Transformer-Based Efficient Salient Instance Segmentation Networks With Orientative Query
IEEE Transactions on Multimedia(2023)
摘要
Salient instance segmentation (SIS) can be considered as the next generation task for the saliency detection community. Most of the existing state-of-the-art methods used for this novel challenging task are built on the mainstream Mask R-CNN architecture. However, this mechanism relies heavily on hand-designed anchors and NMS post-processing. In this paper, we provide a one stage SIS framework with transformers, termed Orientative Query Transformer (OQTR). To leverage the long-range dependencies of transformers, a cross fusion module is designed to efficiently fuse the global features in the encoder and salient query features for salient mask prediction. Furthermore, derived from the center prior in traditional saliency models, we propose an orientative query that is considered as the initial salient object query to accelerate convergence. In addition, to mitigate the issue of the lack of a large-scale dataset with salient instance labels, we collect a new SIS dataset (SIS10 K) containing over 10 K images elaborately annotated with both object- and instance-level labels to promote the community. Without any post-processing, our end-to-end OQTR framework significantly surpasses the top-1 RDPNet by an average of 13.1% AP scores across all three challenging datasets, demonstrating the strong performance of the proposed OQTR. The code and the dataset proposed in this work are available at: https://github.com/ssecv/OQTR.
更多查看译文
关键词
Salient instance segmentation,deep learning,vision transformer,attention model
AI 理解论文
溯源树
样例
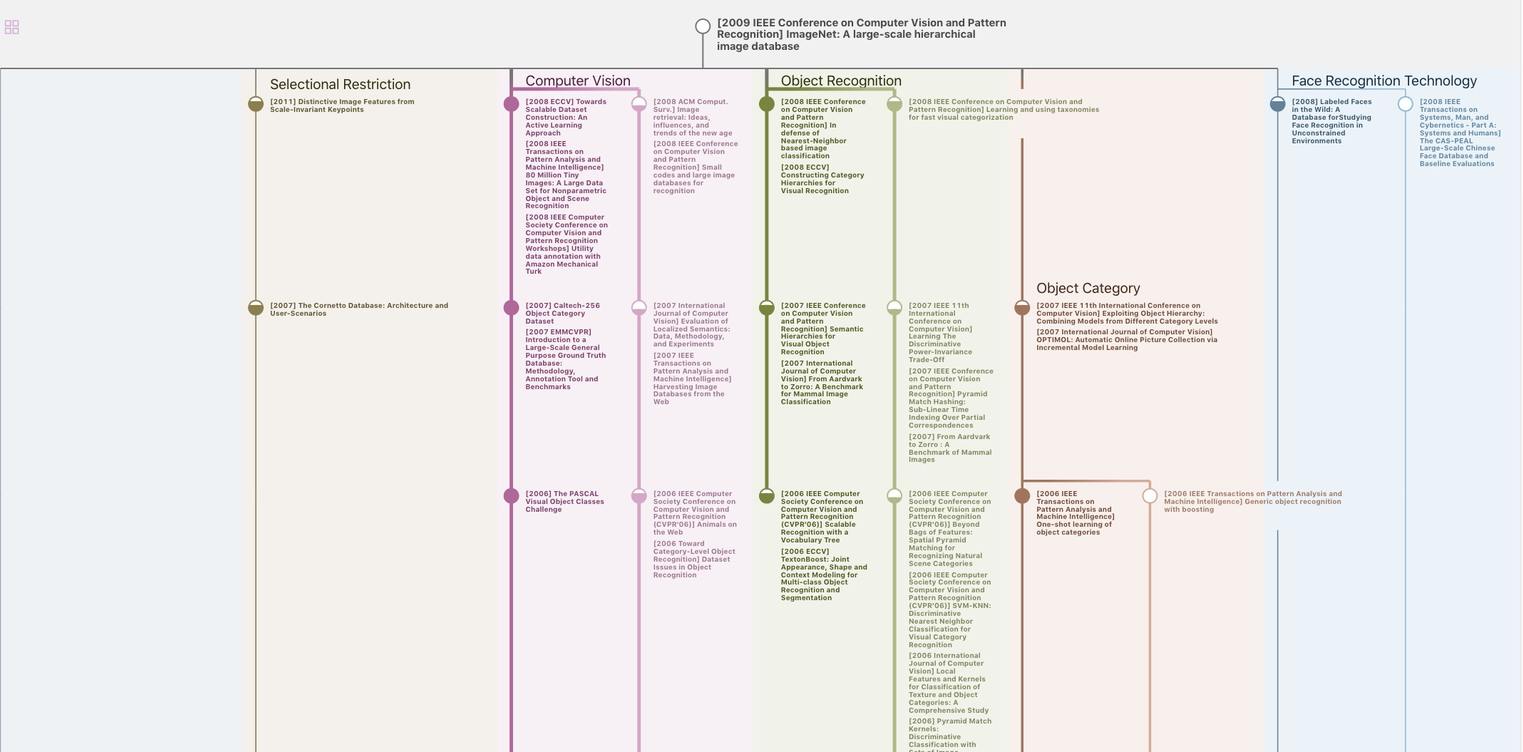
生成溯源树,研究论文发展脉络
Chat Paper
正在生成论文摘要