A Geometric Model-Based Approach to Hand Gesture Recognition
IEEE Transactions on Systems, Man, and Cybernetics: Systems(2022)
摘要
Arm-and-hand tracking by technological means allows gathering data that can be elaborated for determining gesture meaning. To this aim, machine learning (ML) algorithms have been mostly investigated looking for a balance between the highest recognition rate and the lowest recognition time. However, this balance comes mainly from statistical models, which are challenging to interpret. In contrast, we present
$\mu C^{1}$
and
$\mu C^{2}$
, two geometric model-based approaches to gesture recognition which support the visualization and geometrical interpretation of the recognition process. We compare
$\mu C^{1}$
and
$\mu C^{2}$
with respect to two classical ML algorithms, k-nearest neighbor (k-NN) and support vector machine (SVM), and two state-of-the-art (SotA) deep learning (DL) models, bidirectional long short-term memory (BiLSTM) and gated recurrent unit (GRU), on an experimental dataset of ten gesture classes from the Italian Sign Language (LIS), each repeated 100 times by five inexperienced non-native signers, and gathered with wearable technology (a sensory glove and inertial measurement units). As a result, we achieve a compromise between high recognition rates (
$>90\%$
) and low recognition times (
$ < 0.1 {\mathrm{ s}}$
) that is adequate for human–computer interaction. Moreover, we elaborate on the algorithms’ geometric interpretation based on geometric algebra, which supports some understanding of the recognition process.
更多查看译文
关键词
Deep learning (DL),explainable artificial intelligence (XAI),geometric algebra,gesture recognition,machine learning (ML),nearest neighbor classification (NNC)
AI 理解论文
溯源树
样例
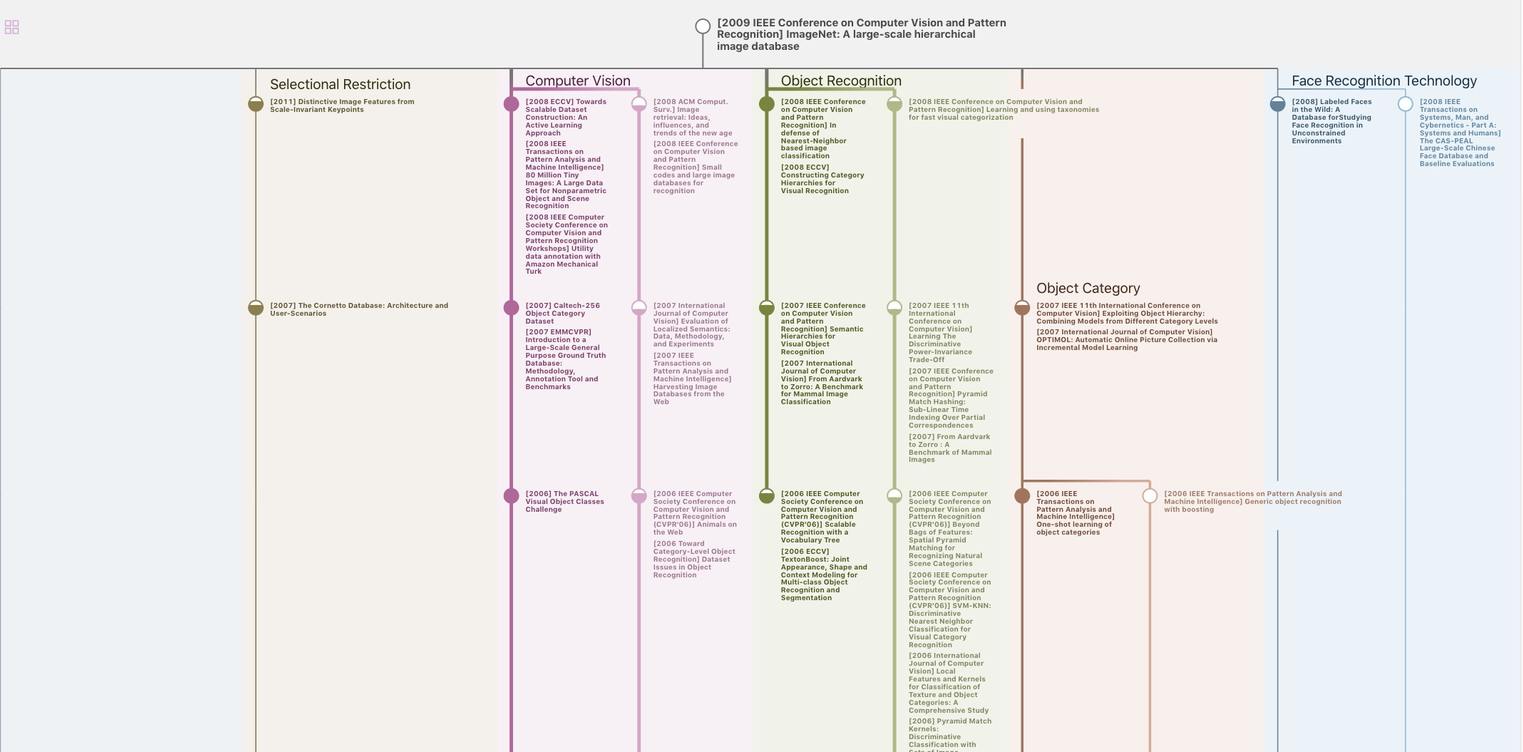
生成溯源树,研究论文发展脉络
Chat Paper
正在生成论文摘要