Distributed Machine Learning for Multiuser Mobile Edge Computing Systems
IEEE Journal of Selected Topics in Signal Processing(2022)
摘要
In this paper, we investigate a distributed machine learning approach for a multiuser mobile edge computing (MEC) network in a cognitive eavesdropping environment, where multiple secondary devices (SDs) have some tasks with different priorities to be computed. The SDs can be allowed to use the wireless spectrum as long as the interference to the primary user is tolerated, and an eavesdropper in the network can overhear the confidential message from the SDs, which threatens the data offloading. For the considered system, we firstly present three optimization criteria, whereas
criterion I
aims to minimize the linear combination of latency and energy consumption,
criterion II
tries to minimize the latency under a constraint on the energy consumption, and
criterion III
is to minimize the energy consumption under a constraint on the latency. We then exploit a federated learning framework to solve these optimization problems, by optimizing the offloading ratio, bandwidth and computational capability allocation ratio. Simulation results are finally demonstrated to show that the proposed method can effectively reduce the system cost in terms of latency and energy consumption, and meanwhile ensure more bandwidth and computational capability allocated to the user with a higher taskpriority.
更多查看译文
关键词
Computational offloading,federated learning,resource allocation,task priority
AI 理解论文
溯源树
样例
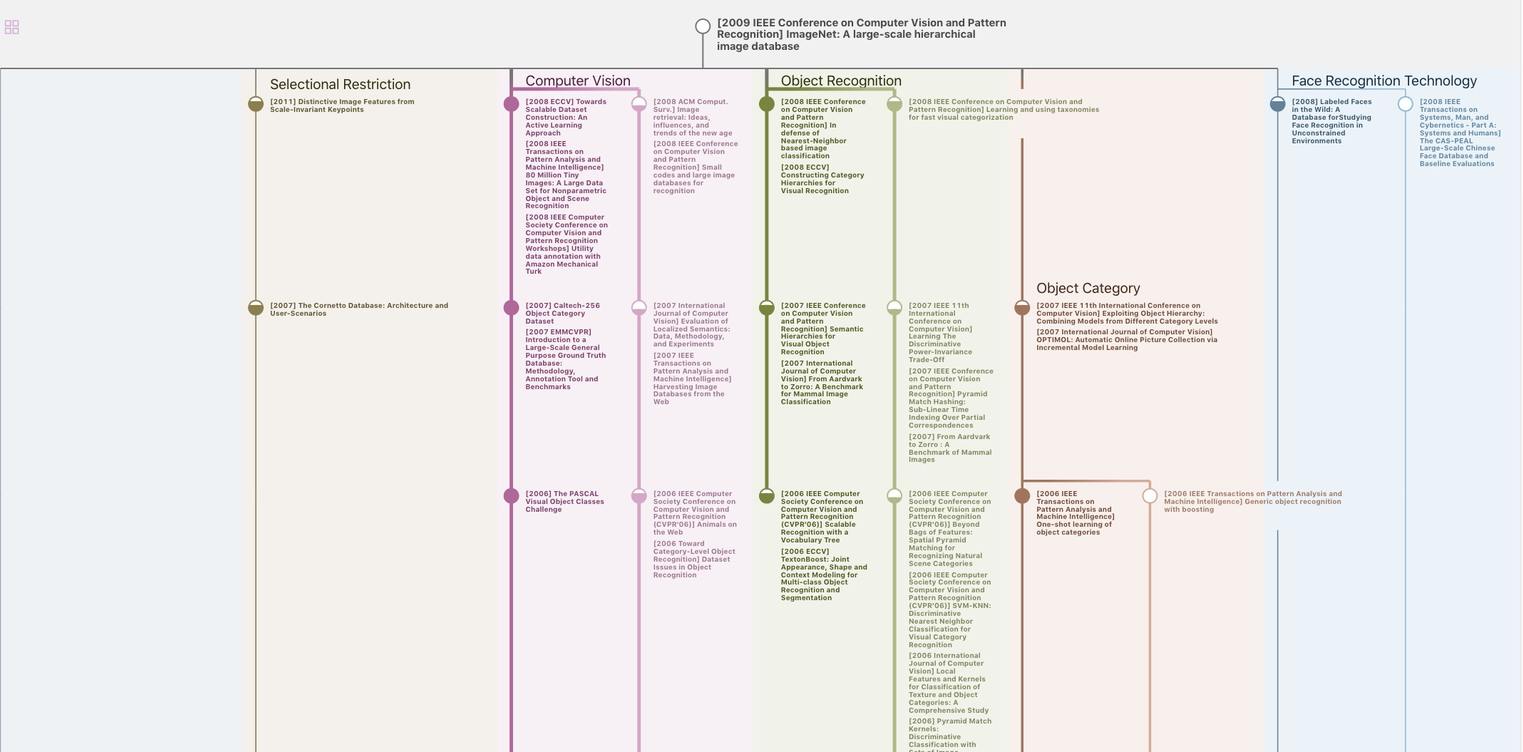
生成溯源树,研究论文发展脉络
Chat Paper
正在生成论文摘要