Trajectory Learning for Stable Bipedal Walking Robots using Sequential Networks
2020 IEEE 7th Uttar Pradesh Section International Conference on Electrical, Electronics and Computer Engineering (UPCON)(2020)
摘要
Bipedal walking in an unknown environment is an extremely challenging problem due to its inherently unstable structure. For bipedal robots, so far, this problem has been tried using analytical methods with predefined sets of parameters, having limited success. Such robots could walk only in a structured environment with a flat floor and other restrictions. This paper proposes such models having learning ability to be imparted to the biped robots based on walking sequences. Two sequential network models have been configured, Gated Recurrent Unit (GRU) and Long Short Term Memory (LSTM), to learn the joint trajectories of the hip, knee, and ankle joints, separately and their performances have been studied. Using these two models, learning has been imparted to a robot both in the sagittal plane and in the frontal plane. We use the Pybullet physics engine of a biped robot for simulation purposes. Data have been collected by running several simulations and trained our sequential network models with these collected data. We observe that the present model's walking patterns could be learned both by GRU and LSTM within ten episodes with L2 regularizer. For our present study, the GRU model has several thousand fewer network parameters to learn than the LSTM model. We recommend using GRU as a first choice for controlling the biped robots, walking with simple modes. However, for more complex modes, such as walking with long steps, brisk walking, walking, having push recovery capability, etc., we may need to collect larger data sets for training and testing to suggest which model would be more suitable. Our recommendations are backed by rigorous experimentation.
更多查看译文
关键词
Biped robot model,Pybullet,Gated Recurrent Unit (GRU),Long short term memory (LSTM)
AI 理解论文
溯源树
样例
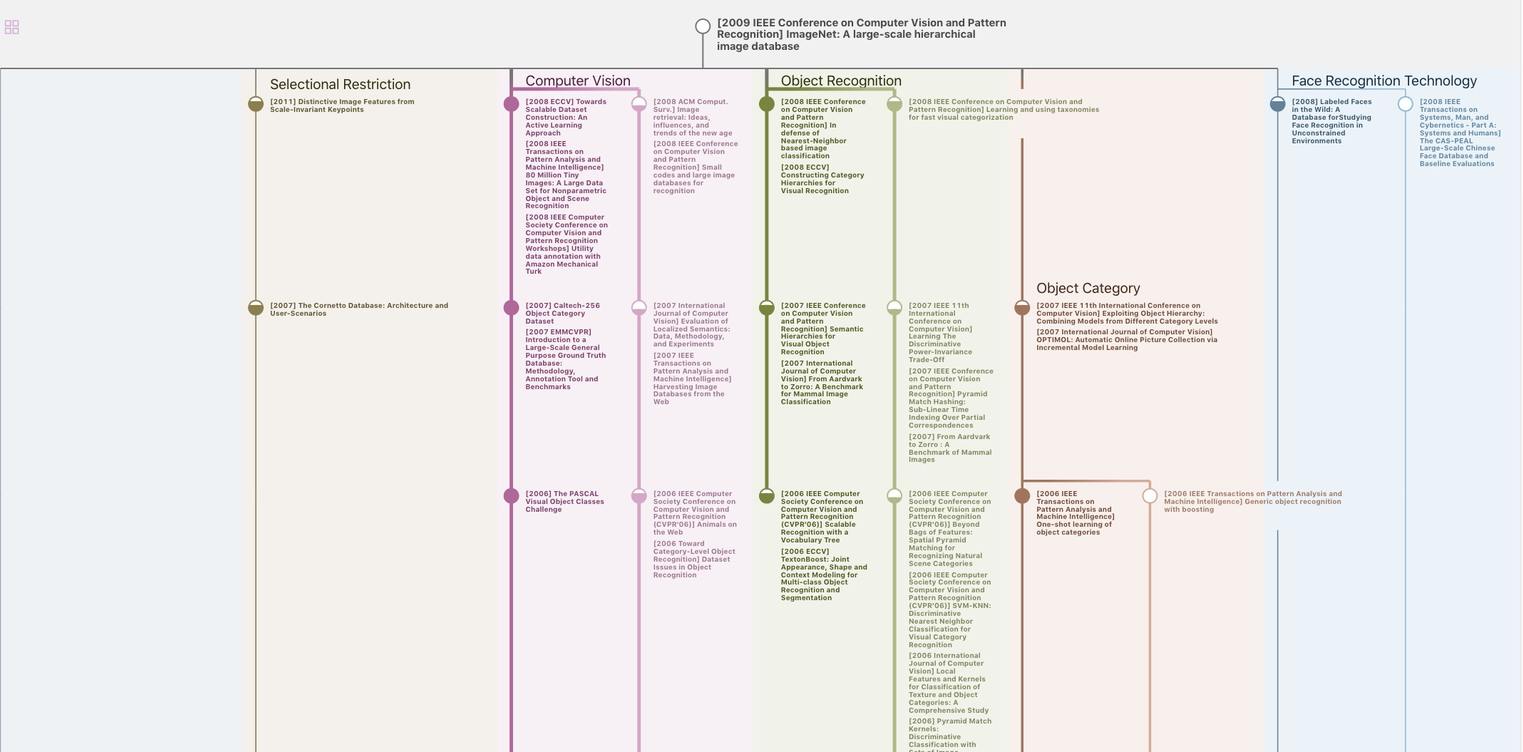
生成溯源树,研究论文发展脉络
Chat Paper
正在生成论文摘要