Monocular Depth Distribution Alignment with Low Computation
IEEE International Conference on Robotics and Automation(2022)
摘要
The performance of monocular depth estimation generally depends on the amount of parameters and computational cost. It leads to a large accuracy contrast between light-weight networks and heavy-weight networks, which limits their application in the real world. In this paper, we model the majority of accuracy contrast between them as the difference of depth distribution, which we call 'Distribution drift'. To this end, a distribution alignment network (DANet) is proposed. We firstly design a pyramid scene transformer (PST) module to capture inter-region interaction in multiple scales. By perceiving the difference of depth features between every two regions, DANet tends to predict a reasonable scene structure, which fits the shape of distribution to ground truth. Then, we propose a local-global optimization (LGO) scheme to realize the supervision of global range of scene depth. Thanks to the alignment of depth distribution shape and scene depth range, DANet sharply alleviates the distribution drift, and achieves a comparable performance with prior heavy-weight methods, but uses only 1% floating-point operations per second (FLOPs) of them. The experiments on two datasets, namely the widely used NYUDv2 dataset and the more challenging iBims-1 dataset, demonstrate the effectiveness of our method. The source code is available at https://github.com/YiLiM1/DANet.
更多查看译文
关键词
monocular depth distribution alignment
AI 理解论文
溯源树
样例
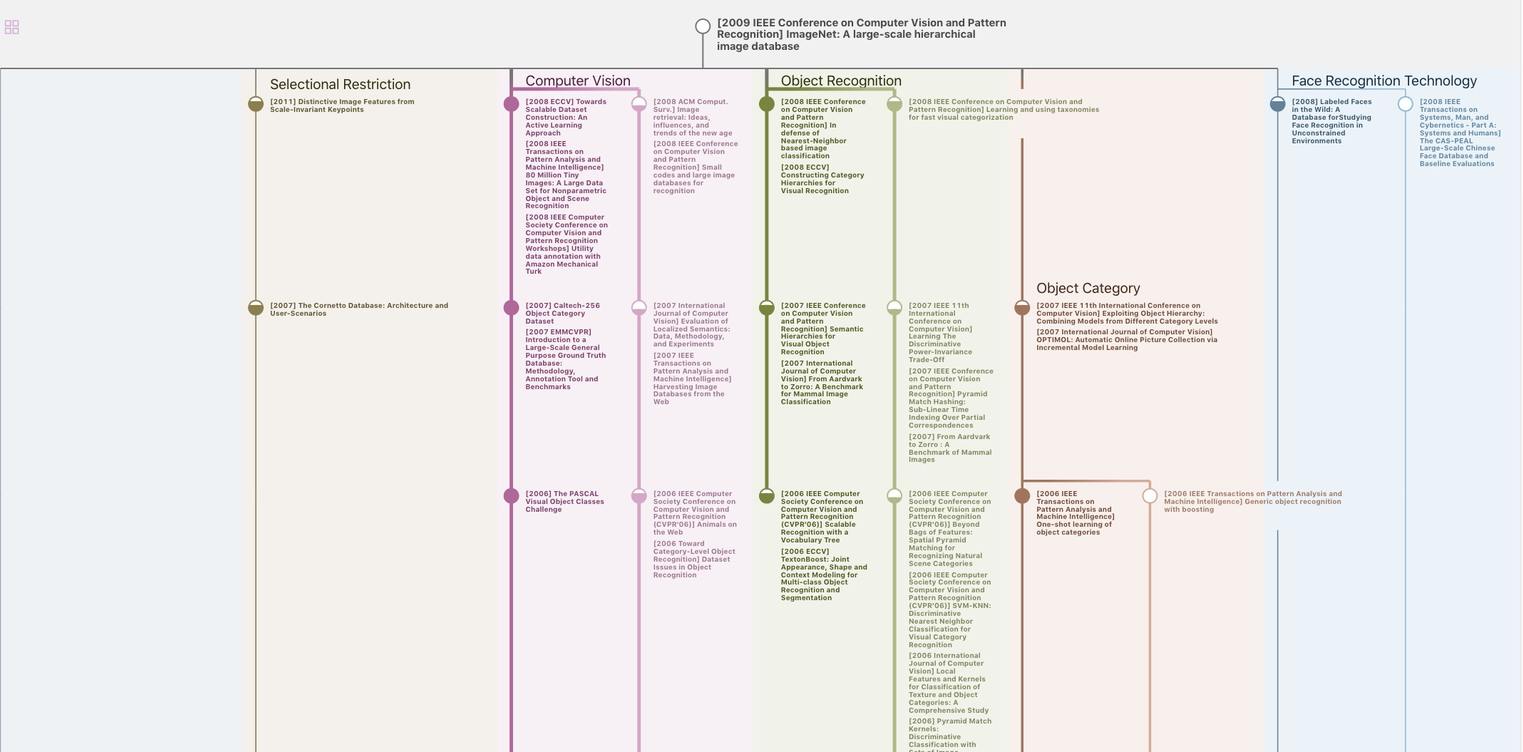
生成溯源树,研究论文发展脉络
Chat Paper
正在生成论文摘要