Make More Connections: Urban Traffic Flow Forecasting with Spatiotemporal Adaptive Gated Graph Convolution Network
ACM TRANSACTIONS ON INTELLIGENT SYSTEMS AND TECHNOLOGY(2022)
摘要
Urban traffic flow forecasting is a critical issue in intelligent transportation systems. Due to the complexity and uncertainty of urban road conditions, how to capture the dynamic spatiotemporal correlation and make accurate predictions is very challenging. In most of existing works, urban road network is often modeled as a fixed graph based on local proximity. However, such modeling is not sufficient to describe the dynamics of the road network and capture the global contextual information. In this paper, we consider constructing the road network as a dynamic weighted graph through attention mechanism. Furthermore, we propose to seek both spatial neighbors and semantic neighbors to make more connections between road nodes. We propose a novel Spatiotemporal Adaptive Gated Graph Convolution Network (STAG-GCN) to predict traffic conditions for several time steps ahead. STAG-GCN mainly consists of two major components: (1) multivariate self-attention Temporal Convolution Network (TCN) is utilized to capture local and long-range temporal dependencies across recent, daily-periodic and weekly-periodic observations; (2) mix-hop AG-GCN extracts selective spatial and semantic dependencies within multi-layer stacking through adaptive graph gating mechanism and mix-hop propagation mechanism. The output of different components are weighted fused to generate the final prediction results. Extensive experiments on two real-world large scale urban traffic dataset have verified the effectiveness, and the multi-step forecasting performance of our proposed models outperforms the state-of-the-art baselines.
更多查看译文
关键词
Traffic forecasting,spatiotemporal data,graph neural network,urban computing
AI 理解论文
溯源树
样例
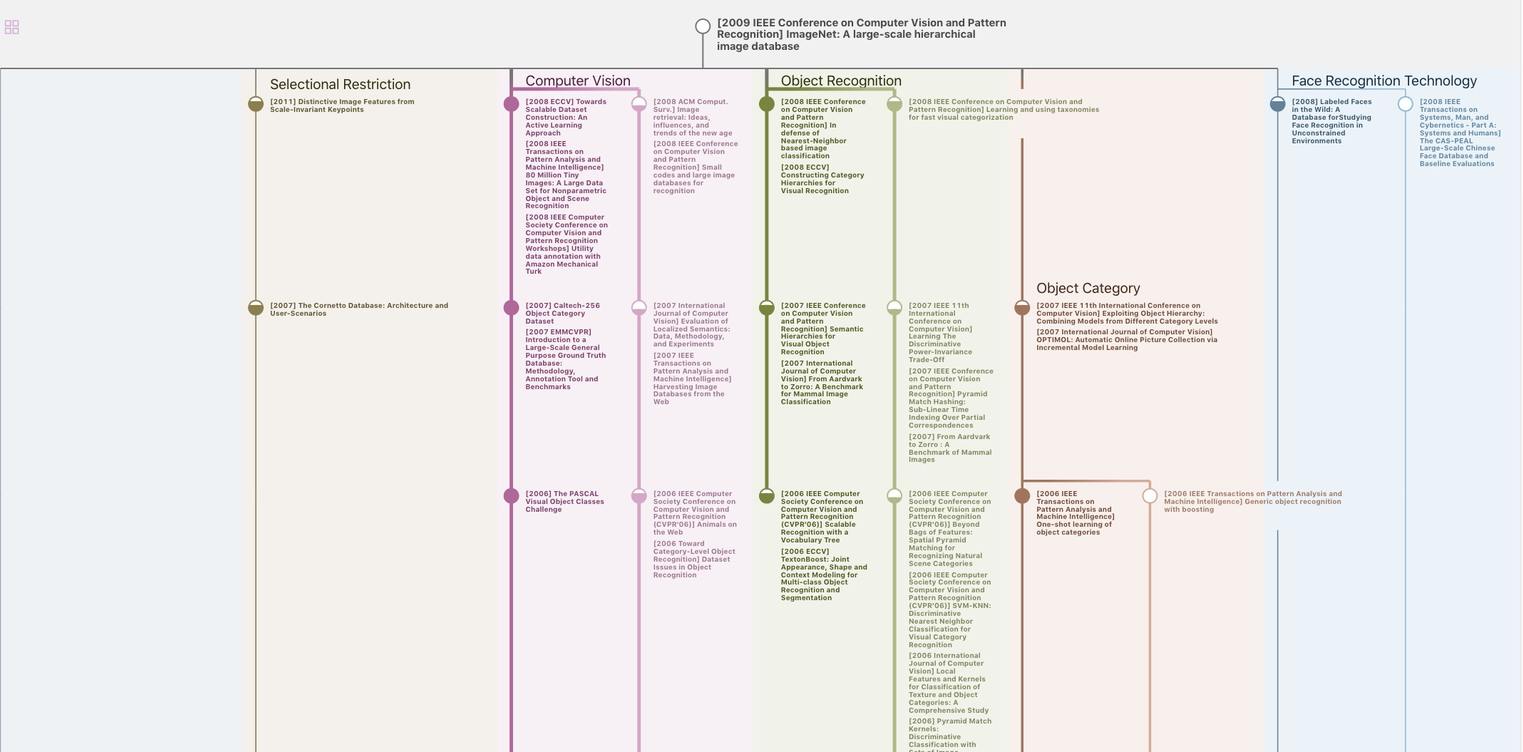
生成溯源树,研究论文发展脉络
Chat Paper
正在生成论文摘要