A Flexible Rolling Regression Framework for the Identification of Time-Varying SIRD Models
Computers & industrial engineering(2022)
摘要
The present paper introduces a rolling regression approach to fitting the time-varying parameters of a susceptible-infected-recovered-deceased (SIRD) model. Our approach provides a domain-aware optimization environment applicable to any epidemiology compartmental model that is linear with respect to those parameters. Our framework is presented as a mixed integer bilevel nonlinear programming problem that is tackled with a real-valued genetic algorithm where we optimize the window size used for regression and estimate the hidden population of infected, recovered, and dead individuals that is not captured by the data available. We test our optimization framework with 2020 COVID-19 data from the state of Minnesota and compare our results both quantitatively and qualitatively, concluding that they are consistent with previously published research. Finally, we compare our proposed method against one of our benchmarks and show that the flexibility of our approach offers better system identification, thus highlighting the importance of domain-aware models that can infer the size of hidden populations from the recorded data and with the aid of equations that describe efficiently the dynamics of an epidemic.
更多查看译文
关键词
Bilevel optimization,Epidemiology models,COVID-19,Rolling regression,Metaheuristics
AI 理解论文
溯源树
样例
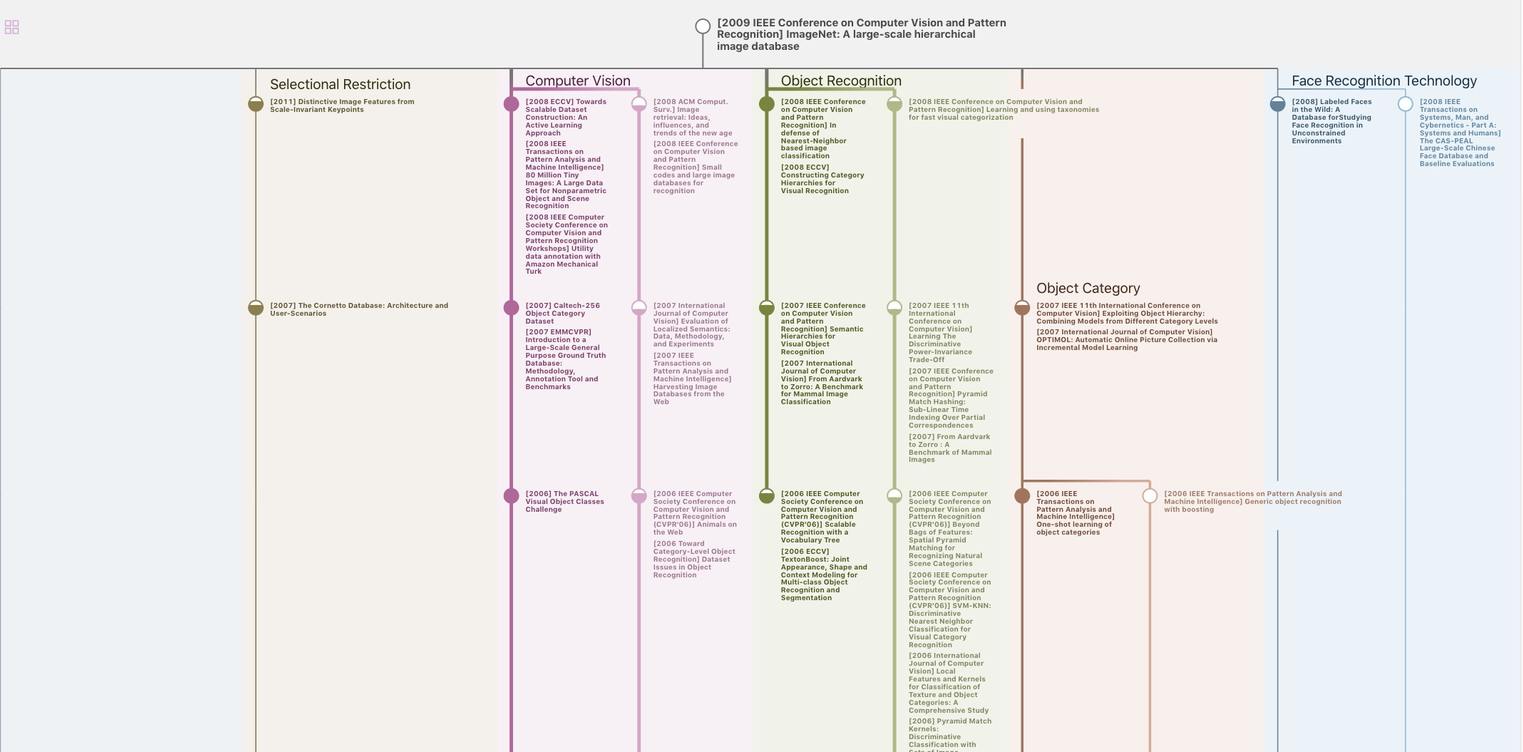
生成溯源树,研究论文发展脉络
Chat Paper
正在生成论文摘要