Video action re-localization using spatio-temporal correlation
2022 IEEE/CVF Winter Conference on Applications of Computer Vision Workshops (WACVW)(2022)
摘要
Video re-localization plays an important role in locating the moments of interest in a long videos, and is critical for a variety of applications such as surveillance video monitoring and retrieving similar archived videos for further comparison and analysis. Current re-localization approaches compute a feature vector using a video query for each video frame, and explore various feature matching techniques. These features do not capture information from varying temporal windows, and the dimension reduction to a vector leads to loss of spatio-temporal context. For efficient feature comparison and matching among thousands of videos, we design a Siamese Spatio-Temporal network comprising Convolution Neural Network and Long Short-term Memory blocks (CNN-LSTM) for feature extraction, followed by a correlation layer for spatio-temporal feature matching. We extract video features at varying temporal scales, and localize one or more segments in the reference video that semantically match the query clip. Our approach is evaluated on two benchmark datasets: AVAv2.1- Search and ActivityNet-Search. We show an improvement of over 12% in the mean average precision compared to existing approaches. We perform ablation experiments and show that the modular architecture and the holistic feature extraction expands the scope of this work to multiple video search applications.
更多查看译文
AI 理解论文
溯源树
样例
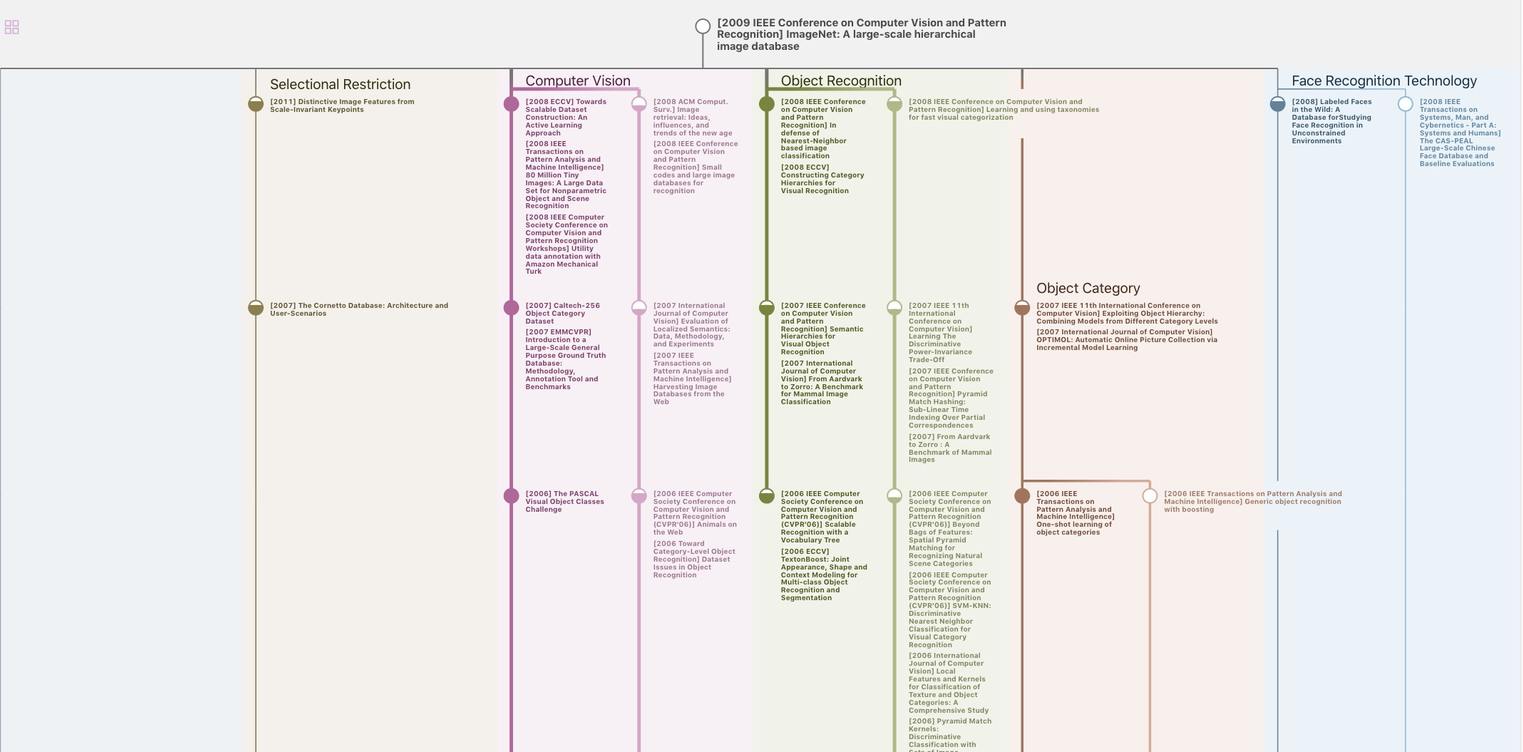
生成溯源树,研究论文发展脉络
Chat Paper
正在生成论文摘要