Recurrent Neural Network-Augmented Locally Adaptive Interpretable Regression for Multivariate Time-Series Forecasting
IEEE ACCESS(2022)
Abstract
Explaining dynamic relationships between input and output variables is one of the most important issues in time dependent domains such as economic, finance and so on. In this work, we propose a novel locally adaptive interpretable deep learning architecture that is augmented by recurrent neural networks to provide model explainability and high predictive accuracy for time-series data. The proposed model relies on two key aspects. First, the base model should be a simple interpretable model. In this step, we obtain our base model using a simple linear regression and statistical test. Second, we use recurrent neural networks to re-parameterize our base model to make the regression coefficients adaptable for each time step. Our experimental results on public benchmark datasets showed that our model not only achieves better predictive performance than the state-of-the-art baselines, but also discovers the dynamic relationship between input and output variables.
MoreTranslated text
Key words
Predictive models, Linear regression, Adaptation models, Forecasting, Data models, Input variables, Mathematical models, Explainable AI, linear regression, recurrent neural network, time-series forecasting
AI Read Science
Must-Reading Tree
Example
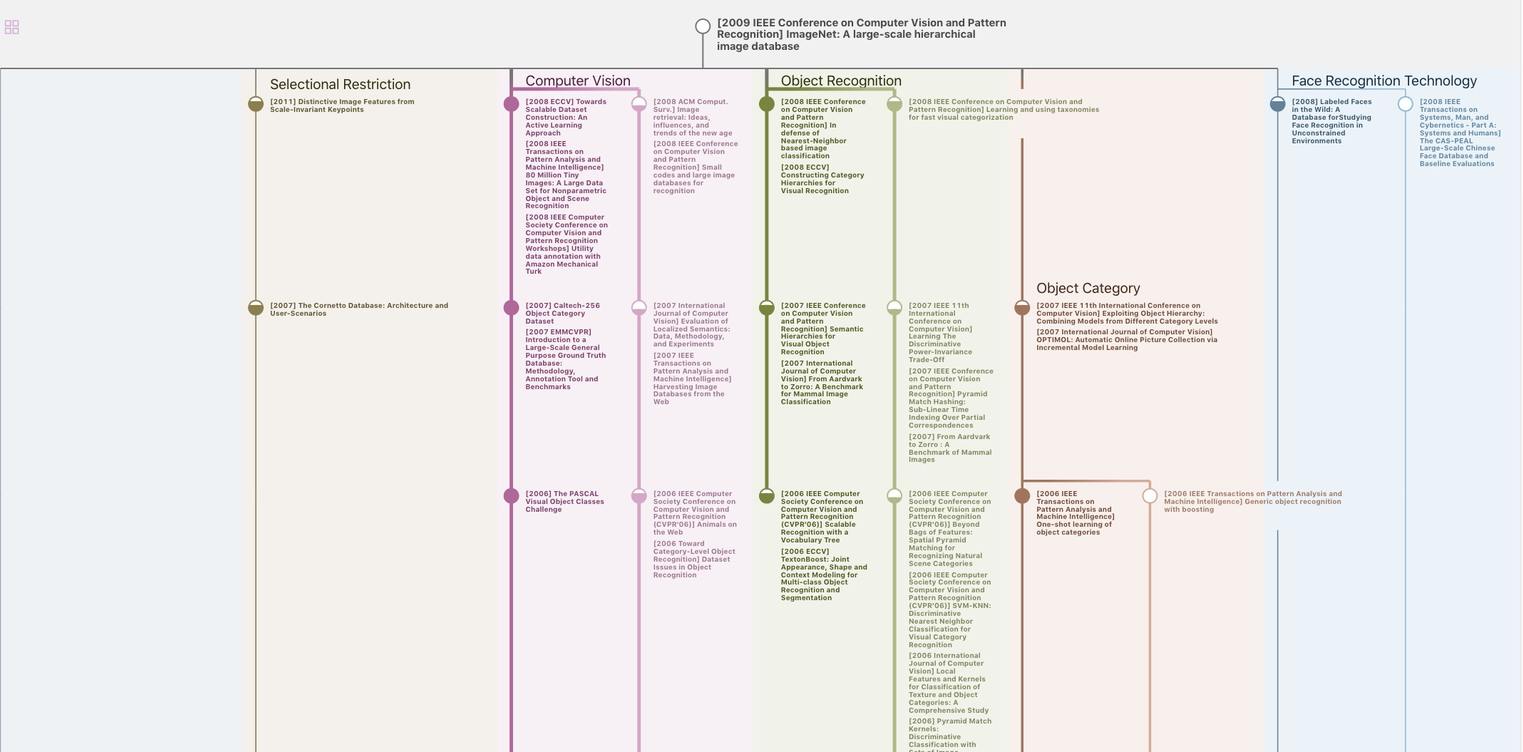
Generate MRT to find the research sequence of this paper
Chat Paper
Summary is being generated by the instructions you defined