Heterogeneous star graph attention network for product attributes prediction
Advanced Engineering Informatics(2022)
摘要
The target of product attributes prediction is to complete the characteristics set for defining a particular product. Most of the existing methods treat the product attributes prediction as a Named-Entity Recognition (NER) problem from the products’ affiliated data, such as product title and introduction. However, in a large number of industrial applications of Alibaba, we found that the existing methods are good at concrete attributes extraction (e.g., color, size) but short of abstract attributes extraction (e.g., applicable event). Moreover, these abstract attributes are usually not easy to extract from the products’ affiliated data. In this paper, we propose a novel heterogeneous star graph attention network called “SAN”, which incorporates the advantages of multiple information in the e-commerce scene to predict the abstract attributes of products. Specifically, we model the customer interactive behaviors, product title and concrete attributes of a product as a star graph. Then, we extract the node features, node types and graph structure information from the heterogeneous star graph network which consists of n star graphs. By leveraging the parallel multiple attention mechanism, SAN can aggregate features and learn weights of nodes for product representation and abstract attribute prediction. Extensive experimental results of a real-world e-commerce dataset have demonstrated that SAN outperforms state-of-the-art methods significantly for product attributes prediction. These series of solutions are already planned for use in the applications of Alibaba.
更多查看译文
关键词
Heterogeneous graph attention network,Product attributes prediction,E-commerce,Parallel attention
AI 理解论文
溯源树
样例
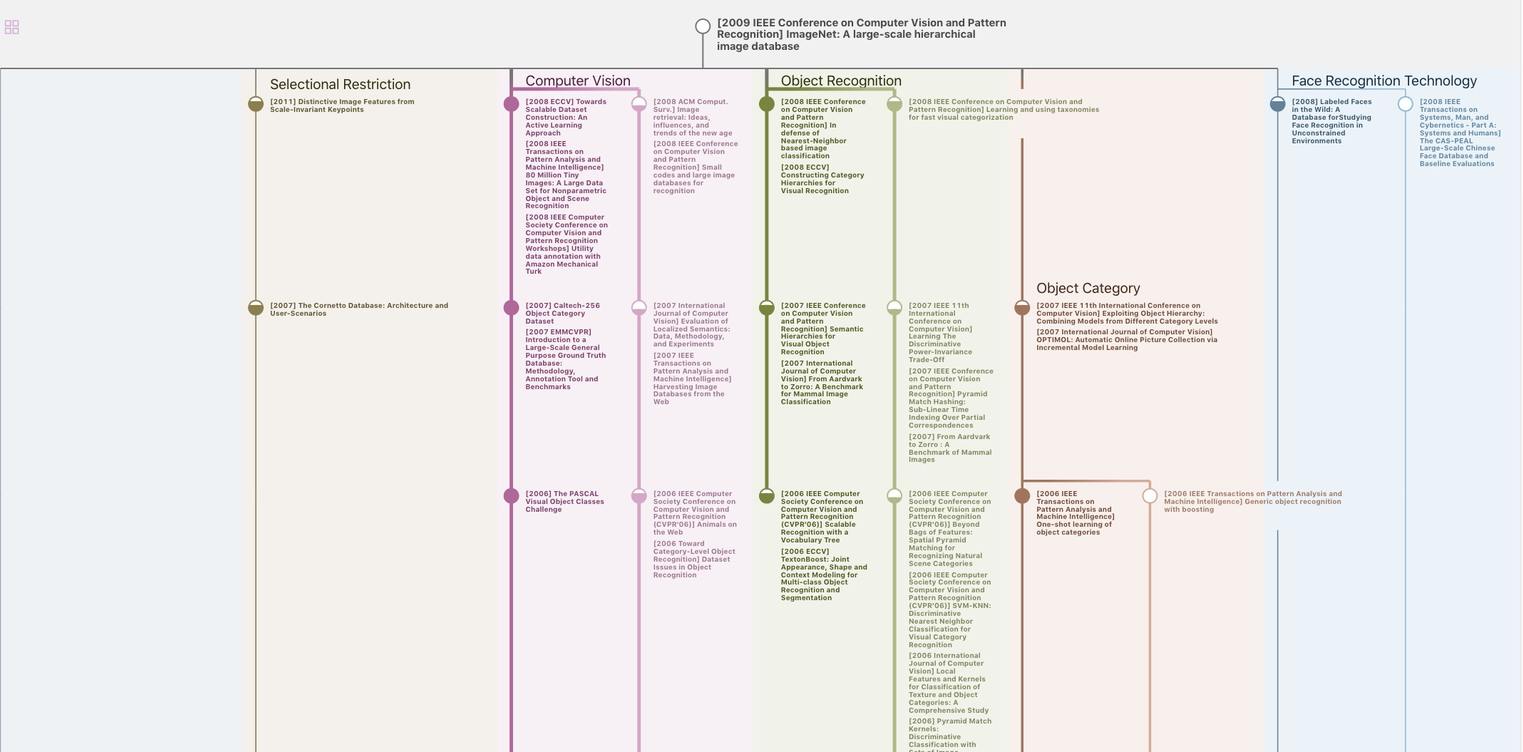
生成溯源树,研究论文发展脉络
Chat Paper
正在生成论文摘要