TAS2-Net: Triple Attention Semantic Segmentation Network for Small Surface Defect Detection
IEEE Transactions on Instrumentation and Measurement(2022)
摘要
Surface defect detection plays a vital role in manufacturing. However, it remains challenging: 1) small samples due to fewer defective products relative to numerous normal products and 2) small defects due to the small size of the defect relative to the large surface. In order to address these problems, this article proposes a triple attention semantic segmentation network for pixel-wise segmentation of surface defects, named as TAS 2 -Net. In the TAS 2 -Net framework, for solving the issue of small samples, a generative adversarial network (GAN)-based data augmentation method is proposed to generate defect training samples from a limited number of true defects. Furthermore, for avoiding the loss of small defects, a multilevel features extraction (MFE) module is proposed to extract multi-level features of small defects. Then, a triple context attention module is designed to capture more defect context information for better identifying these small defects. Finally, the focus context module (FCM) is utilized to fuse defect information and achieve pixel-wise segmentation on small defects. Experiments on three actual defect datasets show that the proposed TAS 2 -Net outperforms the state-of-the-art methods in terms of precision, recall, ${F}$ -measure, and Intersection over Union (IoU) (DAGM: 86.9%, NEU-DET: 86.3%, Magnetic-Tile: 85.1%).
更多查看译文
关键词
Feature extraction, Semantics, Image segmentation, Training, Deep learning, Data mining, Generative adversarial networks, Deep learning, semantic segmentation, small defects, small samples, surface defect detection
AI 理解论文
溯源树
样例
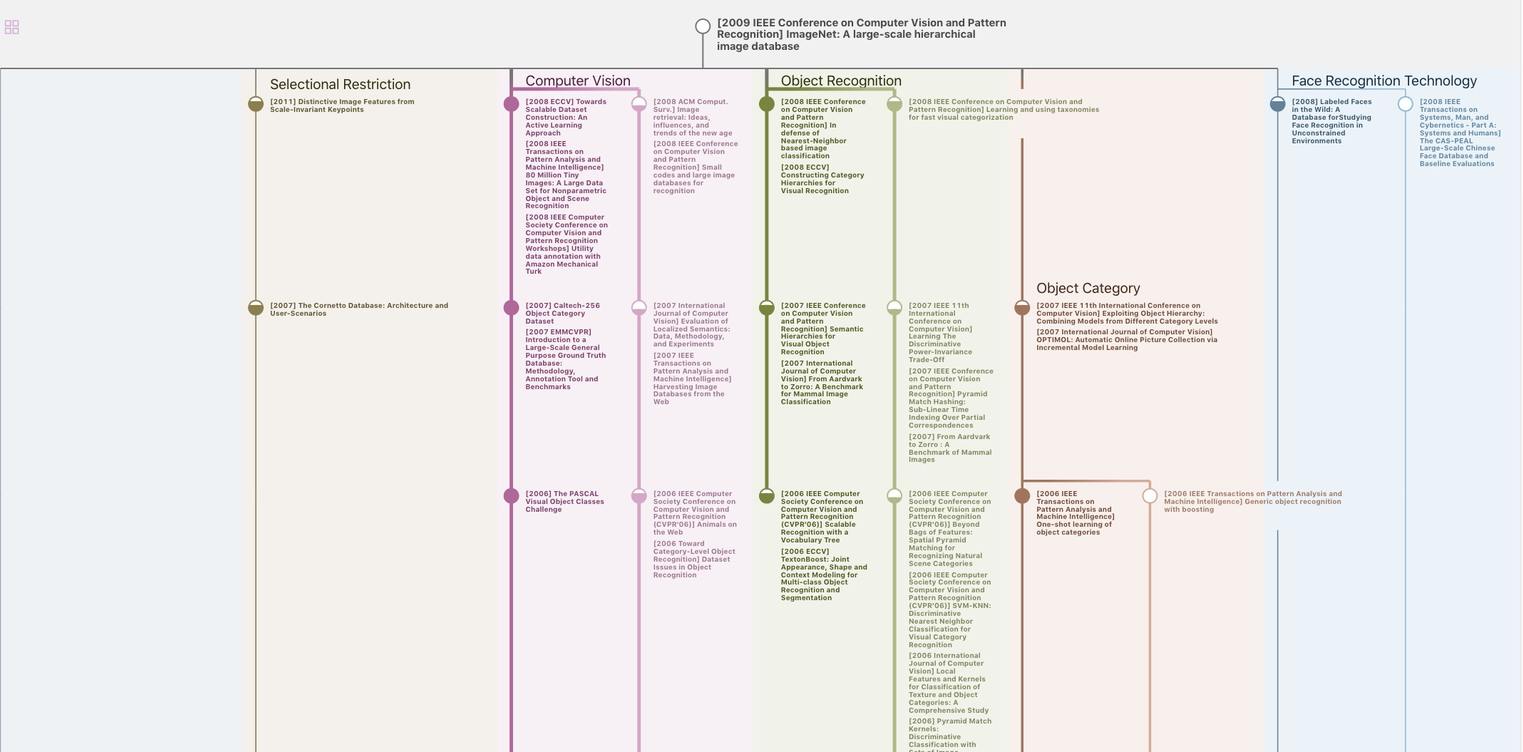
生成溯源树,研究论文发展脉络
Chat Paper
正在生成论文摘要