More or Less (MoL): Defending against Multiple Perturbation Attacks on Deep Neural Networks through Model Ensemble and Compression
2022 IEEE/CVF Winter Conference on Applications of Computer Vision Workshops (WACVW)(2022)
摘要
Deep neural networks (DNNs) have been adopted in many application domains due to their superior performance. However, their susceptibility under test-time adversarial perturbations and out-of-distribution shifts has attracted extensive research efforts. The adversarial training provides an effective defense method withstanding evolving attacking methods. However, DNNs obtained by adversarial training are usually robust to only a single type of adversarial perturbation that they are trained with. To tackle this problem, improvements have been made to incorporate multiple perturbation types into adversarial training process, but with limited flexibility in terms of perturbation types. This work investigates the design problem of deep learning (DL) systems robust to multiple perturbation attacks. To maximize flexibility, we adopt the model ensemble approach, where an ensemble of expert models dealing with various perturbation types are integrated through a trainable aggregator module. Expert models are obtained in parallel through adversarial training, targeting at respective perturbation types. Then, the aggregator module is (adversarially) trained together with fine-tuning of expert models, addressing the obfuscated gradients issue in adversarial robustness. Furthermore, in order to practically implement the robust ensemble model onto edge devices, the model compression approach is leveraged to reduce the ensemble model size. By exploring the most suitable model compression scheme, we significantly reduce the overall model size without compromising robustness. Proposed More or Less (MoL) defense outperforms state-of-the-art defenses against multiple perturbations.
更多查看译文
AI 理解论文
溯源树
样例
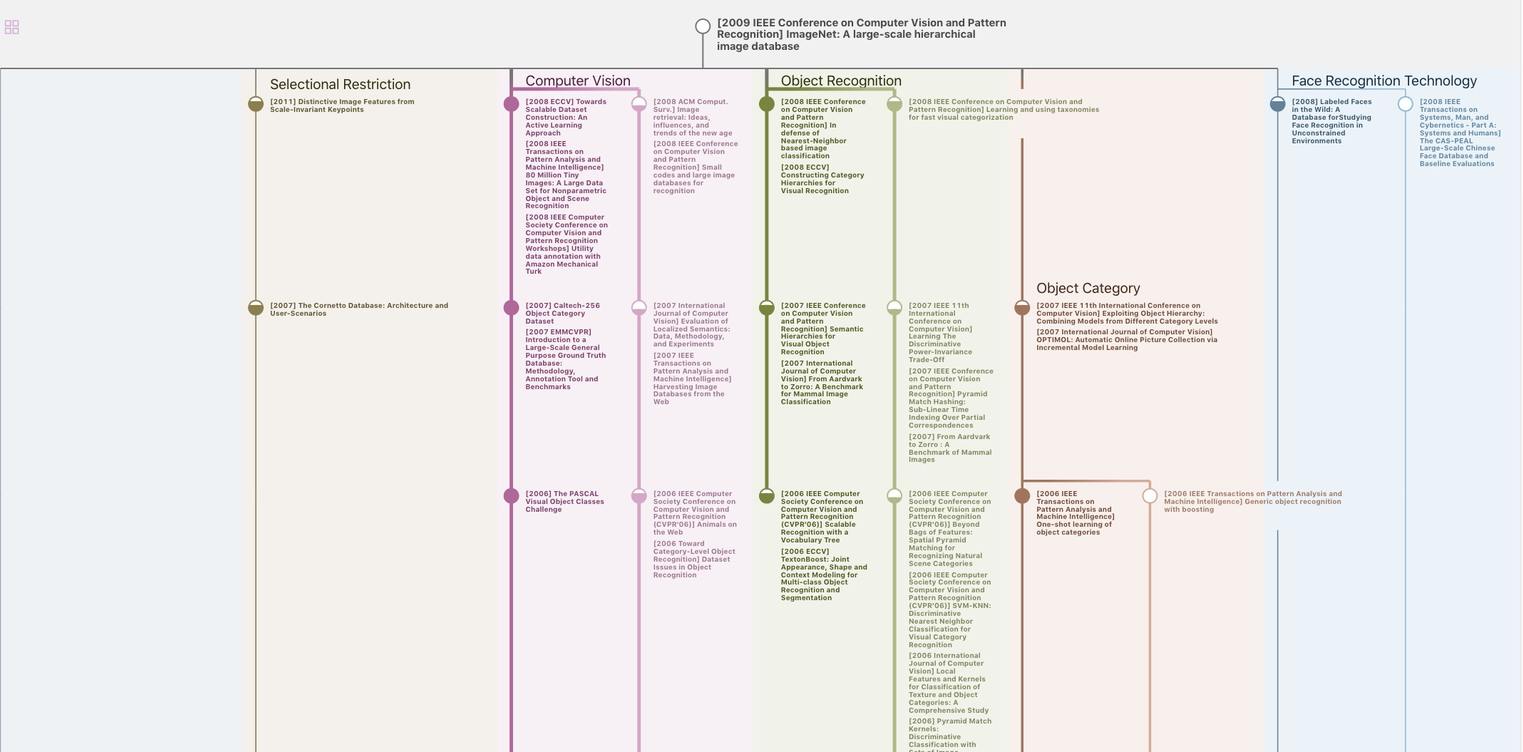
生成溯源树,研究论文发展脉络
Chat Paper
正在生成论文摘要