Predicting fatigue life of metal LPBF components by combining a large fatigue database for different sample conditions with novel simulation strategies
ADDITIVE MANUFACTURING(2022)
摘要
This study presents a unique methodology to systematically predict and evaluate the fatigue behaviour of additively manufactured components. A multitude of factors influence the fatigue performance of end-use application components. To analyse this effect, fatigue behaviour of samples manufactured by laser powder bed fusion (LPBF) is evaluated for different process conditions, namely, build orientation, heat treatment and surface treatment. An extensive experimental test campaign is performed to develop a database of fatigue results capturing all these influencing factors. A Gaussian process is then trained with the observed experimental results to predict the fatigue behaviour for unknown process conditions. This machine learning method is subsequently validated to confirm its capability to represent the experimental data on one hand and to generalize well to unseen data on the other hand. In addition, the method is integrated in a fatigue durability prediction solver. The predictive capability of large-scale simulations was demonstrated for low pressure steam turbine blades manufactured by LPBF and subjected to different combinations of post processes. Subsequent experimental fatigue testing of the blades indicated that the durability solver accurately predicted the crack initiation location. For a combination of stress relieved microstructure and as-fabricated surface, the experimental fatigue lives lie within 95% confidence interval of the lifetimes predicted by the durability solver. For the components with full annealed microstructure and machined surface, the durability solver predicted conservative lifetimes compared to the experimental fatigue lives, demonstrating the capability to predict fatigue behaviour of end-use components in the field.
更多查看译文
关键词
Laser powder bed fusion,Fatigue,Metals,Machine learning
AI 理解论文
溯源树
样例
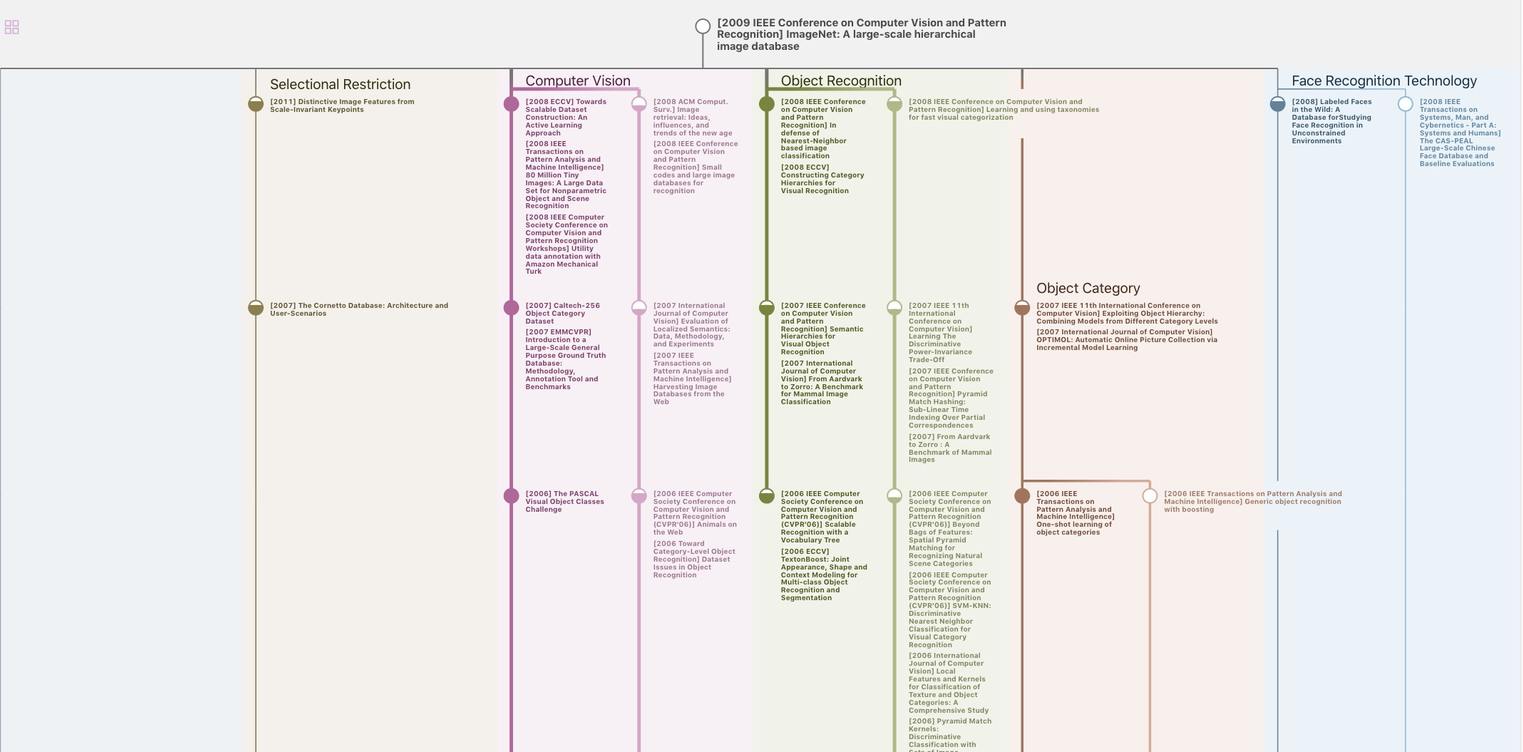
生成溯源树,研究论文发展脉络
Chat Paper
正在生成论文摘要