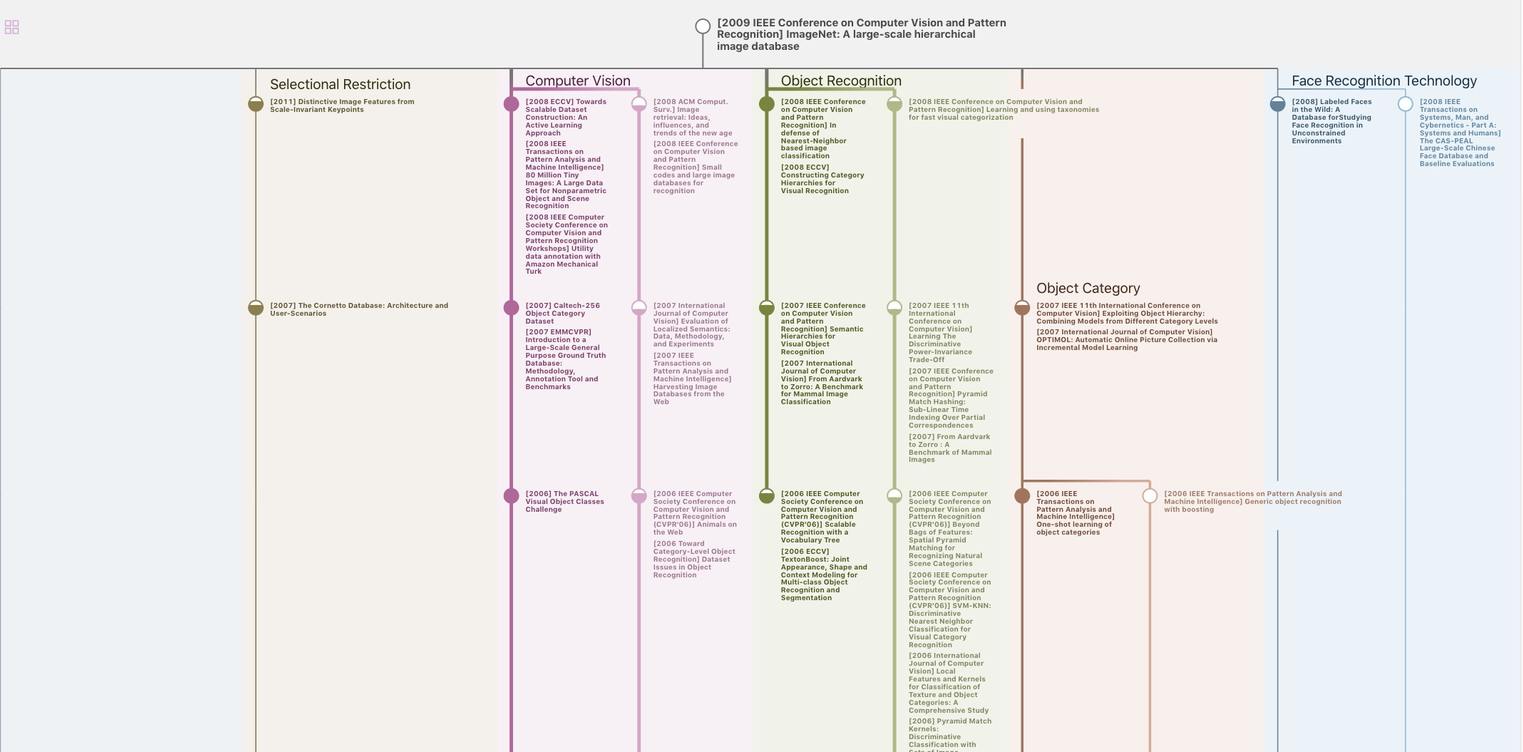
Generate MRT to find the research sequence of this paper
Chat Paper
Summary is being generated by the instructions you defined