Joint Learning of Salient Object Detection, Depth Estimation and Contour Extraction.
IEEE Trans. Image Process.(2022)
摘要
Benefiting from color independence, illumination invariance and location discrimination attributed by the depth map, it can provide important supplemental information for extracting salient objects in complex environments. However, high-quality depth sensors are expensive and can not be widely applied. While general depth sensors produce the noisy and sparse depth information, which brings the depth-based networks with irreversible interference. In this paper, we propose a novel multi-task and multi-modal filtered transformer (MMFT) network for RGB-D salient object detection (SOD). Specifically, we unify three complementary tasks: depth estimation, salient object detection and contour estimation. The multi-task mechanism promotes the model to learn the task-aware features from the auxiliary tasks. In this way, the depth information can be completed and purified. Moreover, we introduce a multi-modal filtered transformer (MFT) module, which equips with three modality-specific filters to generate the transformer-enhanced feature for each modality. The proposed model works in a depth-free style during the testing phase. Experiments show that it not only significantly surpasses the depth-based RGB-D SOD methods on multiple datasets, but also precisely predicts a high-quality depth map and salient contour at the same time. And, the resulted depth map can help existing RGB-D SOD methods obtain significant performance gain.
更多查看译文
关键词
Salient object detection,multi-task learning,multi-modal filtered transformer,modality-specific filters
AI 理解论文
溯源树
样例
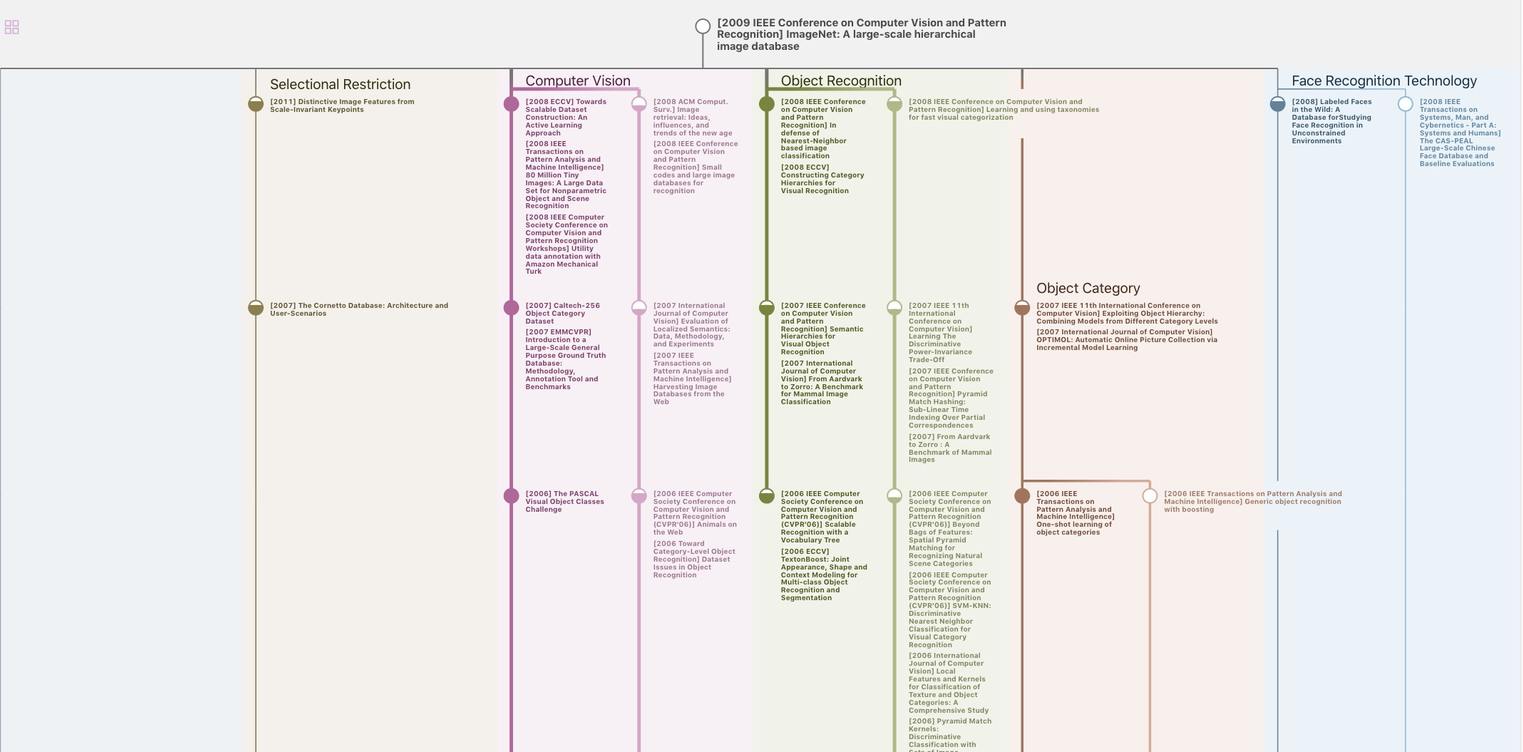
生成溯源树,研究论文发展脉络
Chat Paper
正在生成论文摘要