Applications Of Feature Weighted Fuzzy C-Means Clustering And Genetic Algorithm Optimization For Load Identification In NILM Systems
2020 International Conference on Wavelet Analysis and Pattern Recognition (ICWAPR)(2020)
Abstract
An improved fuzzy clustering non-invasive load monitoring method based on genetic algorithm for feature weight optimization is proposed. The non-intrusive load monitoring research needs to extract the features of electrical appliance waveform data, which has the problems of large number of features and redundant features. In order to achieve a good recognition effect, when using Fuzzy C-Means to recognize, the traditional method often needs to filter the features, but this process is complicated and does not fully consider the different influences of different features on the model performance. In this paper, the Fuzzy C-Means algorithm is improved, considering that different features have different influences on the clustering recognition effect, and each feature is given importance weight coefficient. The genetic algorithm is then used to optimize the feature weights in order to find the best model performance Combination of feature weight coefficient. Experimental results show that this processing method can effectively improve the performance of the classifier, and at the same time does not require manual tedious feature selection process.
MoreTranslated text
Key words
Non-intrusive load monitoring,Improved fuzzy clustering,Genetic algorithm,Feature weight coefficient
AI Read Science
Must-Reading Tree
Example
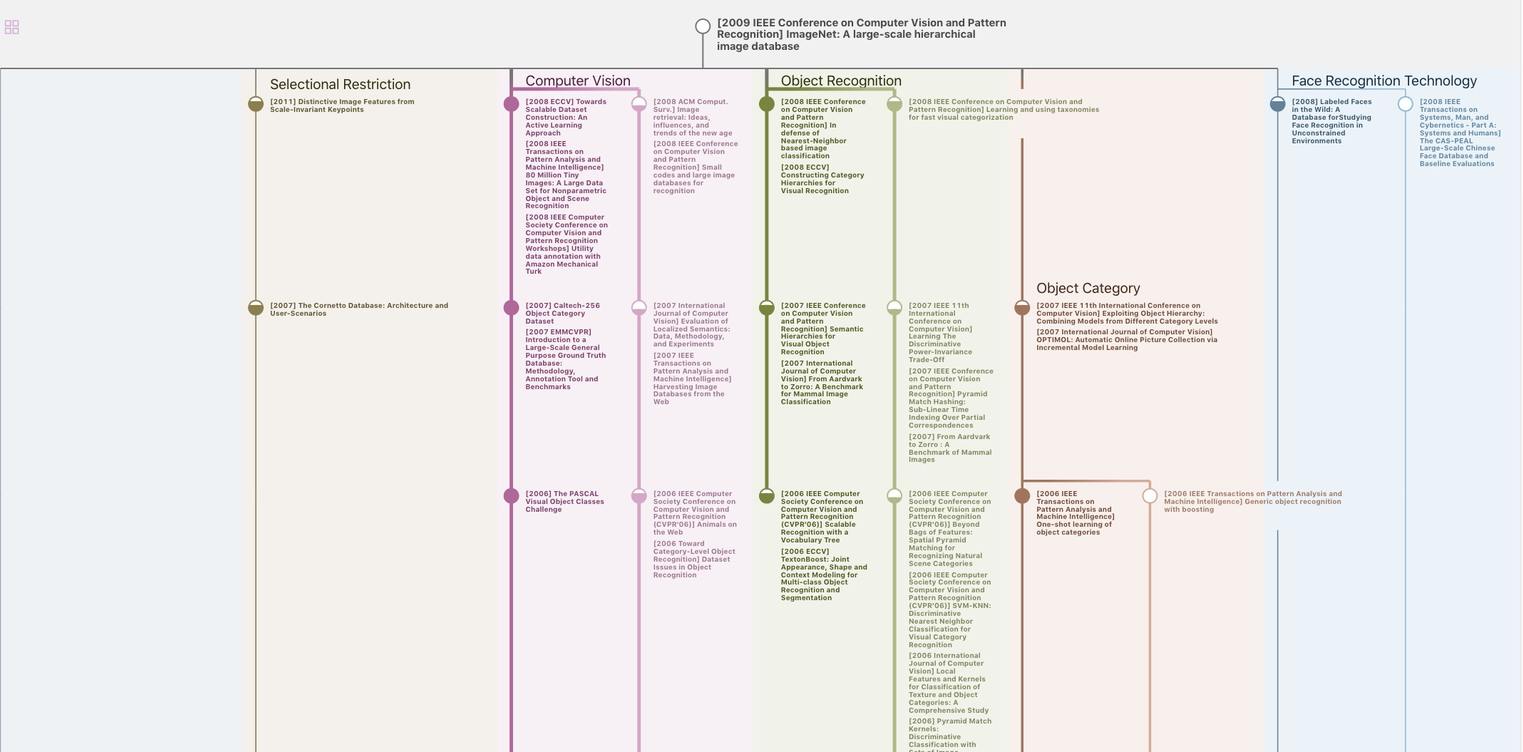
Generate MRT to find the research sequence of this paper
Chat Paper
Summary is being generated by the instructions you defined