Towards large-scale and spatiotemporally resolved diagnosis of electronic density of states by deep learning
PHYSICAL REVIEW B(2022)
摘要
Modern laboratory techniques such as ultrafast laser excitation and shock compression can bring matter into highly nonequilibrium states with complex structural transformation, metallization, and dissociation dynamics. To understand and model the dramatic change of both electronic structures and ion dynamics during such dynamic processes, the traditional method faces difficulties. Here, we demonstrate the ability of a deep neural network (DNN) to capture the atomic local-environment dependence of the electronic density of states (DOS) for both multicomponent systems under exoplanet thermodynamic conditions and nonequilibrium systems during superheated melting processes. Large-scale and time-resolved diagnosis of DOS can be efficiently achieved within the accuracy of the ab initio method. Moreover, the atomic contribution to DOS given by the DNN model accurately reveals the information of the local neighborhood for the selected atom, which thus can serve as robust order parameters to identify different phases and intermediate local structures, strongly highlighting the efficacy of this DNN model in studying dynamic processes.
更多查看译文
关键词
electronic density,deep learning,large-scale
AI 理解论文
溯源树
样例
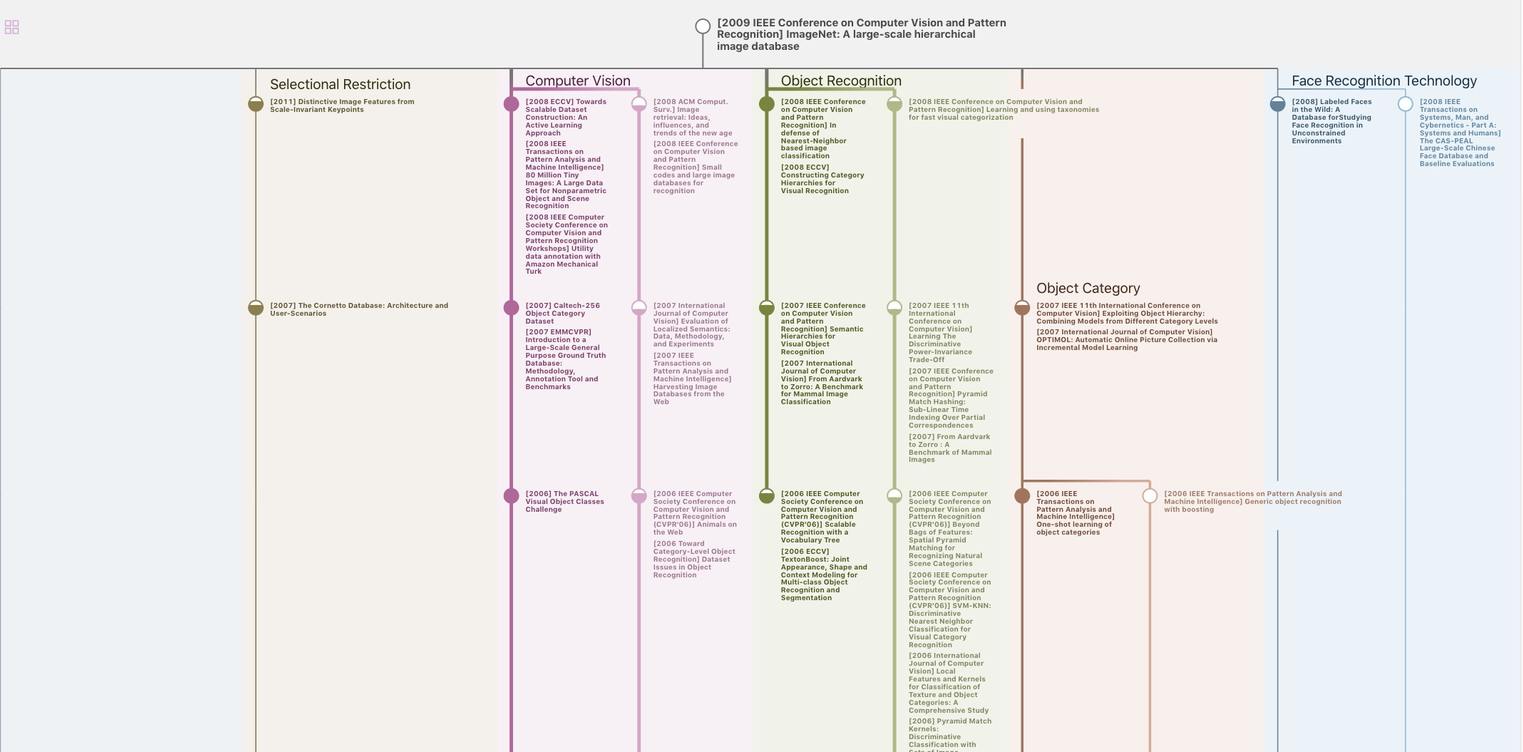
生成溯源树,研究论文发展脉络
Chat Paper
正在生成论文摘要