Machine Learning Tools Set for Natural Gas Fuel Cell System Design
ECS transactions(2021)
摘要
This study is focusing on leveraging the system design tools set for next-generation solid oxide fuel cell (SOFC) based natural gas fuel cell (NGFC) system. Conventionally, system design and optimization of NGFC systems rely heavily on traditional reduced order model (ROM) techniques and designers’ experience level. For overcoming the technical barriers of system design, multiple multi-physics models and machine learning (ML) tools have been utilized to automate the conceptual design process and enhance the reliability of solutions for the NGFC system. The proposed tools set includes a physics-informed ML tool for automated ROM construction that leverages advances in deep neural networks to significantly reduce ROM prediction error for the NGFC power island compared to traditional approaches. The constructed physics-informed ML ROM can be used in system design and optimization tools set Institute for the Design of Advanced Energy Systems (IDAES) Process Systems Engineering (PSE) framework. The tools set also provides user-friendly graphic user interface built within Jupyter Notebooks, and the whole tools set is open-source public available.
更多查看译文
关键词
Solid Oxide Fuel Cells,Fuel Cell Technology,Model Reduction,Direct Methanol Fuel Cells
AI 理解论文
溯源树
样例
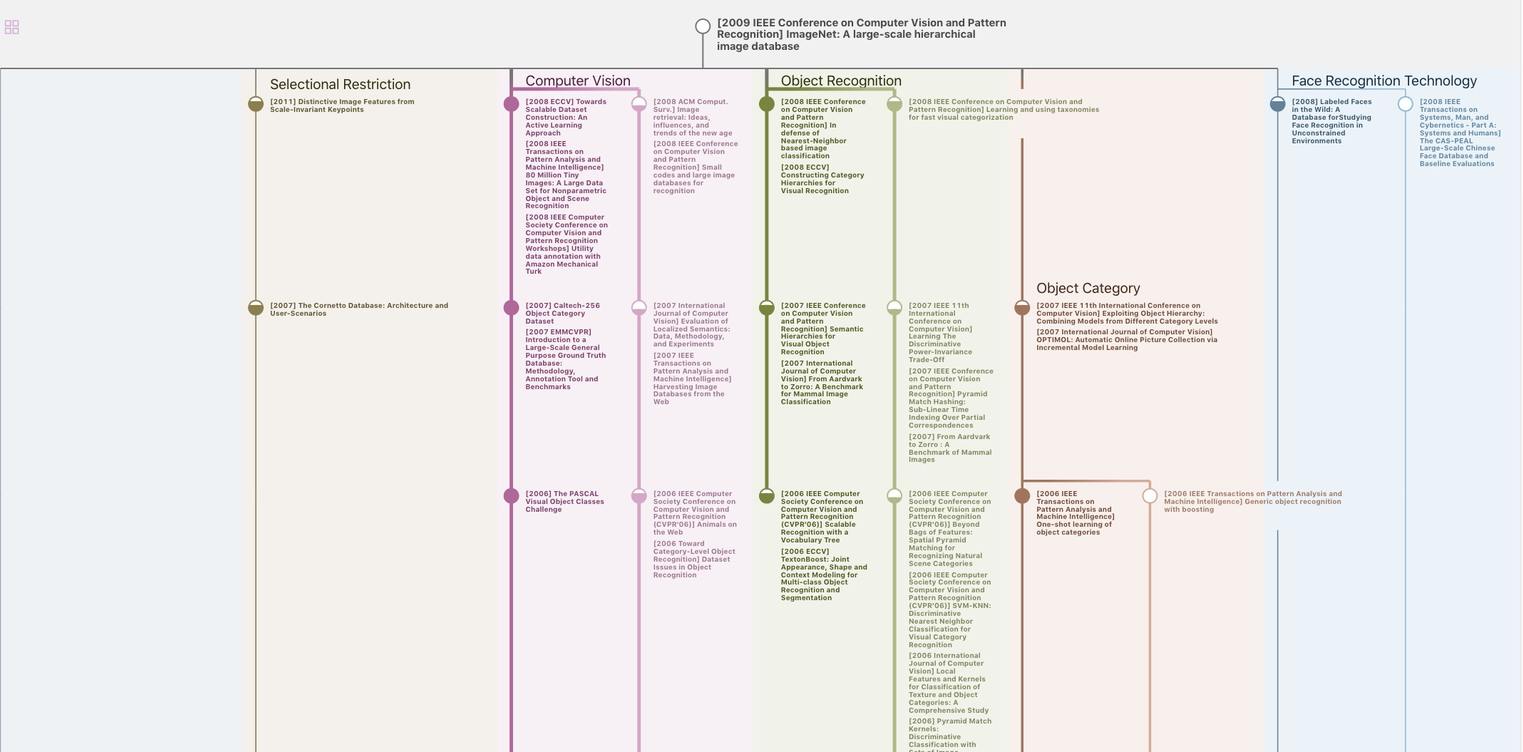
生成溯源树,研究论文发展脉络
Chat Paper
正在生成论文摘要