Towards Protecting Sensitive Text with Differential Privacy
2021 IEEE 20th International Conference on Trust, Security and Privacy in Computing and Communications (TrustCom)(2021)
摘要
Natural language processing can often require handling privacy-sensitive text. To avoid revealing confidential information, data owners and practitioners can use differential privacy, which provides a mathematically guaranteeable definition of privacy preservation. In this work, we explore the possibility of applying differential privacy to feature hashing. Feature hashing is a common technique for handling out-of-dictionary vocabulary, and for creating a lookup table to find feature weights in constant time. Traditionally, differential privacy involves adding noise to hide the true value of data points. We show that due to the finite nature of the output space when using feature hashing, a noiseless approach is also theoretically sound. This approach opens up the possibility of applying strong differential privacy protections to NLP models trained with feature hashing. Preliminary experiments show that even common words can be protected with (0.04, 10(-5))-differential privacy, with only a minor reduction in model utility.
更多查看译文
关键词
Differential privacy,Privacy,Vocabulary,Computational modeling,Conferences,Natural language processing,Security
AI 理解论文
溯源树
样例
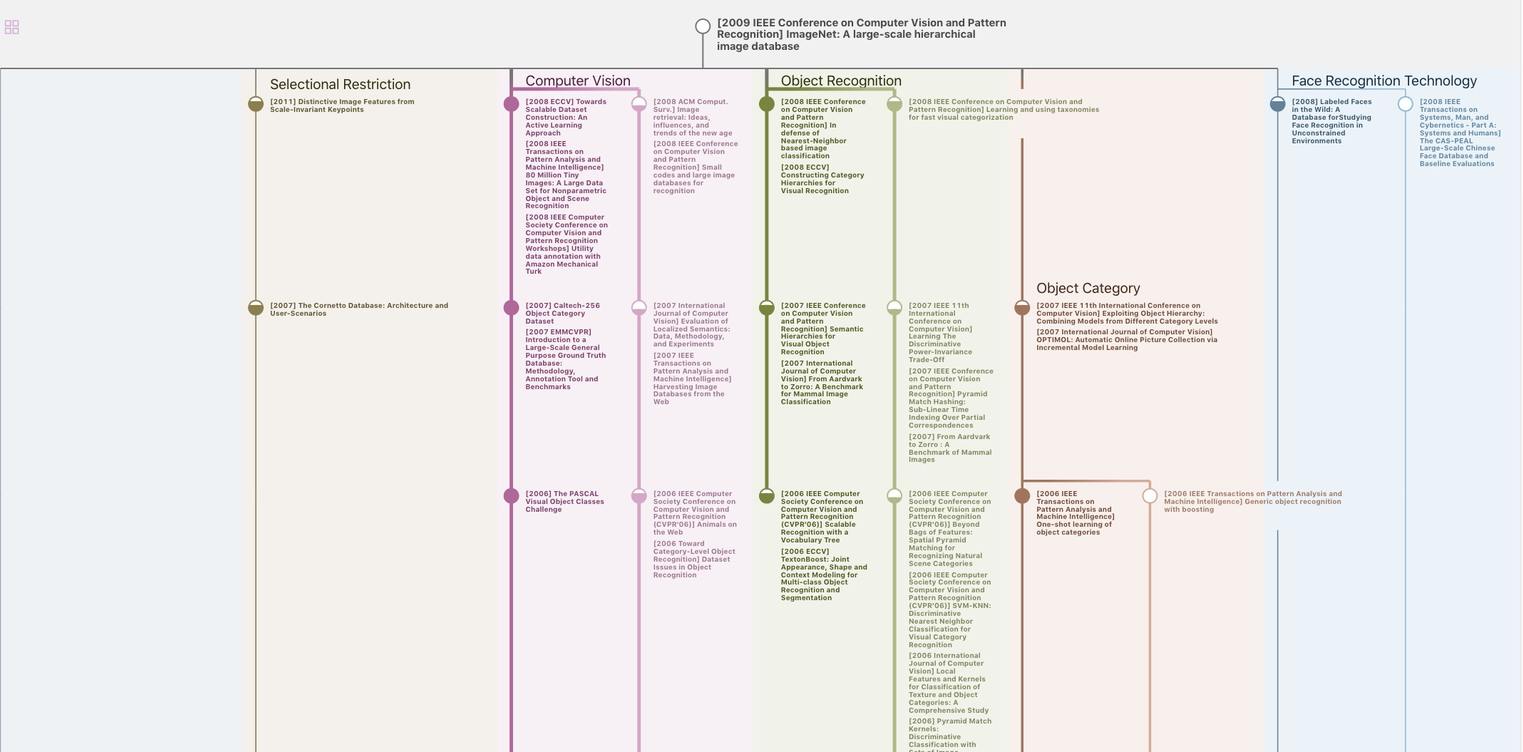
生成溯源树,研究论文发展脉络
Chat Paper
正在生成论文摘要