Generation of synthetic training data for SEEG electrodes segmentation
International Journal of Computer Assisted Radiology and Surgery(2022)
摘要
Purpose Stereoelectroencephalography (SEEG) is a minimally invasive surgical procedure, used to locate epileptogenic zones. An accurate identification of the metallic contacts recording the SEEG signal is crucial to ensure effectiveness of the upcoming treatment. However, due to the presence of metal, post-operative CT scans contain strong streak artefacts that interfere with deep learning segmentation algorithms and require a lot of training data to distinguish from actual contacts. We propose a method to generate synthetic data and use them to train a neural network to precisely locate SEEG electrode contacts. Methods Random electrodes were generated following manufacturer’s specifications and dimensions and placed in acceptable regions inside metal-free CT images. Metal artefacts were simulated in the generated data set using radon transform, beam hardening, and filtered back projection. A UNet neural network was trained for the contacts segmentation task using various training set-ups combining real data, basic augmented data, and synthetic data. The results were compared. Results We reported a higher accuracy when including synthetic data during the network training, while training only on real and basic augmented data more often led to misclassified artefacts or missed contacts. The network segments post-operative CT slices in less than 2 s using 4 GeForce RTX2080 Ti GPUs and in under a minute using a standard PC with GeForce GTX1060. Conclusion Using synthetic data to train the network significantly improves contact detection and segmentation accuracy.
更多查看译文
关键词
Stereoelectroencephalography, Epilepsy, Data augmentation, Segmentation, Sinogram, Radon transform
AI 理解论文
溯源树
样例
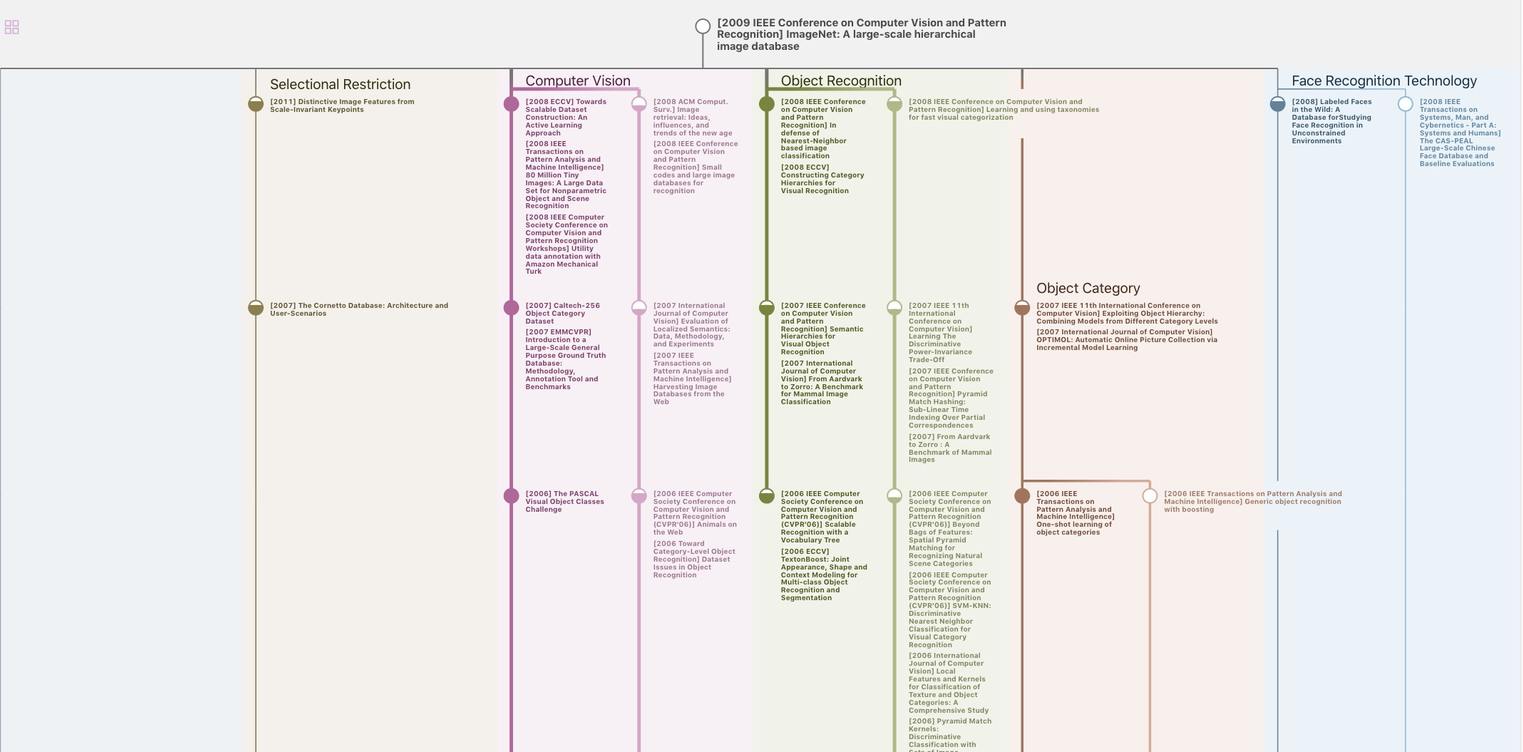
生成溯源树,研究论文发展脉络
Chat Paper
正在生成论文摘要