Leveraging Labeling Representations in Uncertainty-Based Semi-supervised Segmentation
MEDICAL IMAGE COMPUTING AND COMPUTER ASSISTED INTERVENTION, MICCAI 2022, PT VIII(2022)
摘要
Semi-supervised segmentation tackles the scarcity of annotations by leveraging unlabeled data with a small amount of labeled data. A prominent way to utilize the unlabeled data is by consistency training which commonly uses a teacher-student network, where a teacher guides a student segmentation. The predictions of unlabeled data are not reliable, therefore, uncertainty-aware methods have been proposed to gradually learn from meaningful and reliable predictions. Uncertainty estimation, however, relies on multiple inferences from model predictions that need to be computed for each training step, which is computationally expensive. This work proposes a novel method to estimate the pixel-level uncertainty by leveraging the labeling representation of segmentation masks. On the one hand, a labeling representation is learnt to represent the available segmentation masks. The learnt labeling representation is used to map the prediction of the segmentation into a set of plausible masks. Such a reconstructed segmentation mask aids in estimating the pixel-level uncertainty guiding the segmentation network. The proposed method estimates the uncertainty with a single inference from the labeling representation, thereby reducing the total computation. We evaluate our method on the 3D segmentation of left atrium in MRI, and we show that our uncertainty estimates from our labeling representation improve the segmentation accuracy over state-of-the-art methods. Code is released at GitHub.
更多查看译文
关键词
Semi-supervised learning, Segmentation, Labeling representation, Uncertainty
AI 理解论文
溯源树
样例
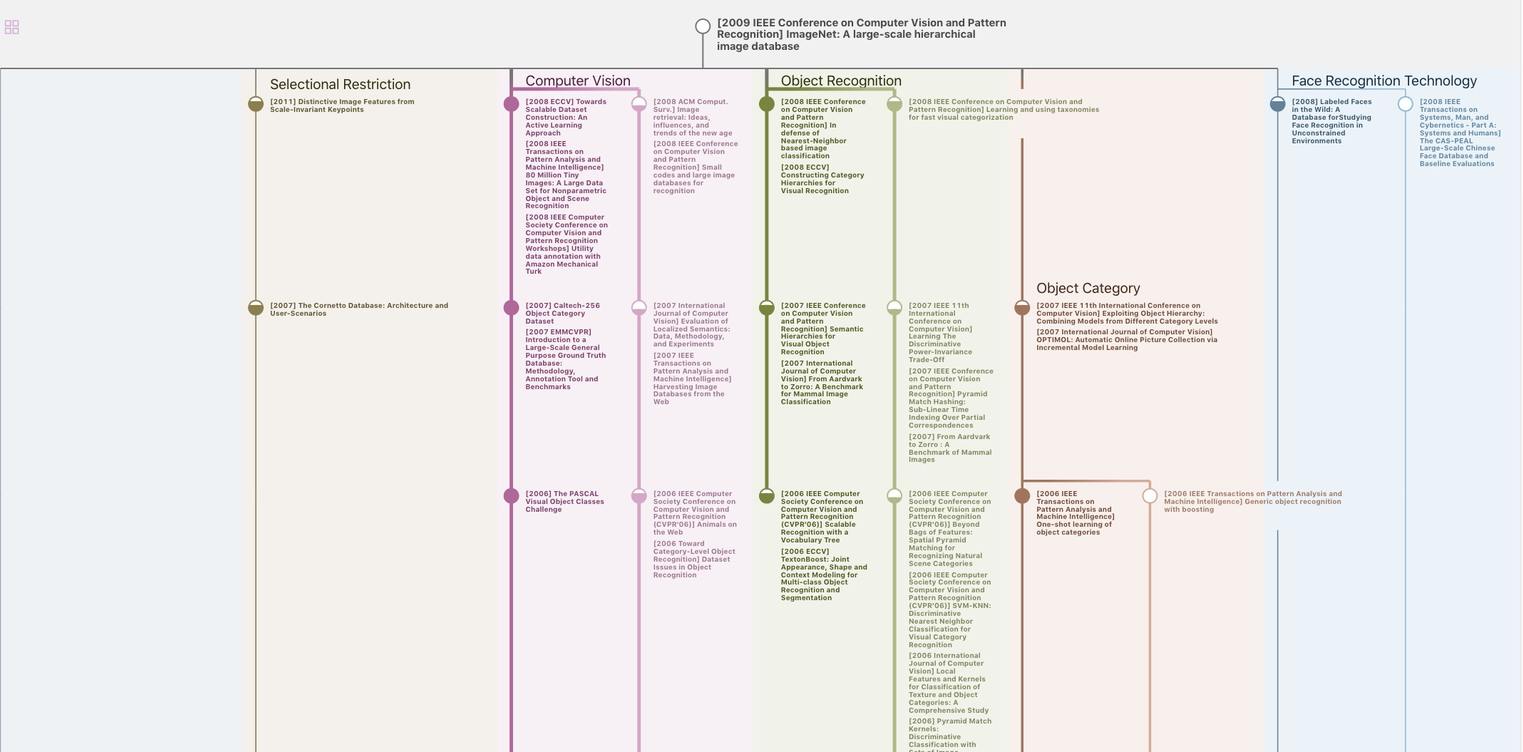
生成溯源树,研究论文发展脉络
Chat Paper
正在生成论文摘要