Localization of Hotspots from Lock-in Thermography Images for Failure Analysis
2021 IEEE 23rd Electronics Packaging Technology Conference (EPTC)(2021)
摘要
Lock-in Thermography (LIT) is a commonly used non-destructive technique in the failure analysis (FA) of integrated circuits. The presence of defects changes the heat flow, resulting in the formation of thermals hotspots that are captured by the LIT imaging system. Currently, the identification of the hotspots requires knowledge of an experienced FA specialist, making the identification time consuming and prone to human error. In this paper, an algorithm has been developed to automate the process of hotspot localization by training on existing annotated images. In the annotated images, thermal signal, represented by colored pixels obtained through the mapping of scalar values onto a jet color mapping, were overlaid with gray X-ray or topological background. The algorithm was able to identify the thermal signal as colored pixels from the gray background. It was also able to identify diffused or fragmented hotspot signal as a single hotspot through Density-Based Spatial Clustering of Applications with Noise (DBSCAN) and separate the true hotspot from spurious noise through noise removal and ranking of candidate hotspots by size. The algorithm was applied on 103 images. For 86 images, the hotspots were correctly identified as the only hotspots in the images. The correct hotspots were identified along with other incorrect hotspots in 11 images. The incorrectly identified hotspots were not removed during the noise removal and hotspots ranking steps as they were larger than the size threshold and comparable in size to the actual hotspots. For the last 6 images, the actual hotspots were not identified, and incorrect hotspots were reported instead. The incorrectly identified hotspots were often larger than the actual hotspots and usually found outside of the package region. In the future, identification of hotspots localization could be improved by determining region of interest within the images.
更多查看译文
AI 理解论文
溯源树
样例
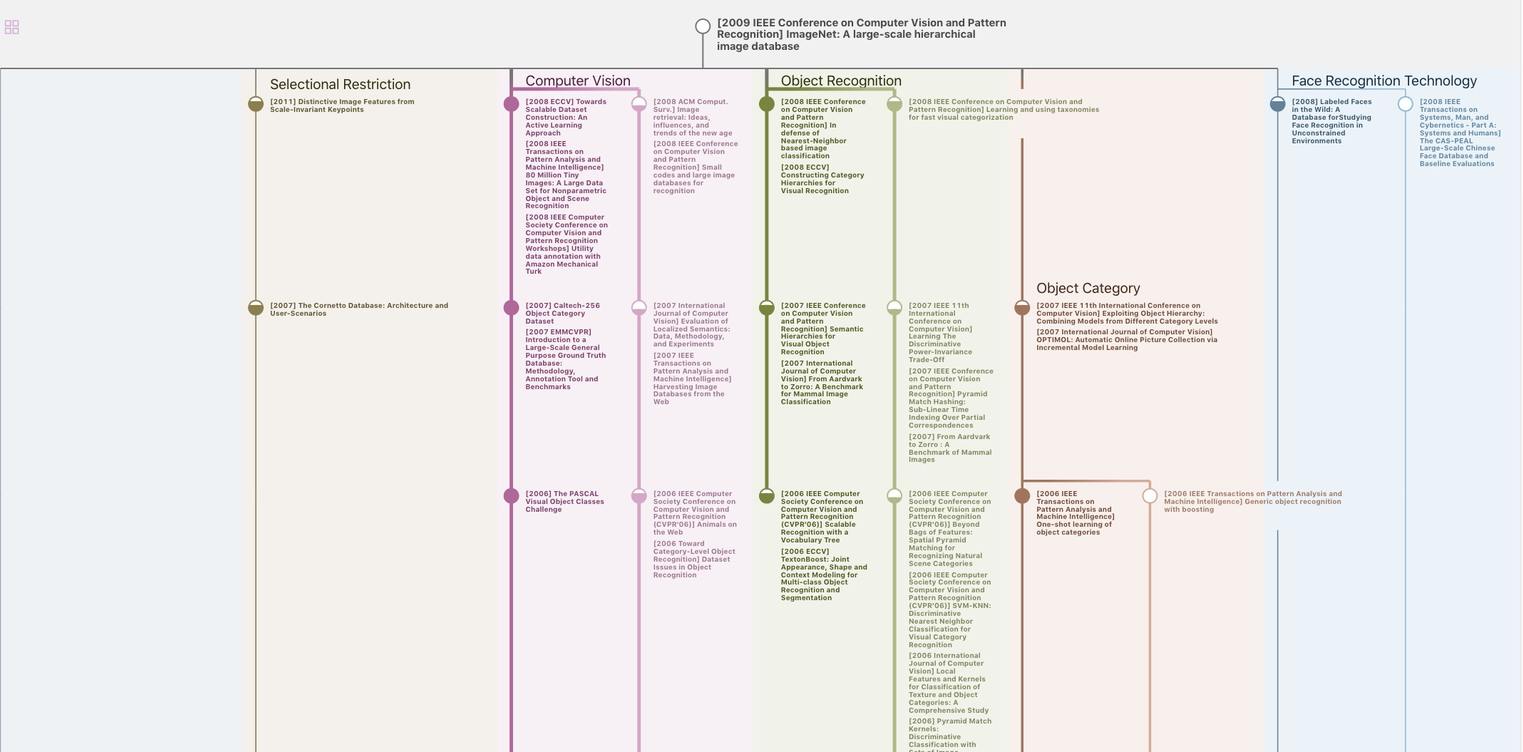
生成溯源树,研究论文发展脉络
Chat Paper
正在生成论文摘要