Efficiency Enhancement of Evolutionary Neural Architecture Search via Training-Free Initialization
2021 8th NAFOSTED Conference on Information and Computer Science (NICS)(2021)
摘要
In this paper, we adapt a method to enhance the efficiency of multi-objective evolutionary algorithms (MOEAs) when solving neural architecture search (NAS) problems by improving the initialization stage with minimal costs. Instead of sampling a small number of architectures from the search space, we sample a large number of architectures and estimate the performance of each one without invoking the computationally expensive training process but by using a zero-cost proxy. After ranking the architectures via their zero-cost proxy values and efficiency metrics, the best architectures are then chosen as the individuals of the initial population. To demonstrate the effectiveness of our method, we conduct experiments on the widely-used NAS-Bench-101 and NAS-Bench-201 benchmarks. Experimental results exhibit that the proposed method achieves not only considerable enhancements on the quality of initial populations but also on the overall performance of MOEAs in solving NAS problems. The source code of the paper is available at https://github.com/ELO-Lab/ENAS-TFI.
更多查看译文
关键词
Evolutionary computation,Neural architecture search,Multi-objective optimization
AI 理解论文
溯源树
样例
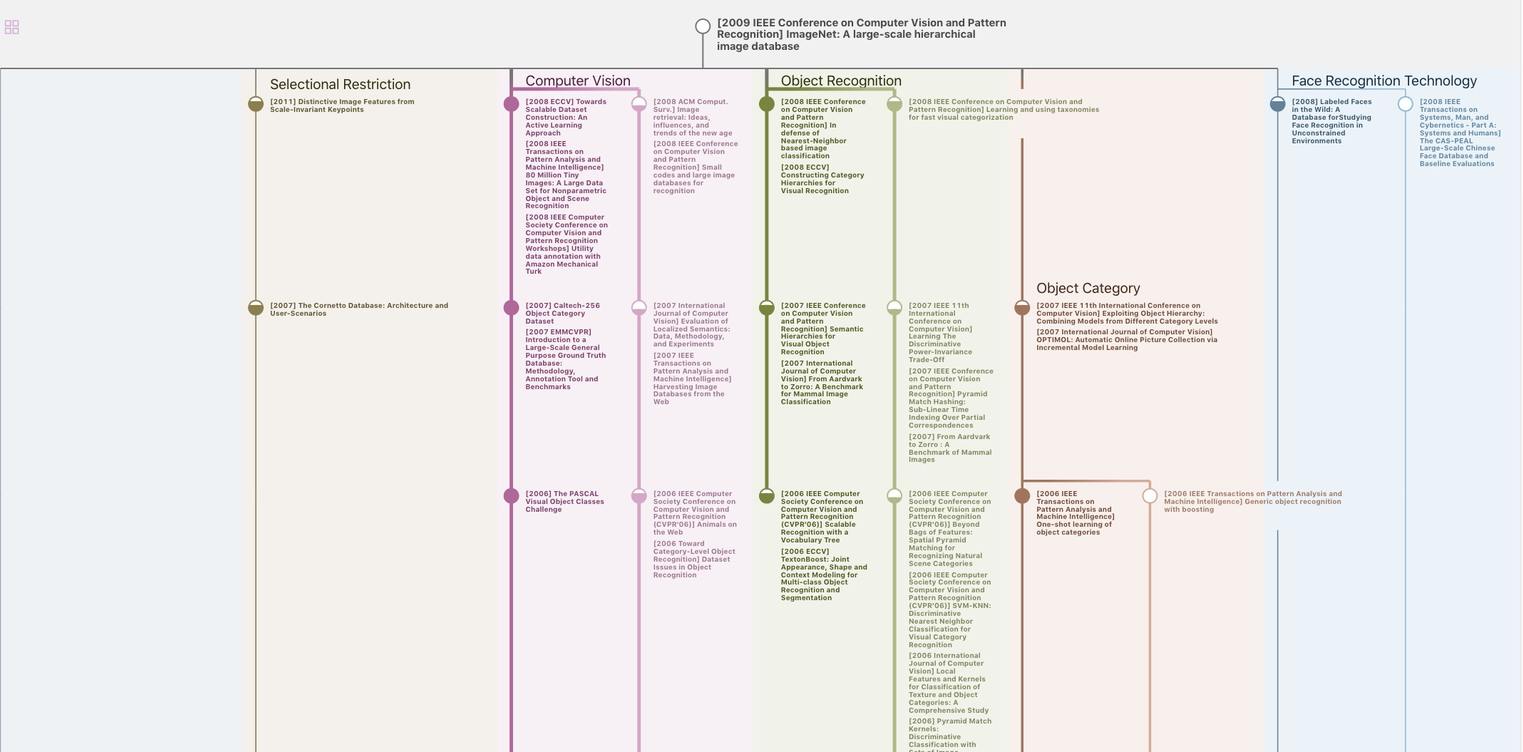
生成溯源树,研究论文发展脉络
Chat Paper
正在生成论文摘要