A Deep Learning Approach to Predict Conductive Hearing Loss in Otitis Media with Effusion Using Otoscopic Images
Social Science Research Network(2022)
摘要
Background: Otitis media with effusion (OME) is one of the most common causes of acquired conductive hearing loss. However, hearing thresholds are often difficult to obtain. We aimed to test whether deep learning techniques could predict conductive hearing loss from otoscopic images and secondly whether a logistic regression model based on tympanic membrane features could predict conductive hearing loss.Methods: A deep learning model was developed and validated from 2790 otoscopic images sourced from multiple-centers. The deep learning model was developed to predict an averaged air-bone gap (ABG) > 10 dB at 0.5k, 1k, 2k, and 4k Hz. Conductive hearing loss prediction was evaluated using the area under the receiver operation curve (AUC), accuracy, and F1score. A logistic regression model based on otoscopic findings and three otologists were compared with the deep learning model. Findings: This deep learning model provided an AUC of 0.75, accuracy of 81%, and F1 score of 0.89. The performance of three otologists was worse, with accuracies of 16%, 30%, 39%, and F1 scores of 0.09, 0.18, 0.25 respectively. The logistic regression model provided an AUC of 0.60, accuracy of 76%, and F1 score of 0.82. Furthermore, the deep learning model took 2.5s to complete its prediction in 205 otoscopic images, whilst the three otologists spent 633s, 645s, and 692s respectively.Interpretation: This deep learning model could be incorporated into the OME route hearing test workflow to enable fast and convenient evaluation of conductive hearing loss.Funding Information: This work was funded by Medical artificial intelligence project of Sun Yat-Sen Memorial Hospital (YXYGZN201904), Key R&D Program of Guangdong Province, China (Grant No. 2018B030339001), National Natural Science Foundation of China (Grant No. 82071062) and Guangdong Basic and Applied Basic Research Foundation (Grant No. 2021A1515012038).Declaration of Interests: All the authors have nothing to disclose.Ethics Approval Statement: This study was approved by the institutional review board of Sun Yat-sen Memorial Hospital (reference number SYSEC-KY-KS-2021-191).
更多查看译文
AI 理解论文
溯源树
样例
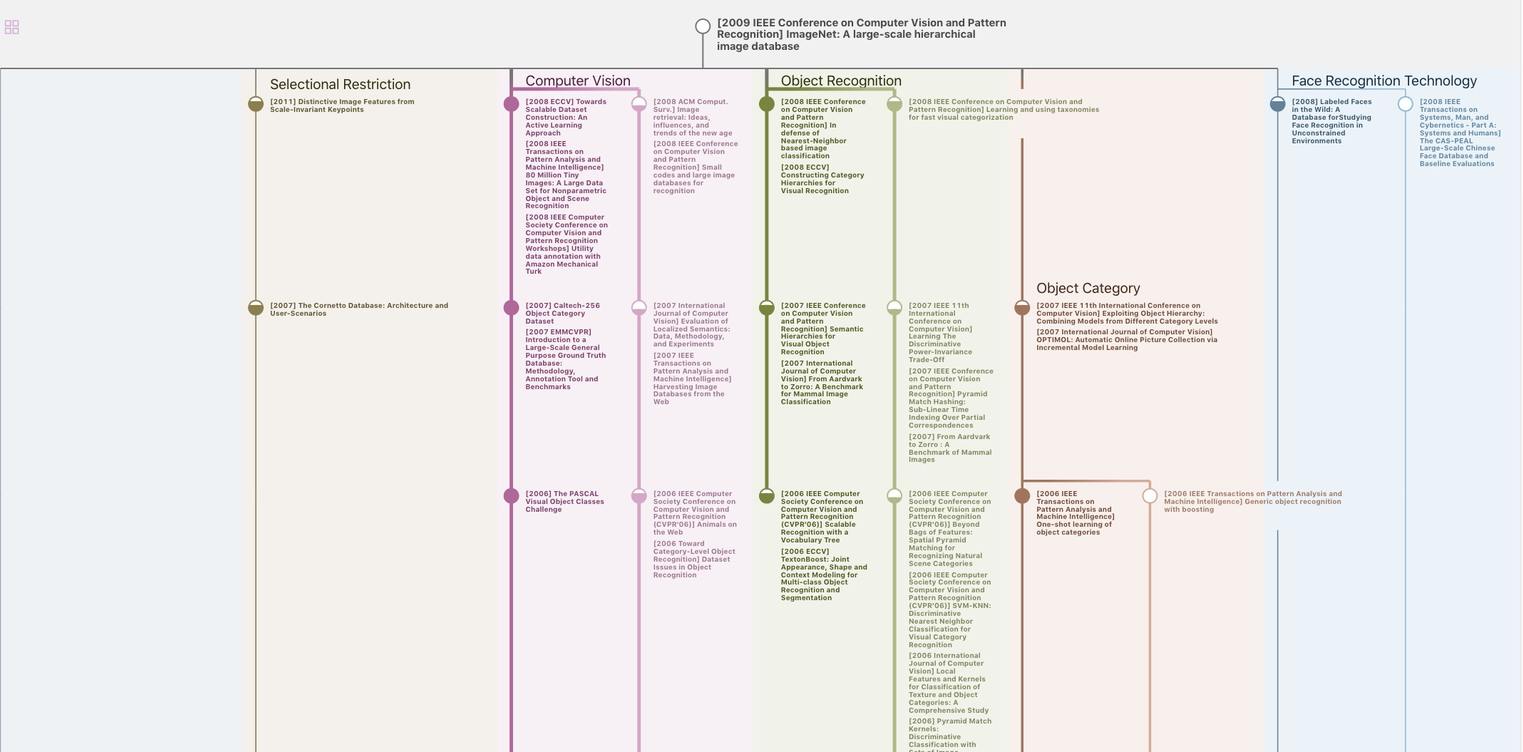
生成溯源树,研究论文发展脉络
Chat Paper
正在生成论文摘要