A survey of the extraction and applications of causal relations
NATURAL LANGUAGE ENGINEERING(2022)
摘要
Causationin written natural language can express a strong relationship between events and facts. Causation in the written form can be referred to as a causal relation where a cause event entails the occurrence of an effect event. A cause and effect relationship is stronger than a correlation between events, and therefore aggregated causal relations extracted from large corpora can be used in numerous applications such as question-answering and summarisation to produce superior results than traditional approaches. Techniques like logical consequence allow causal relations to be used in niche practical applications such as event prediction which is useful for diverse domains such as security and finance. Until recently, the use of causal relations was a relatively unpopular technique because the causal relation extraction techniques were problematic, and the relations returned were incomplete, error prone or simplistic. The recent adoption of language models and improved relation extractors for natural language such as Transformer-XL (Dai et al. (2019). Transformer-xl: Attentive language models beyond a fixed-length context. arXiv preprint arXiv:1901.02860) has seen a surge of research interest in the possibilities of using causal relations in practical applications. Until now, there has not been an extensive survey of the practical applications of causal relations; therefore, this survey is intended precisely to demonstrate the potential of causal relations. It is a comprehensive survey of the work on the extraction of causal relations and their applications, while also discussing the nature of causation and its representation in text.
更多查看译文
关键词
Causal relations,Survey,Sentiment,Event prediction,Information retrieval,Cause identification
AI 理解论文
溯源树
样例
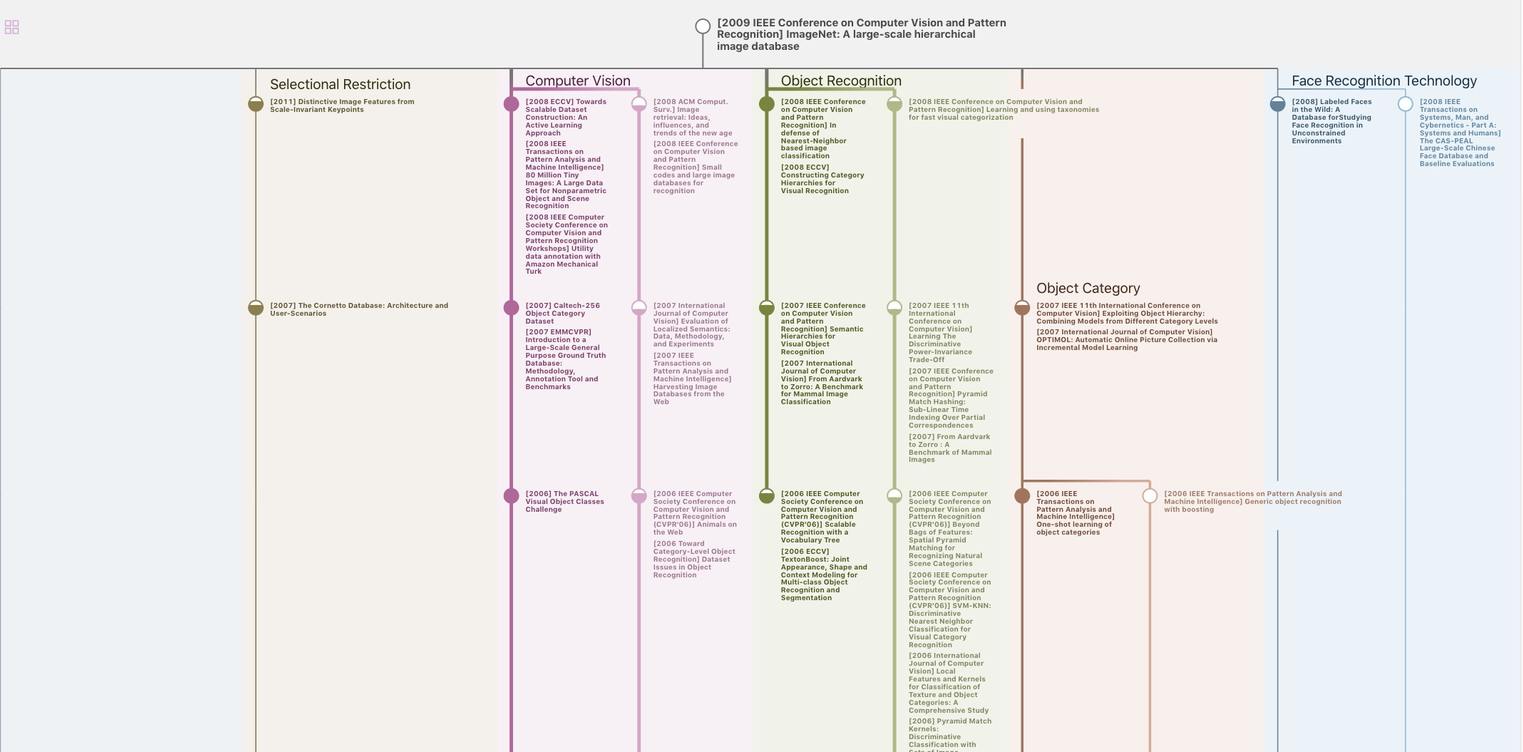
生成溯源树,研究论文发展脉络
Chat Paper
正在生成论文摘要