Adversarial learning with data selection for cross-domain histopathological breast Cancer segmentation
Multimedia Tools and Applications(2022)
摘要
Histopathology plays an important role in the clinical diagnosis of breast diseases. Early diagnosis and adjuvant therapy are of great help to patients. With the development of deep learning, fully convolutional networks (FCNs) have achieved remarkable results in the field of segmentation. However, this approach suffers from obtaining sufficient labelled data and underperforms when it comes to a new domain. Recently, adversarial learning becomes prevalent in domain adaptation, which is able to transfer learned knowledge between domains and greatly reduces the workload of labeling. In this paper, we propose a new domain adaptation method, which consists of three steps: adversarial learning, data selection and pseudo-label model refinement. Our method combines the advantages of adversarial learning and pseudo-labelling for domain adaptation. We also introduce a new data selection method to select target domain data with their pseudo-label for model refinement, considering prediction confidence and representativeness, which further strengths the model capability in target domain. We evaluate our method on private HE- and IHC-stained datasets. In order to strength the robustness, the color augmentation is utilized in this paper, the cross-domain prediction performance has been improved from 0.213 Dice to 0.703 Dice . The experimental results show that with only using unlabeled data, the proposed method can achieve 0.846 Dice on target domain, which outperforms the state-of-the-art method by 1.8%.
更多查看译文
关键词
Breast cancer segmentation,Domain adaptation,Pseudo label
AI 理解论文
溯源树
样例
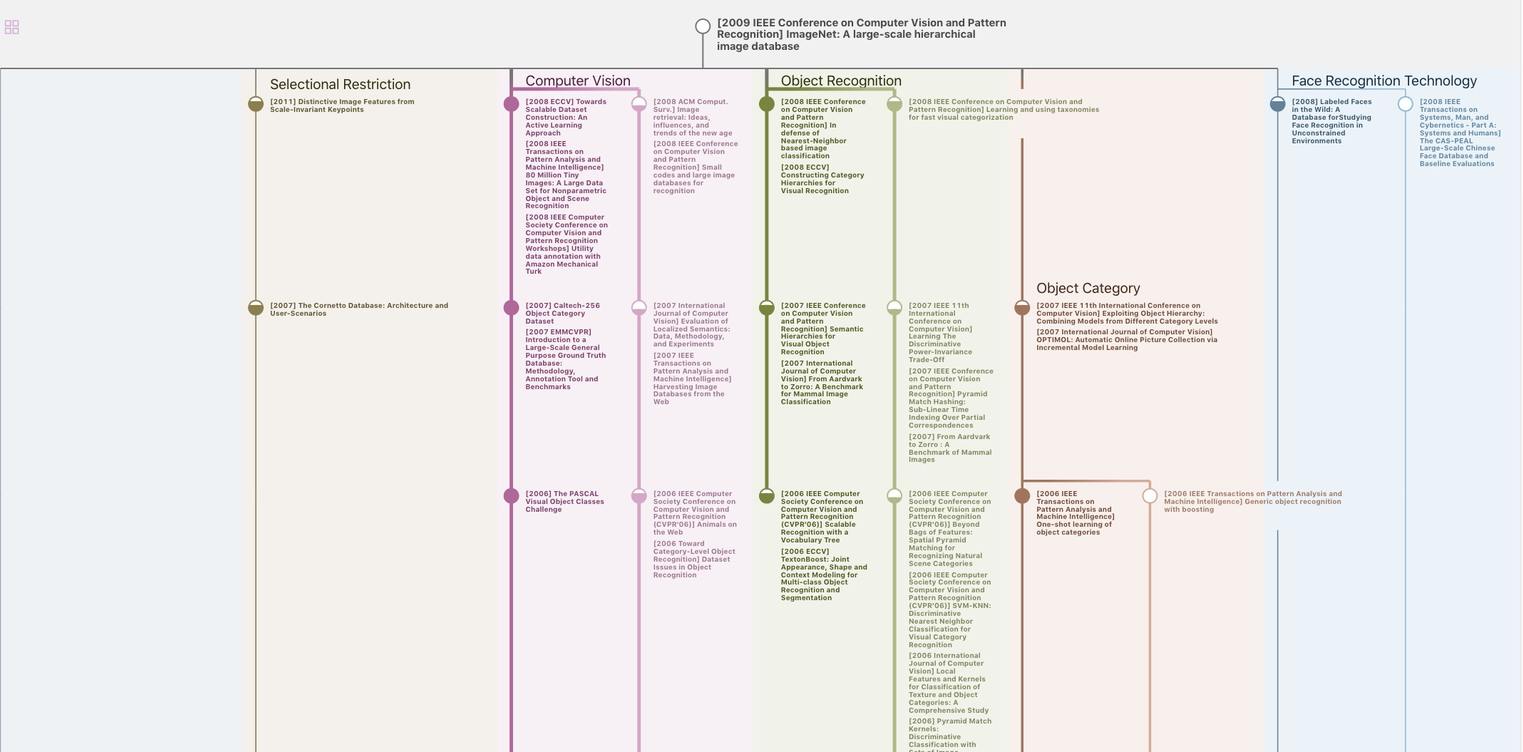
生成溯源树,研究论文发展脉络
Chat Paper
正在生成论文摘要