A hybrid cooperative differential evolution assisted by CMA-ES with local search mechanism
Neural Computing and Applications(2022)
摘要
In this paper, a hybrid cooperative differential evolution with the perturbation of the Covariance Matrix Adaptation Evolutionary Strategy (CMA-ES) with the local search of Limited-Memory Broyden–Fletcher–Goldfarb–Shanno (LBFGS) mechanism, named jSO_CMA-ES_LBFGS, is proposed to solve the complex continuous problems. In the proposed algorithm, jSO, as a variant of Differential Evolution (DE), is used as a global search operator to explore the entire solution space. When the population falls into stagnation, a relatively reliable initial solution for the local search operator is generated by the CMA-ES, which is activated to perturb the optimal candidates in the solution space. The LBFGS utilized as the local search strategy is embedded in CMA-ES to obtain the potential local optimal solutions. A cooperative co-evolutionary dynamic system is formed by jSO and CMA-ES with a local search operator. The proposed jSO_CMA-ES_LBFGS is tested on the CEC2017 benchmark test suite and compared with eleven state-of-the-art algorithms. Further, two practical engineering problems are investigated utilizing the proposed method. The experimental results reveal the effectiveness and efficiency of the jSO_CMA-ES_LBFGS.
更多查看译文
关键词
Differential Evolution (DE), Covariance Matrix Adaptation Evolutionary Strategy (CMA-ES), Limited-Memory Broyden–Fletcher–Goldfarb–Shanno (LBFGS), Cooperative coevolution, Numerical optimization
AI 理解论文
溯源树
样例
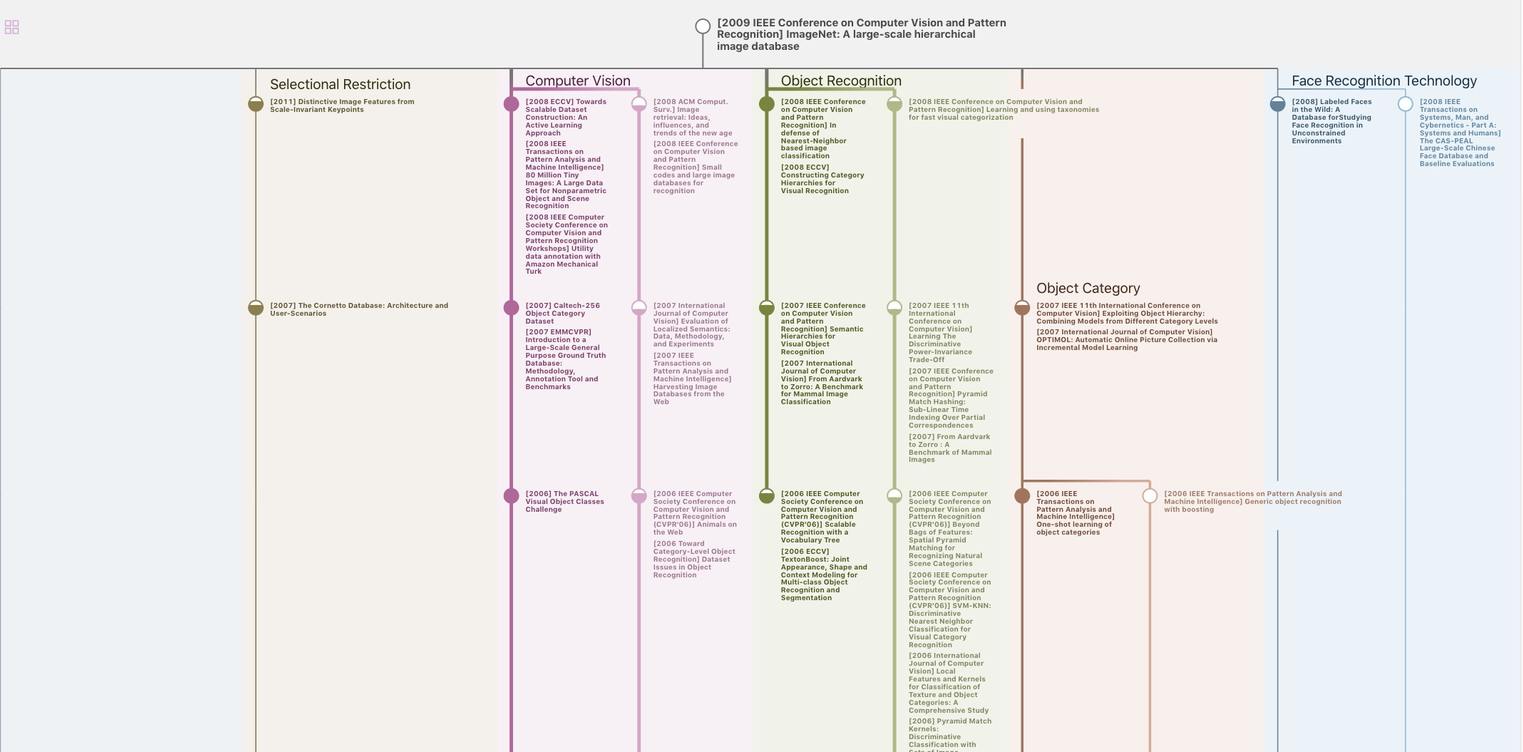
生成溯源树,研究论文发展脉络
Chat Paper
正在生成论文摘要