Online meta-learning for POI recommendation
Geoinformatica(2022)
摘要
Studying the POI recommendation in an online setting becomes meaningful because large volumes of user-POI interactions are generated in a chronological order. Although a few online update strategies have been developed, they cannot be applied in POI recommendation directly because they can hardly capture the long-term user preference only by updating the model with the current data. Besides, some latent POI information is ignored because existing update strategies are designed for traditional recommder systems without considering the addtional factors in POIs. In this paper, we propose an Online Meta-learning POI Recommendation (OMPR) method to solve the problem. To consider the geographical influences among POIs, we use a location-based self-attentive encoder to learn the complex user-POI relations. To capture the drift of user preference in online recommendation, we propose a meta-learning based transfer network to capture the knowledge transfer from both historical and current data. We conduct extensive experiments on two real-world datasets and the results show the superiority of our approaches in online POI recommendation.
更多查看译文
关键词
Online learning, POI recommendation, Meta-learning
AI 理解论文
溯源树
样例
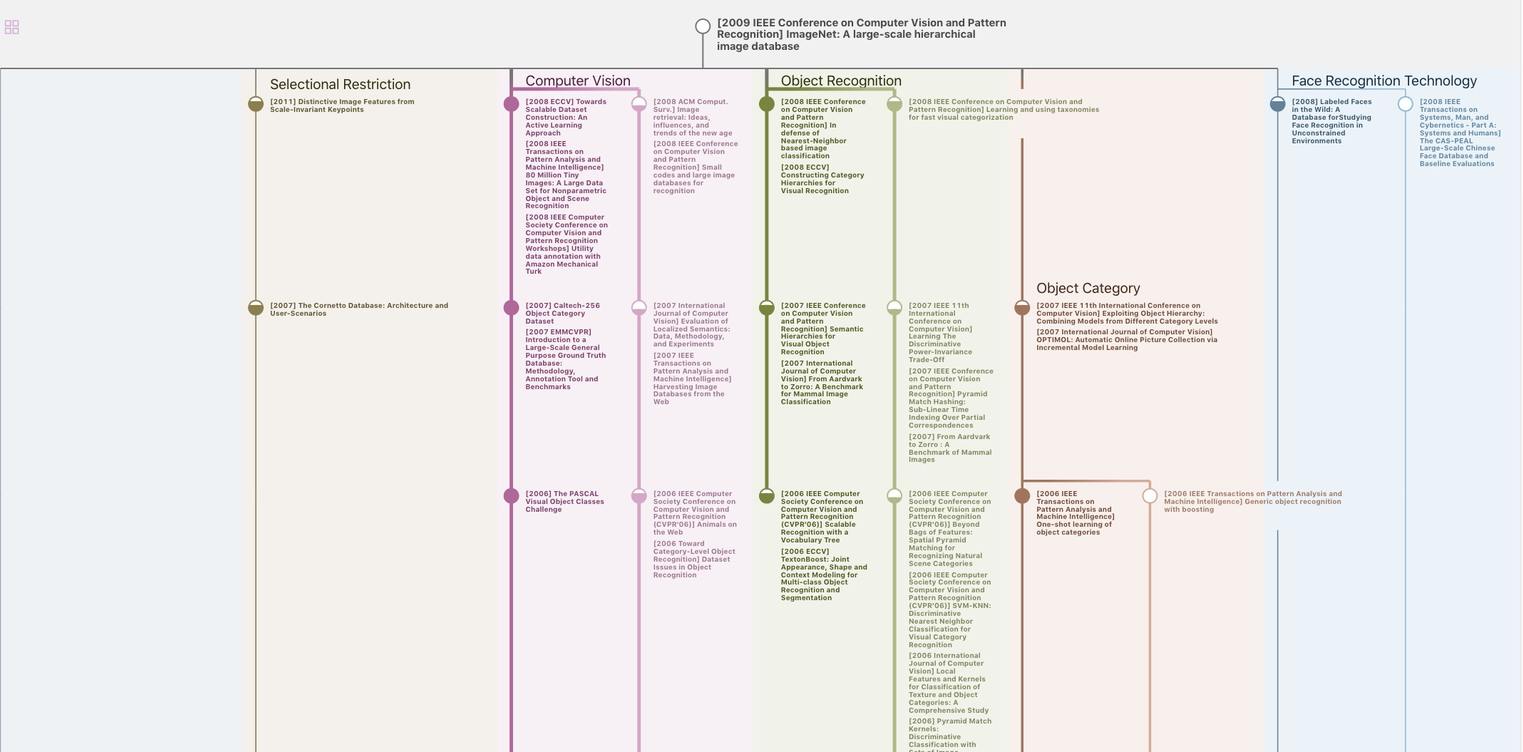
生成溯源树,研究论文发展脉络
Chat Paper
正在生成论文摘要