On-Robot Policy Learning with $\mathrm{O}(2)$-Equivariant SAC
arxiv(2022)
摘要
Recently, equivariant neural network models have been shown to be useful in improving sample efficiency for tasks in computer vision and reinforcement learning. This paper explores this idea in the context of on-robot policy learning where a policy must be learned entirely on a physical robotic system without reference to a model, a simulator, or an offline dataset. We focus on applications of $\mathrm{SO}(2)$-Equivariant SAC to robotic manipulation and explore a number of variations of the algorithm. Ultimately, we demonstrate the ability to learn several non-trivial manipulation tasks completely through on-robot experiences in less than an hour or two of wall clock time.
更多查看译文
AI 理解论文
溯源树
样例
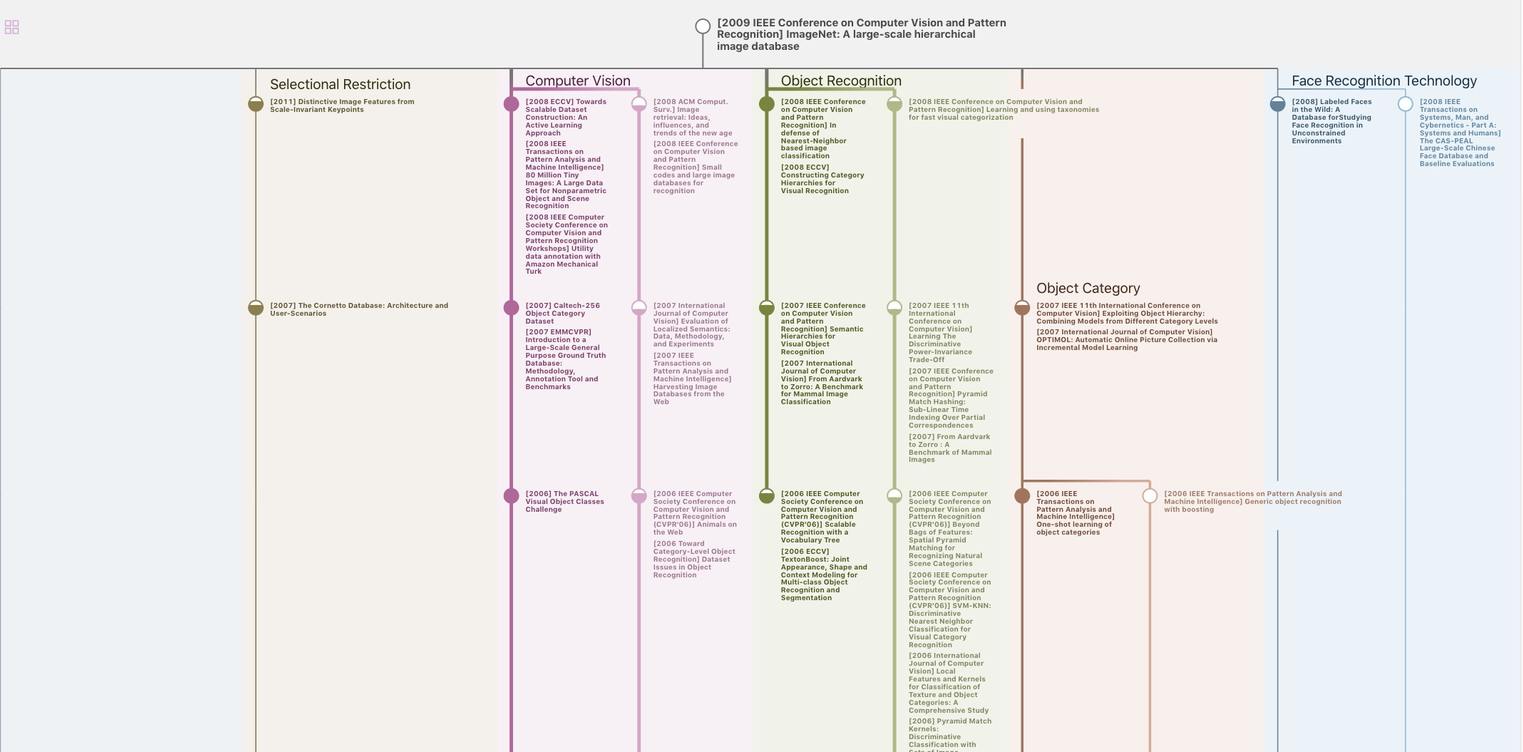
生成溯源树,研究论文发展脉络
Chat Paper
正在生成论文摘要