Joint Learning and Optimization for Multi-product Pricing under a General Cascade Click Model
Social Science Research Network(2018)
摘要
We consider a pricing problem for a set of products displayed on a list. We assume a general cascade click model, in which customers examine the products in a decreasing order of display, from the top to (potentially) the bottom of the list. At each step, customers can decide to either purchase the current product, forego the current product and continue examining the next product, or simply terminate the search without purchasing any product. The objective of the firm is to optimally price the products to maximize its expected total revenues. We first study the case where the firm knows all problem parameters and derive a relatively explicit expression for the optimal prices of the products, for some cases. This is useful for uncovering some interesting managerial insights regarding the properties of the optimal prices when customers behave in the manner prescribed by the general cascade click model. We then study the case where the parameters are unknown and need to be learned/estimated from the data. For this case, we develop an online algorithm that jointly learns the unknown parameters and optimizes the prices of the products. Moving beyond the base model where the only decision of the firm is the prices, we also consider several extensions to more complex settings that include filtering options (i.e., we allow customers to filter out some of the products using some filtering options) and display ranking decisions. We discuss how our algorithm in the base setting can be extended to these more general settings. Our numerical results highlight the value of properly taking into account customer search behavior when designing a learning algorithm.
更多查看译文
AI 理解论文
溯源树
样例
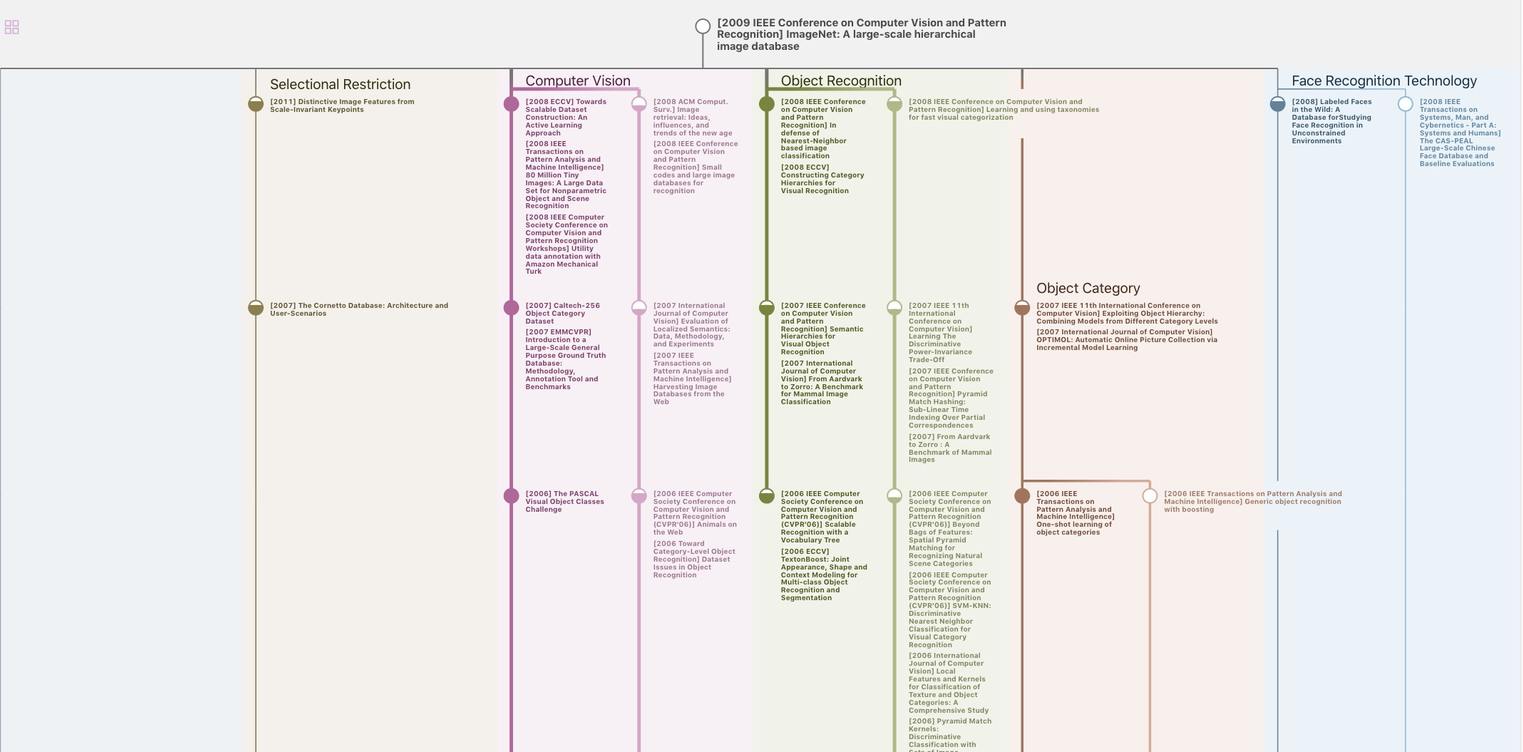
生成溯源树,研究论文发展脉络
Chat Paper
正在生成论文摘要