Weakly-supervised anomaly detection in video surveillance via graph convolutional label noise cleaning
Neurocomputing(2022)
摘要
In this paper, we seek to solve the problem of video anomaly detection under the weakly-supervised setting. Different from previous works that usually deal with the problem in a multiple-instance learning manner, we formulate it as a fully-supervised learning task with label noises. Under this new perspective, we can fully leverage the advantages of well-designed action classifiers for anomaly detection as long as the label noises are cleaned. For this purpose, we devise a graph convolutional network for label noise cleaning, which integrates two crucial characteristics for anomaly analysis: feature similarity and temporal consistency. Supervised by both direct and indirect signals, the net propagates supervision information from high-confidence snippets to low-confidence ones and provides cleaned labels for action classifier training. In this way, we design an alternate learning strategy to progressively promote the discrimination of the action classifier. During the test phase, we directly utilize the learned action classifier for anomaly detection in an end-to-end fashion without any intermediate processing. We have conducted extensive experiments on various anomaly datasets of three scales with two main types of action classifiers, and achieved superior or comparable performances compared with state-of-the-art methods. Furthermore, we manually annotate the temporal duration of anomalies in the training data of UCF-Crime, and give out the upper-limit performance of our cleaner net. The annotation can also be used as the ground truth for studying anomaly detection models under multi-level supervisory signals, which will mitigate the present shortage of large-scale anomaly datasets.
更多查看译文
关键词
Anomaly detection,Weakly-supervised learning,Graph convolutional networks,Learning with noise labels,Video action detection
AI 理解论文
溯源树
样例
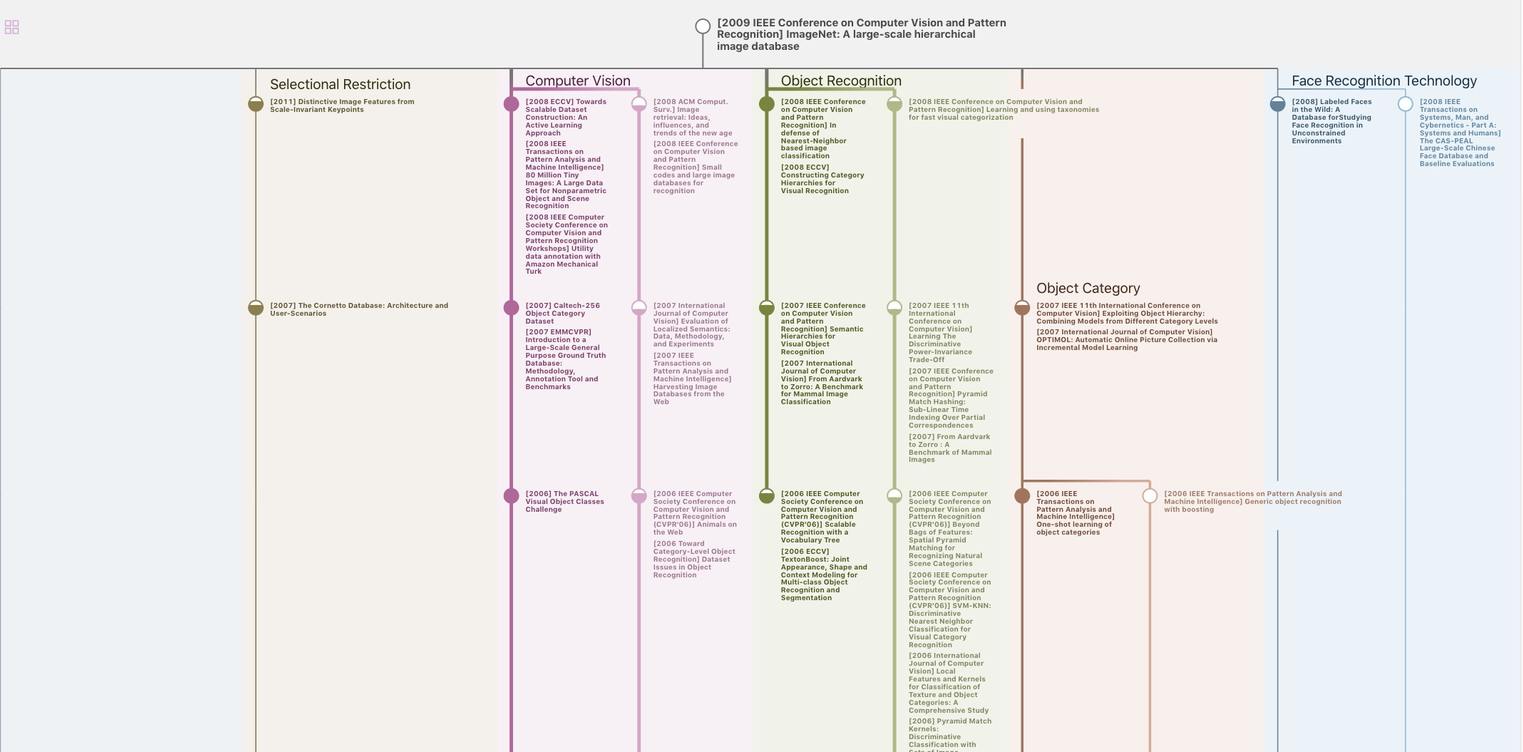
生成溯源树,研究论文发展脉络
Chat Paper
正在生成论文摘要