Feature Extraction Approach Selection of Non-GO Termed Proteins for the Backup Method of Protein Subcellular Localization Prediction
2018 International Conference on Computer, Communication, Chemical, Material and Electronic Engineering (IC4ME2)(2018)
摘要
In protein subcellular localization prediction, several types of feature extraction methods have been proposed to produce different levels of accuracy. Among the feature extraction methods, feature extraction based on GO terms provides better accuracy. However, there are several cases, especially for newly discovered proteins, where the GO term feature representations are not available. Here, this types of proteins are called as ‘non-GO termed’ proteins. In such cases, researcher depends on some backup methods using other features extraction approaches but in most of the cases, prediction performance of only the backup method is not provided separately, that is, combined prediction performance is given based on GO term based method along with the backup method. This makes it harder to get any idea about the prediction performance of the non-GO termed proteins. In this paper, we have considered five sequence driven feature extraction approaches and investigated how feature extraction approaches affect the performance for non-GO termed proteins. Finally, we have developed three prediction systems using three different methods to get classifier independent result. The experimental result shows that, Dipeptide Composition provides better actual accuracy for the gram-positive bacteria dataset, while Amino Acid Composition provides higher actual accuracy for the gram-negative bacteria dataset.
更多查看译文
关键词
Subcellular Localization Prediction,non-GO Termed Proteins,Feature Extraction Approach Selection,Imbalance Data Management
AI 理解论文
溯源树
样例
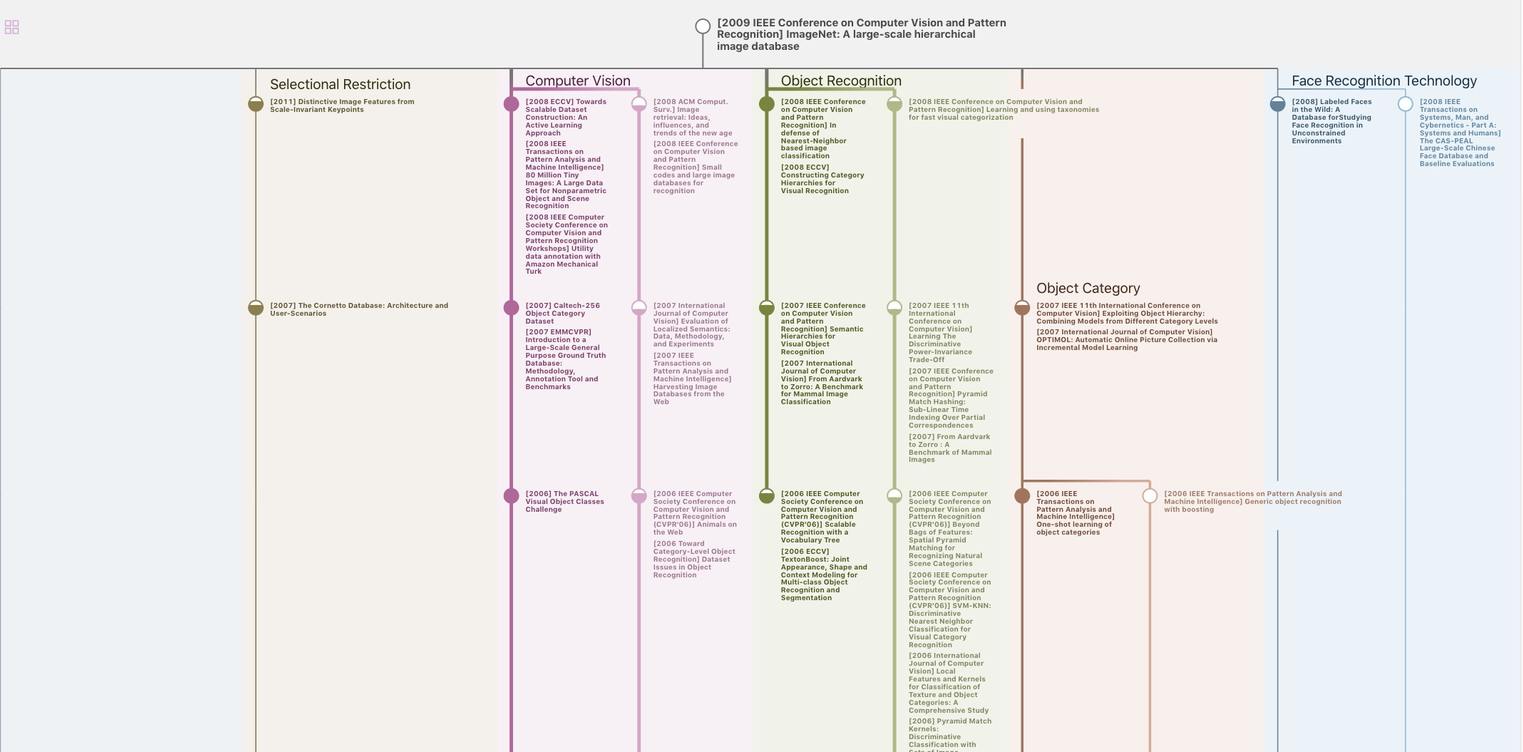
生成溯源树,研究论文发展脉络
Chat Paper
正在生成论文摘要