A Unified Structure Learning Framework for Graph Attention Networks
Neurocomputing(2022)
摘要
Graph Neural Networks (GNNs) have achieved state-of-the-art performance in many fields and attracted a lot of attention in the communit. Most Graph Neural Networks can be merely used when graph-structured data is available. However, many graph structures have noise, or data itself has no graph structures, so learning the dynamic and adaptive graph structures is necessary. In this paper, we propose a unified structure learning framework for Graph Attention Networks. Specifically, we first design a strategy to learn the graph structures. Then we develop a novel attention mechanism based on structure context information of graph and node representations. Further, we devise Structure Learning Graph Attention Networks (SLGAT) and Structure Learning Attention-based Graph Neural Networks (SLAGNN) by using the new attention mechanism on the new graph. Finally, we demonstrate that our approaches outperform competing methods on six standard datasets for the semi-supervised node classification task. (c) 2022 Elsevier B.V. All rights reserved.
更多查看译文
关键词
Graph attention networks,Graph structure learning,Semi-supervised classification
AI 理解论文
溯源树
样例
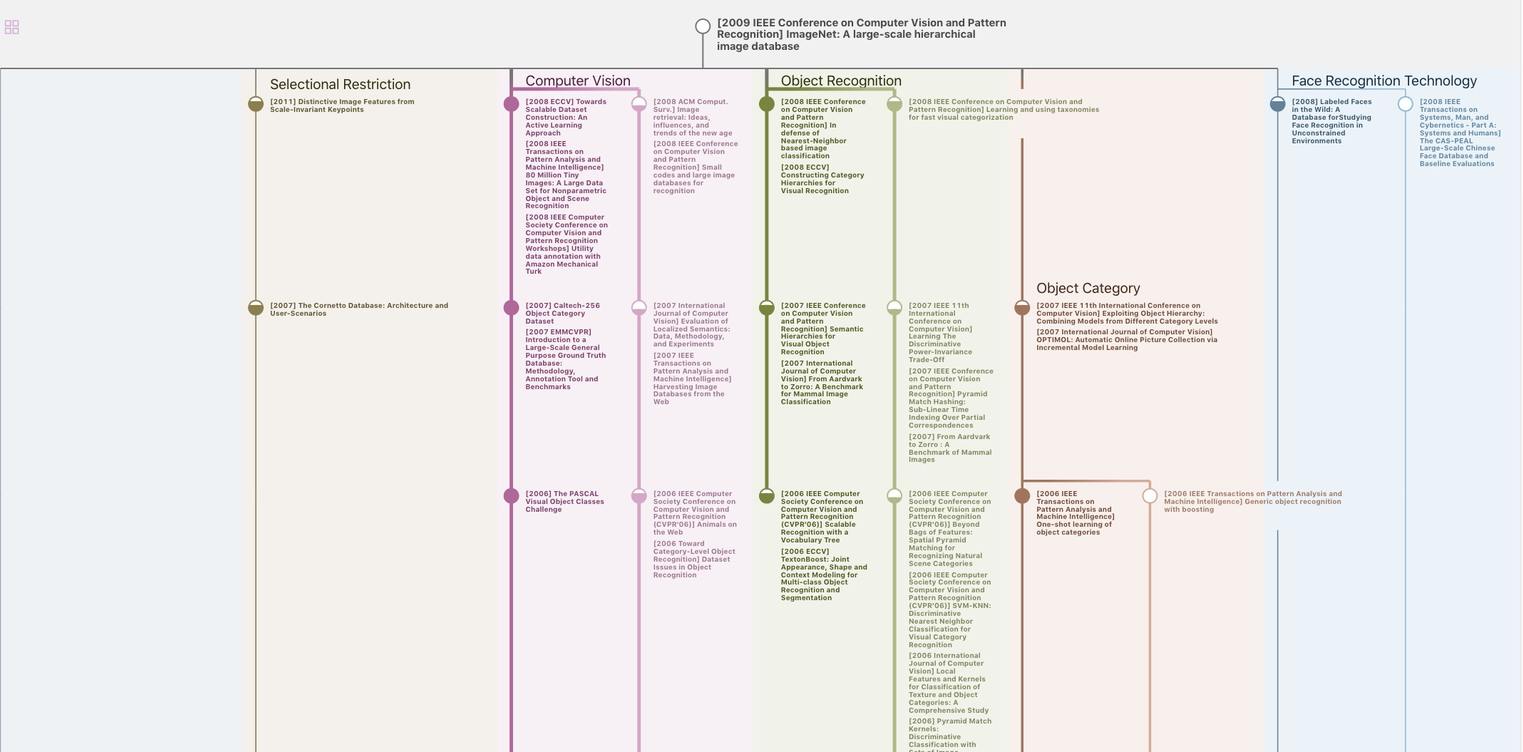
生成溯源树,研究论文发展脉络
Chat Paper
正在生成论文摘要