A continuous multiple hypothesis testing framework for optimal exoplanet detection
ANNALS OF APPLIED STATISTICS(2024)
摘要
When searching for exoplanets, one wants to count how many planets orbit a given star, and to determine what their characteristics are. If the estimated planet characteristics are too far from those of a planet truly present, this should be considered as a false detection. This setting is a particular instance of a general one: aiming to retrieve parametric components in a dataset corrupted by nuisance signals, with a certain accuracy on their parameters. We exhibit a detection criterion minimizing false and missed detections, either as a function of their relative cost or when the expected number of false detections is bounded. If the components can be separated in a technical sense discussed in detail, the optimal detection criterion is a posterior probability obtained as a by-product of Bayesian evidence calculations. Optimality is guaranteed within a model, and we introduce model criticism methods to ensure that the criterion is robust to model errors. We show on two simulations emulating exoplanet searches that the optimal criterion can significantly outperform other criteria. Finally, we show that our framework offers solutions for the identification of components of mixture models and Bayesian false discovery rate control when hypotheses are not discrete.
更多查看译文
关键词
Exoplanets,Bayesian,false discovery rate,decision theory,maximum utility
AI 理解论文
溯源树
样例
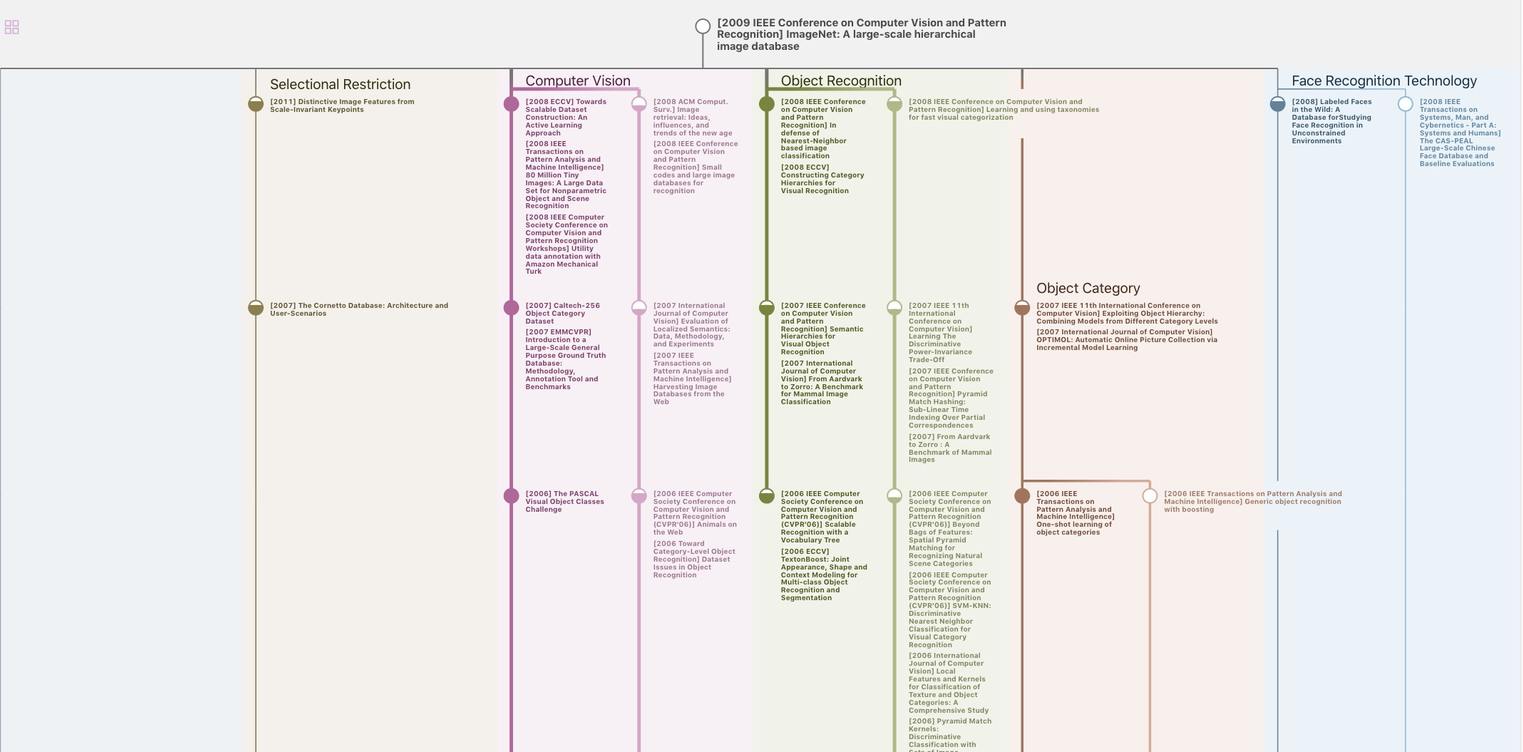
生成溯源树,研究论文发展脉络
Chat Paper
正在生成论文摘要