Score-Based Generative Models for Molecule Generation
arxiv(2022)
摘要
Recent advances in generative models have made exploring design spaces easier for de novo molecule generation. However, popular generative models like GANs and normalizing flows face challenges such as training instabilities due to adversarial training and architectural constraints, respectively. Score-based generative models sidestep these challenges by modelling the gradient of the log probability density using a score function approximation, as opposed to modelling the density function directly, and sampling from it using annealed Langevin Dynamics. We believe that score-based generative models could open up new opportunities in molecule generation due to their architectural flexibility, such as replacing the score function with an SE(3) equivariant model. In this work, we lay the foundations by testing the efficacy of score-based models for molecule generation. We train a Transformer-based score function on Self-Referencing Embedded Strings (SELFIES) representations of 1.5 million samples from the ZINC dataset and use the Moses benchmarking framework to evaluate the generated samples on a suite of metrics.
更多查看译文
关键词
generative models,molecule,generation,score-based
AI 理解论文
溯源树
样例
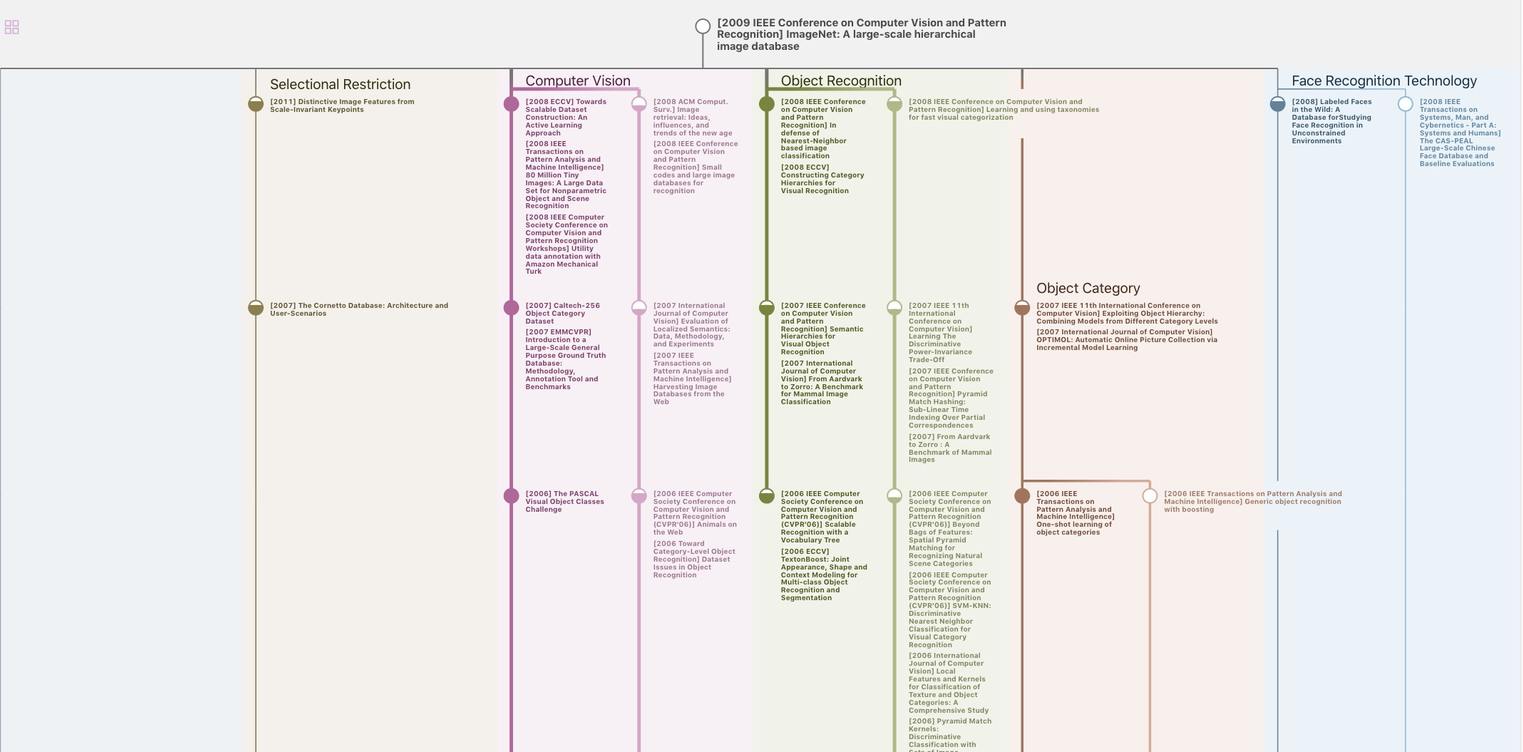
生成溯源树,研究论文发展脉络
Chat Paper
正在生成论文摘要