An explainable XGBoost model improved by SMOTE-ENN technique for maize lodging detection based on multi-source unmanned aerial vehicle images
COMPUTERS AND ELECTRONICS IN AGRICULTURE(2022)
摘要
Remote sensing image is becoming an increasingly popular tool for crop lodging detection because it conveniently provides features for building machine learning models and predicting lodging. However, difficulties in interpreting machine learning models and their predictions limit the confidence of using remote sensing images to detect lodging. In addition, the lodging datasets used for modeling are difficult to balance under natural conditions. Designing a robust and interpretable classification model for the detection of lodging in an imbalanced distribution dataset poses a particularly difficult challenge. In this study, visible and multi-spectral images were collected with a UAV to extract relevant features from remote sensing images.In a preliminary step, Synthetic Minority Oversampling Technique (SMOTE) and Edited Nearest Neighbors (ENN) method were used to treat imbalanced datasets. The SMOTE-ENN-XGBoost model is proposed for the efficient identification of maize lodging at the plot scale. The SMOTE-ENN-XGBoost model achieved an F1-score of 0.930 and a recall of 0.899 on a testing set, suggesting that it can be used for modeling lodging detection. Additionally, the SHapley Additive exPlanations (SHAP) approach was employed to interpret the identification and prioritization of features that determine lodging classification and activity prediction. The results showed that canopy structure and textural features are relatively stable compared with spectral features, which are susceptible to the external environment when modeling is employed to detect lodging. This work also showed that canopy structural, spectral, and textural information should be considered simultaneously rather than separately when detecting crop lodging in a crop breeding program in order to prevent differences in expression controlled by the interaction between genotype and environment obscuring the change in a single feature before and after lodging. For practical applications of machine learning models in crop lodging detection, such insights are of critical relevance. Taken together, the results of this study encourage further applications of remote sensing techniques to build interpretable machine learning models.
更多查看译文
关键词
Lodging,XGBoost,SHAP,Remote sensing,SMOTE
AI 理解论文
溯源树
样例
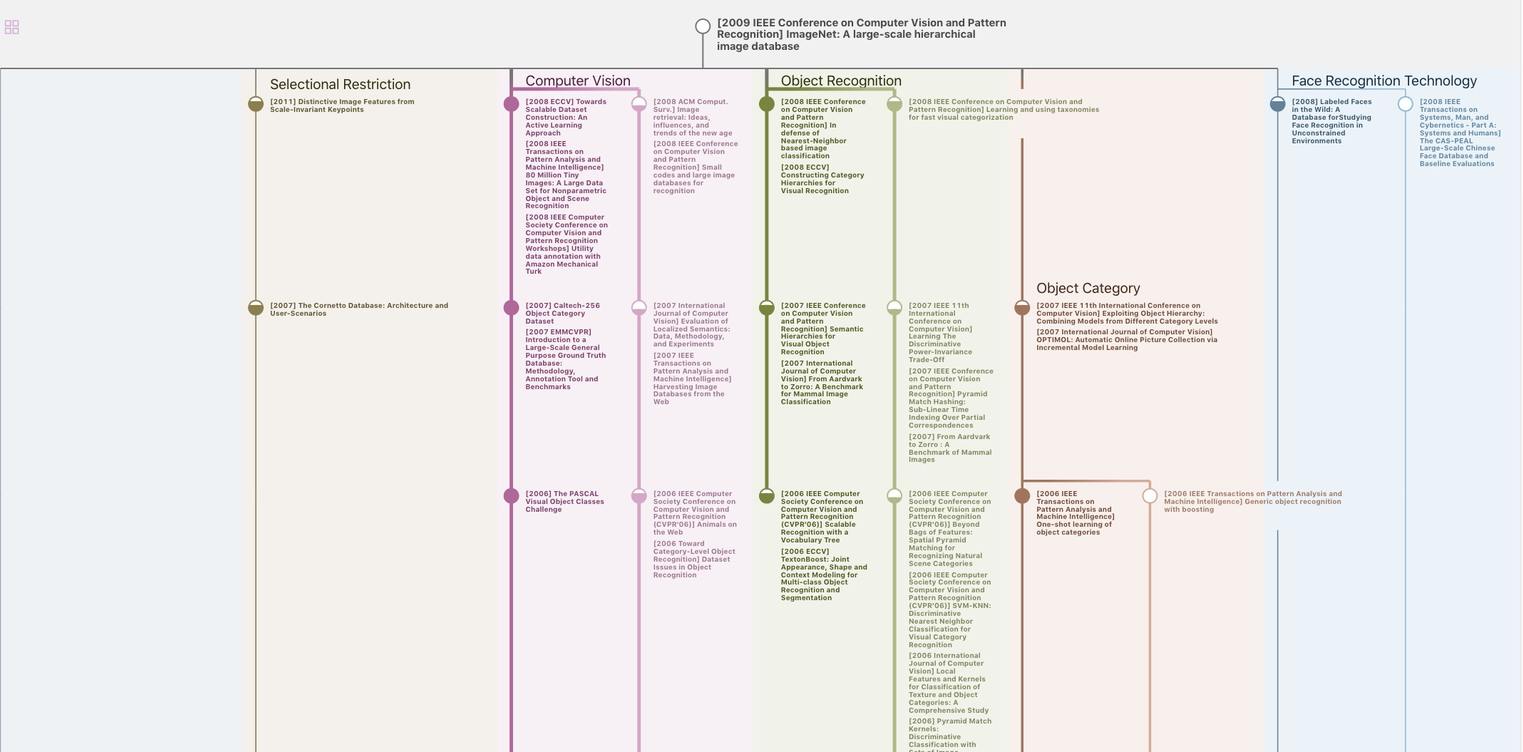
生成溯源树,研究论文发展脉络
Chat Paper
正在生成论文摘要