Global-Guided Asymmetric Attention Network for Image-Text Matching
Neurocomputing(2022)
摘要
Image-text matching is a vital yet challenging task in the field of vision and language. Unlike previous methods that usually adopt a symmetrical network to independently embed images and sentences into a joint latent space, we propose a novel Global-guided Asymmetric Attention Network (GAAN) to represent the two modalities more comprehensively. Specifically, we first design a Global Information-guided Transformer Encoder (GITE) to effectively mitigate the drawback of the lack of contextual information of the region features. Taking full advantage of the image global information, GITE is able to model the regional relations and region-global relations simultaneously, so as to obtain a more accurate visual representation. Then, we adopt a Textual Self-Attention (TSA) module to explore the word-word relations and produce the context-aware word representations. Finally, we deploy an Image-guided Textual Attention (ITA) module to explore the fine-grained correspondence between image regions and sentence words. By using context-aware visual information to guide textual representation learning, we can build asymmetric connections between vision and language to better exploit textual information. Experimental results on two benchmark datasets including MSCOCO and Flickr30k show that GAAN significantly surpasses state-of-the-art methods.
更多查看译文
关键词
Image-text matching,Asymmetric relation modeling,Self-attention,Cross-attention
AI 理解论文
溯源树
样例
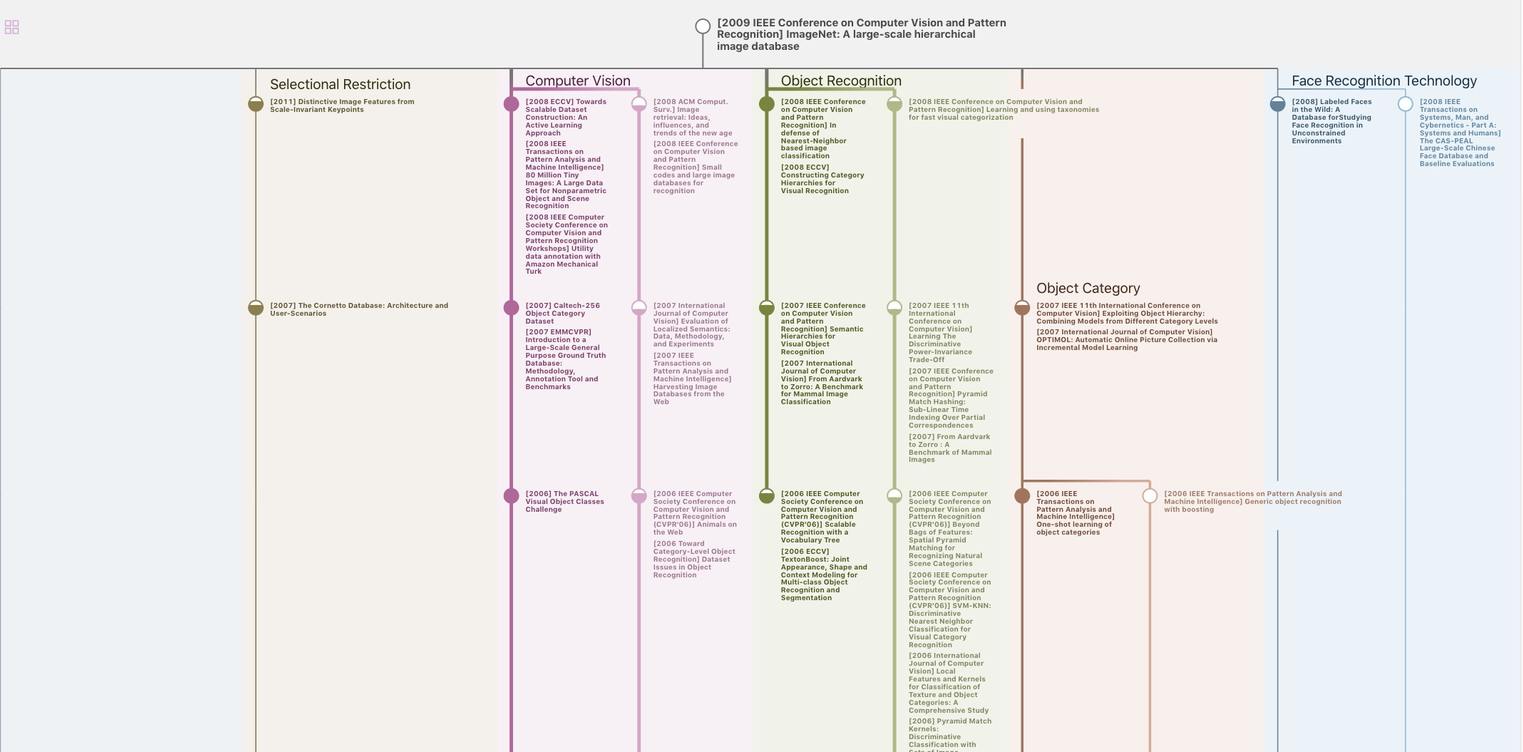
生成溯源树,研究论文发展脉络
Chat Paper
正在生成论文摘要