VersaT2I: Improving Text-to-Image Models with Versatile Reward
arXiv (Cornell University)(2024)
Zhejiang University | University of Washington | Hong Kong University of Science and Technology (GZ | Fudan University
Abstract
Recent text-to-image (T2I) models have benefited from large-scale andhigh-quality data, demonstrating impressive performance. However, these T2Imodels still struggle to produce images that are aesthetically pleasing,geometrically accurate, faithful to text, and of good low-level quality. Wepresent VersaT2I, a versatile training framework that can boost the performancewith multiple rewards of any T2I model. We decompose the quality of the imageinto several aspects such as aesthetics, text-image alignment, geometry,low-level quality, etc. Then, for every quality aspect, we select high-qualityimages in this aspect generated by the model as the training set to finetunethe T2I model using the Low-Rank Adaptation (LoRA). Furthermore, we introduce agating function to combine multiple quality aspects, which can avoid conflictsbetween different quality aspects. Our method is easy to extend and does notrequire any manual annotation, reinforcement learning, or model architecturechanges. Extensive experiments demonstrate that VersaT2I outperforms thebaseline methods across various quality criteria.
MoreTranslated text
PDF
View via Publisher
AI Read Science
AI Summary
AI Summary is the key point extracted automatically understanding the full text of the paper, including the background, methods, results, conclusions, icons and other key content, so that you can get the outline of the paper at a glance.
Example
Background
Key content
Introduction
Methods
Results
Related work
Fund
Key content
- Pretraining has recently greatly promoted the development of natural language processing (NLP)
- We show that M6 outperforms the baselines in multimodal downstream tasks, and the large M6 with 10 parameters can reach a better performance
- We propose a method called M6 that is able to process information of multiple modalities and perform both single-modal and cross-modal understanding and generation
- The model is scaled to large model with 10 billion parameters with sophisticated deployment, and the 10 -parameter M6-large is the largest pretrained model in Chinese
- Experimental results show that our proposed M6 outperforms the baseline in a number of downstream tasks concerning both single modality and multiple modalities We will continue the pretraining of extremely large models by increasing data to explore the limit of its performance
Try using models to generate summary,it takes about 60s
Must-Reading Tree
Example
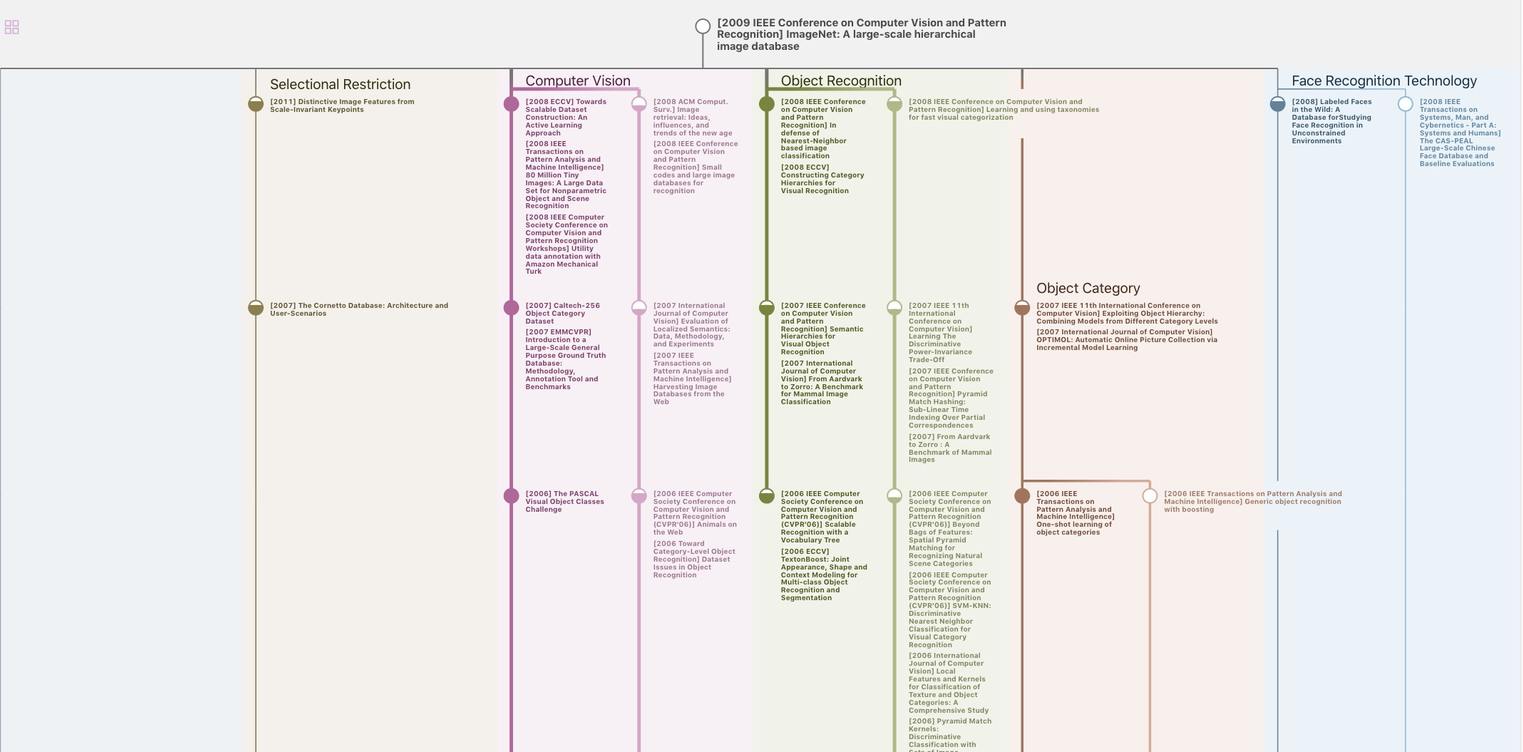
Generate MRT to find the research sequence of this paper
Related Papers
2022
被引用139 | 浏览
2022
被引用8 | 浏览
2024
被引用12 | 浏览
2023
被引用75 | 浏览
2023
被引用4 | 浏览
2023
被引用57 | 浏览
2023
被引用3 | 浏览
2024
被引用82 | 浏览
2023
被引用34 | 浏览
Data Disclaimer
The page data are from open Internet sources, cooperative publishers and automatic analysis results through AI technology. We do not make any commitments and guarantees for the validity, accuracy, correctness, reliability, completeness and timeliness of the page data. If you have any questions, please contact us by email: report@aminer.cn
Chat Paper
去 AI 文献库 对话