iNL: Implicit non-local network q
Neurocomputing(2022)
摘要
The attention mechanism of computer vision represented by a non-local network improves the performance of numerous vision tasks while bringing computational burden for deployment Wang et al. (2018). In this work, we explore to release the inference computation for non-local network by decoupling the training/inference procedure. Specifically, we propose the implicit non-local network (iNL). During training, iNL models the dependency between features across long-range affinities like original non-local blocks; during inference, iNL could be reformulated as only two convolution layers but can rival non-local network. In this way, the computation complexity and the memory costs are reduced. In addition, we take a further step and extend our iNL into a more generalized form, which covers the attentions of different orders in computer vision tasks. iNL brings steady improvements on multiple benchmarks of different vision tasks including classification, detection, and instance segmentation. In the meantime, it provides a brand-new perspective to understand the attention mechanism in deep neural networks. (c) 2022 Elsevier B.V. All rights reserved.
更多查看译文
关键词
Attention,Computation cost,Implicit method,Generalized form
AI 理解论文
溯源树
样例
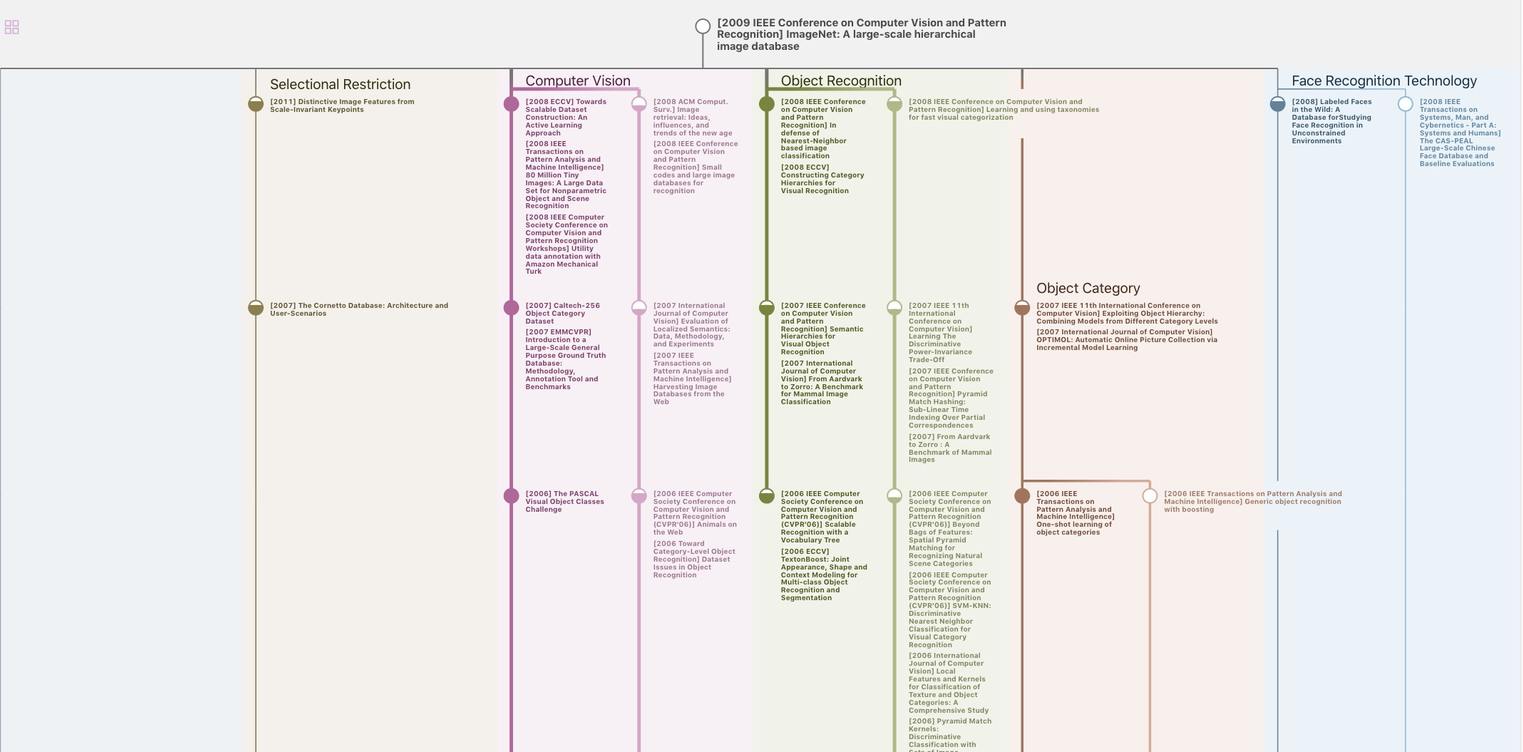
生成溯源树,研究论文发展脉络
Chat Paper
正在生成论文摘要