Deep boundary-aware clustering by jointly optimizing unsupervised representation learning
Multimedia Tools and Applications(2022)
摘要
Deep clustering obtains feature representation generally and then performs clustering for high dimension real-world data. However, conventional solutions are two-stage embedding learning-based methods and these two processes are separate and independent, which often leads to clustering results cannot feedback to optimize the representation learning and reduces the performance of deep clustering. In this paper, we aim to propose a deep boundary-aware clustering by jointly optimizing unsupervised representation learning. More specifically, we joint boundary-aware variational auto-encoder and deep regularized clustering for deep regularized clustering for unsupervised learning, named B oundary- a ware DE ep C lustering (BaDEC). BaDEC is able to learn feature representation and clustering simultaneously, and it introduces deep regularized clustering to reduce the unreliability of the similarity measures. In particular, we present a boundary-aware variational auto-encoder that tunes variable evidence lower bounds flexibly to assist feature representation learning better for more accurate clustering. Extensive experiments on various datasets from multiple domains demonstrate that the proposed method outperforms several popular comparison baseline methods.
更多查看译文
关键词
Unsupervised representation learning,Deep clustering,Variational bounds
AI 理解论文
溯源树
样例
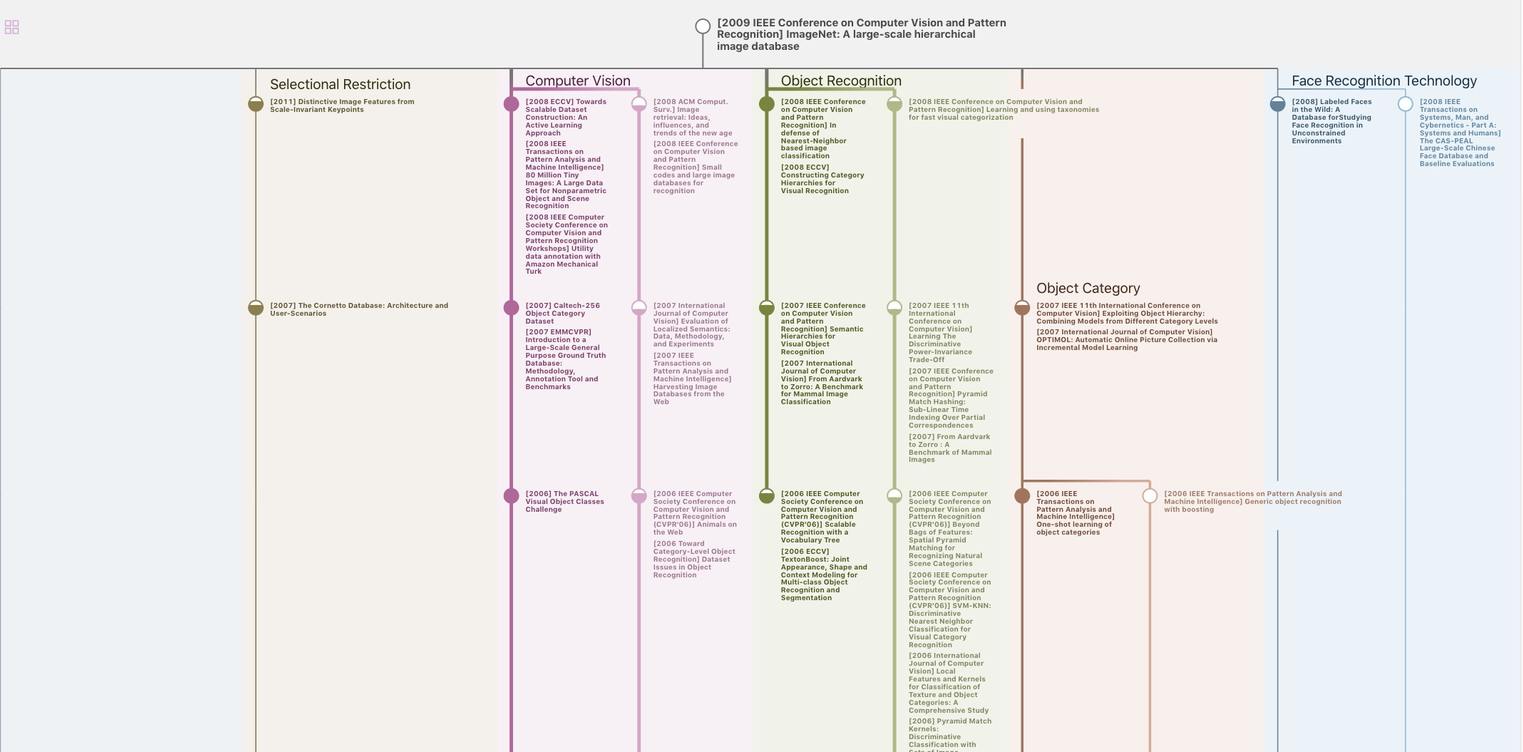
生成溯源树,研究论文发展脉络
Chat Paper
正在生成论文摘要