A Novel Vehicle Destination Prediction Model with Expandable Features Using Attention Mechanism and Variational Autoencoder
IEEE transactions on intelligent transportation systems(2022)
摘要
The daily passage of vehicles generates a huge amount of location-aware social data, which provides a rich source of data for analyzing vehicle travel behavior. Being able to accurately predict the future destinations of vehicle travel has great economic value and social impact. The presence of larger sparsity, fewer features and error information in the real dataset led to difficulties in convergence of previous models. Therefore, we propose a Novel Vehicle Destination Prediction Model with Expandable Features Using Attention Mechanism and Variational Autoencoder (EFAMVA). The EFAMVA model combines the autoencoder model and the attention mechanism has overcome the above mentioned problems. The variational autoencoder model obtains the hidden features conforming to the characteristics of the data from the structured vehicle driving data. And the attention mechanism can learn the appropriate combination of weight parameters. The comprehensive experimental results with other comparison models show that the EFAMVA model achieved the best index score, with the MSE value of 0.750, the RMSE value of 1.215, and the MAE value of 0.955. Therefore, it can be shown that the EFAMVA model has a better predictive effect on the future destination of the vehicle.
更多查看译文
关键词
Predictive models,Data models,Vehicle driving,Trajectory,Feature extraction,Mathematical models,Load modeling,Variational autoencoder,vehicle driving data,vehicle travel destination prediction,attentional mechanisms
AI 理解论文
溯源树
样例
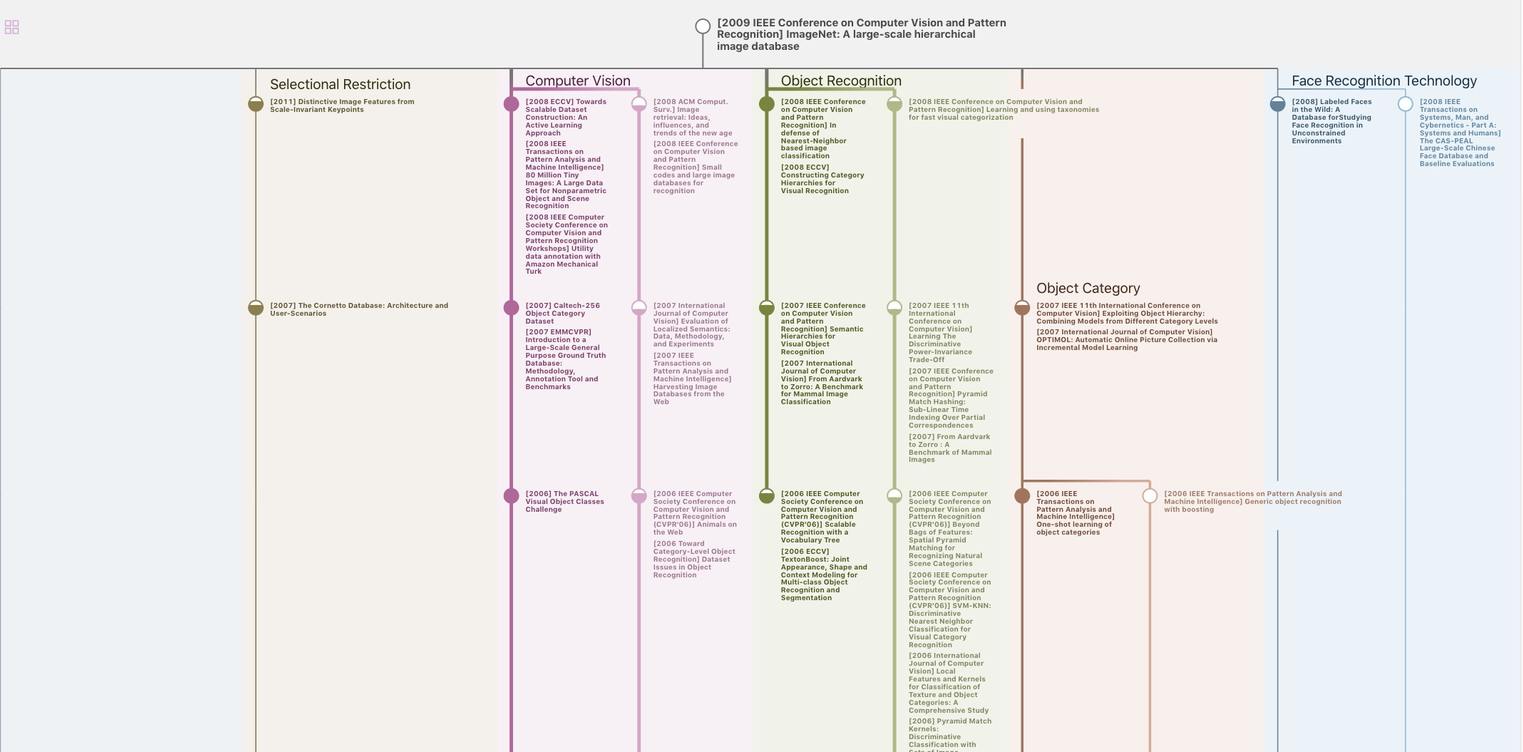
生成溯源树,研究论文发展脉络
Chat Paper
正在生成论文摘要