Deep Convolutional Networks With Tunable SpeedAccuracy Tradeoff for Human Activity Recognition Using Wearables
IEEE TRANSACTIONS ON INSTRUMENTATION AND MEASUREMENT(2022)
摘要
Activity recognition plays a critical role in various applications, such as medical monitoring and rehabilitation. Deep learning has recently made great development in the wearable-based human activity recognition (HAR) area. However, real HAR applications should be adaptive and flexible to the available computational budget. So far, this problem has rarely been explored. In contrast to existing deep HAR studies focusing on static networks, this article aims to investigate adaptive networks, which can adjust their structure conditioned on available computing resources to trade off between accuracy and speed. We, for the first time, present an adaptive convolutional neural network by dynamically modifying network width. Specifically, first, instead of a normal convolution, the network is stacked by lower triangular convolutional layers in order to remove the impact of activation statistics caused by varying widths. Second, instead of fixed sampling, we perform random sampling over width, which can provide smooth control for the tradeoff between accuracy and speed. As a consequence, the networks with different widths are simultaneously trained as subnetworks by accumulating their losses during each iteration. On multiple HAR datasets, such as UCI-HAR, PAMAP2, and OPPORTUNITY, extensive experiments verify that the proposed approach can consistently provide further improved efficiency on top of state-of-the-art CNNs for HAR. Finally, evaluations are conducted on a Raspberry Pi platform to demonstrate its usefulness and practicality.
更多查看译文
关键词
Computational modeling,Convolution,Adaptation models,Wearable computers,Convolutional neural networks,Adaptive systems,Switches,Activity recognition,convolutional neural networks (CNNs),deep learning,sensor,wearable device
AI 理解论文
溯源树
样例
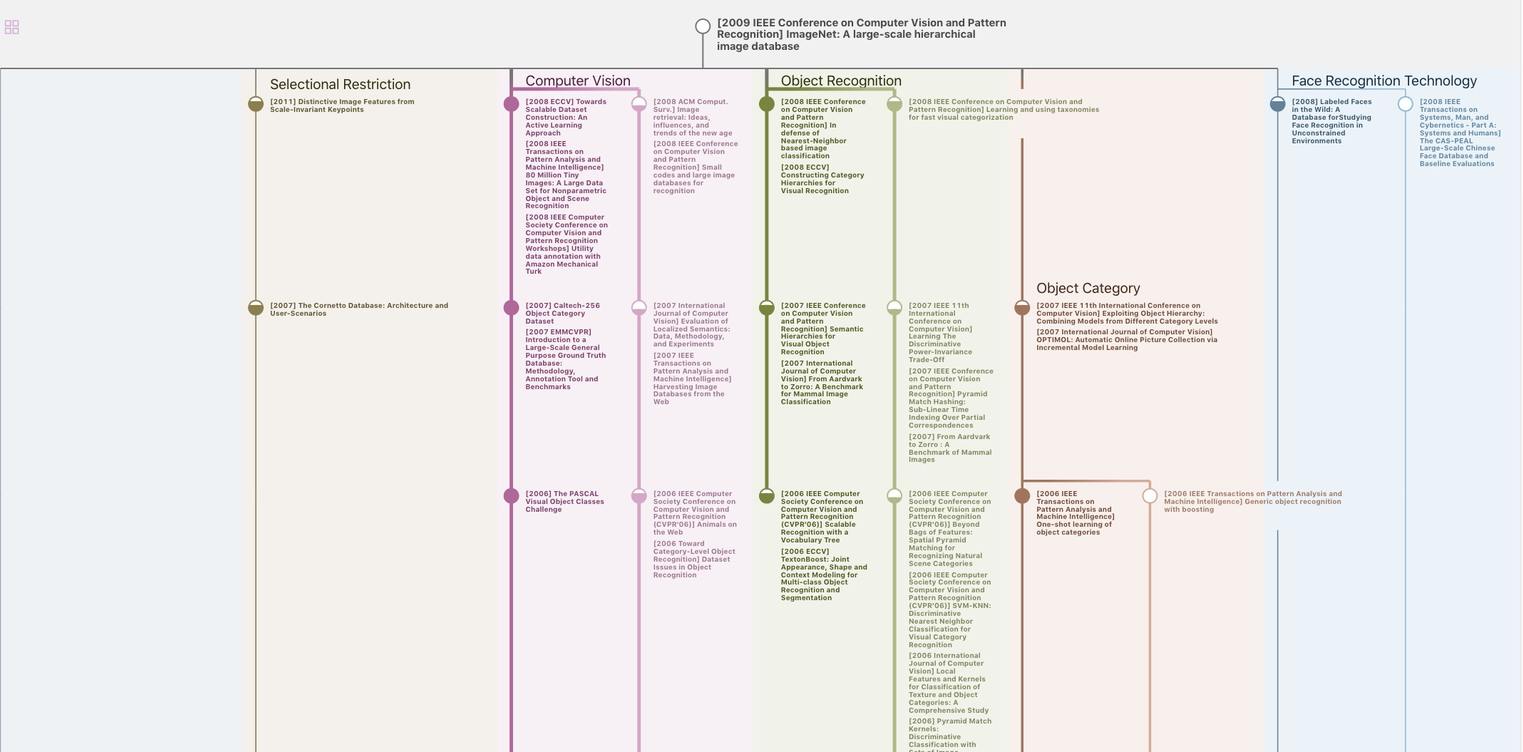
生成溯源树,研究论文发展脉络
Chat Paper
正在生成论文摘要