Machine learning constitutive models of elastomeric foams
COMPUTER METHODS IN APPLIED MECHANICS AND ENGINEERING(2022)
摘要
Flexible foams are a class of materials often used in transportation systems to mitigate mechanical shocks and vibrations. Polydispersity causes these foams to have a complex microstructure composed of a matrix polymer material and nearly spherical voids. This structure leads to a complex material response upon loading where the load for a given displacement becomes highly dependent upon the current volume fraction of voids in the deformed foam. This complex behavior makes it challenging to develop constitutive models for flexible foams where typically only the homogenized response of the foam is considered during model development, calibration, and eventual deployment. To overcome these challenges we utilize a micromechanics finite element method simulation-informed machine learning framework to develop new constitutive models with idealized foam microstructures of moderate density in mind. Several different machine learned models will be presented and validated against micromechanics finite element simulation which were absent from the training dataset. Specifically, traditional data-driven machine learned regression models will be compared with machine learned models which learn the deviation of representative volume element data from a traditional homogenized constitutive model. A discussion on the strengths and weaknesses of each of the approaches will be presented. (c) 2022 Elsevier B.V. All rights reserved.
更多查看译文
关键词
Flexible foams,Machine learning,Hyper elasticity,Neural networks,Gaussian processes
AI 理解论文
溯源树
样例
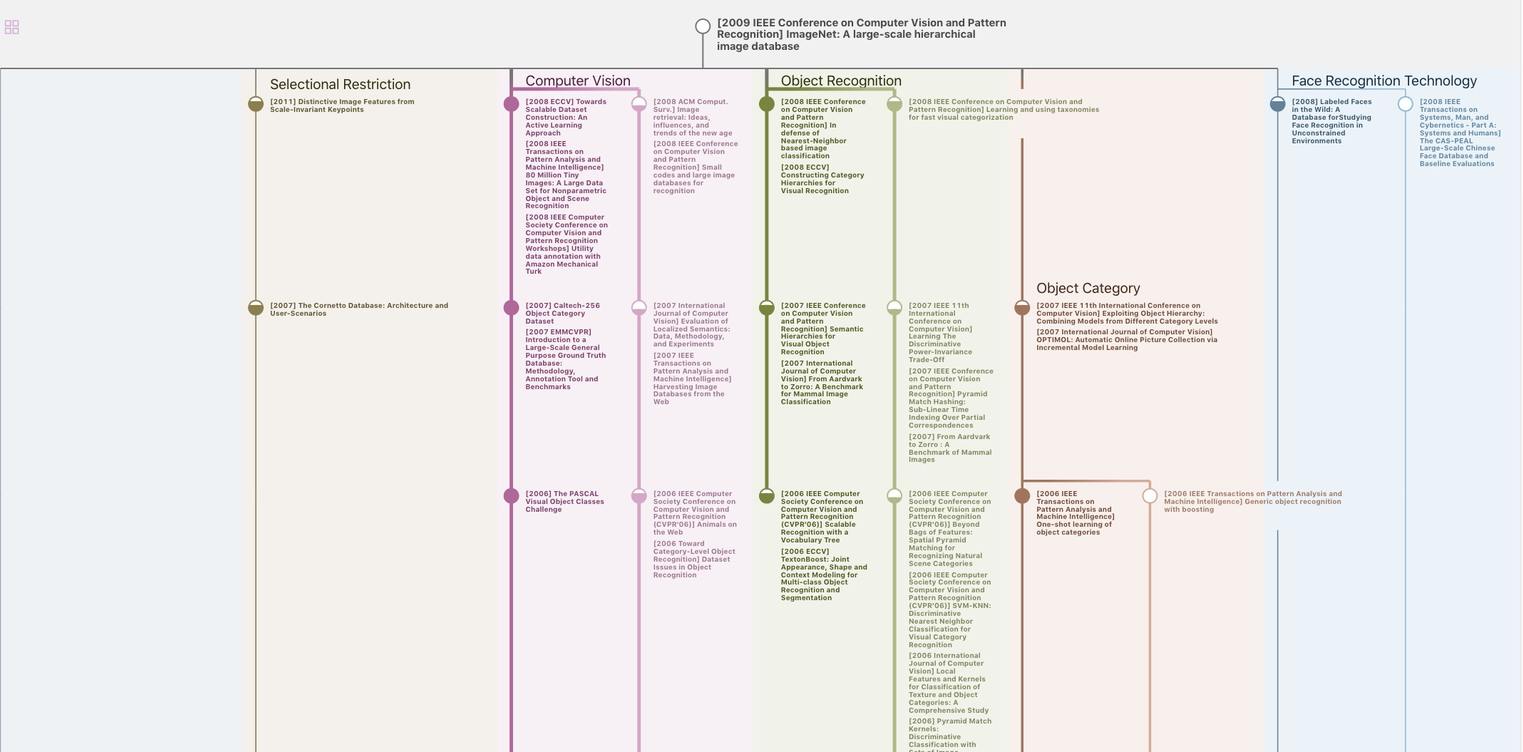
生成溯源树,研究论文发展脉络
Chat Paper
正在生成论文摘要