Augmenting BERT with CNN for Multiple Choice Question Answering
2021 24th International Conference on Computer and Information Technology (ICCIT)(2021)
摘要
Multiple Choice Question (MCQ) answering is a strenuous task intended to determine the right answer from a set of given options. It demands a deep semantic understanding of the question, answer, and knowledge. In this article, we present a Convolutional Neural Networks (CNN) based model extended with Bidirectional Encoder Representations from Transformers (BERT) to answer complex multiple-choice questions. Given an article and a MCQ, the model selects the correct option by ranking each question-option tuple. The proposed CNN based model uses question-option tuple as input to perform better than Long Short-Term Memory(LSTM) based baselines by 22.7 for the Textbook Question Answering (TQA) [1] and SciQ [2] datasets.
更多查看译文
关键词
Question Answering,BERT,CNN,TQA,SciQ
AI 理解论文
溯源树
样例
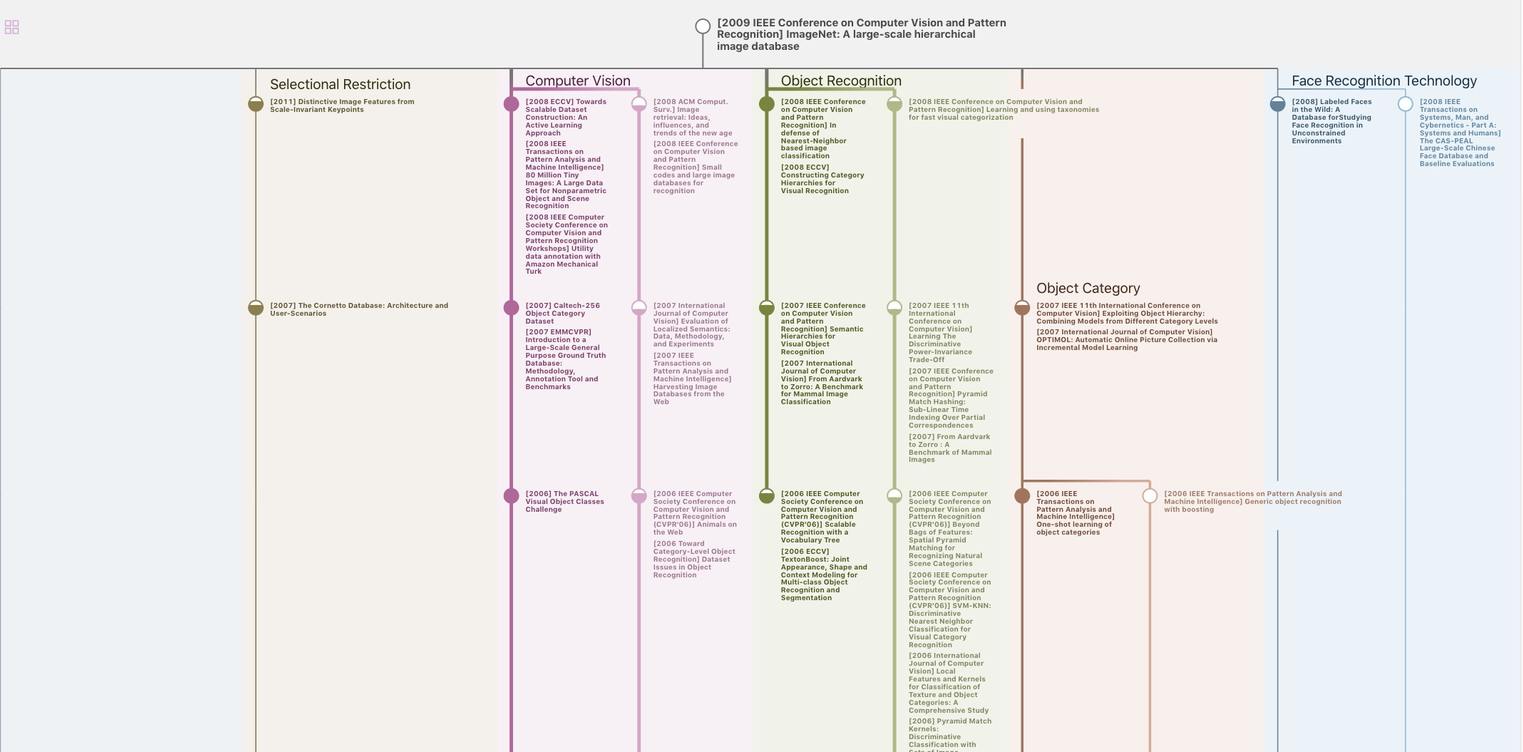
生成溯源树,研究论文发展脉络
Chat Paper
正在生成论文摘要