Data-driven generalized minimum variance regulatory control using routine operation data
Asian Journal of Control(2023)
摘要
This paper provides a new generalized minimum variance (GMV) control using routine operation data. The proposed method achieves disturbance rejection without additional experiments and reference models, which is different from other data-driven techniques. In this paper, a new data-driven criterion is proposed for the Box and Jenkins (BJ) model, which is a more general description including the Auto-Regressive and Moving Average eXogeneous (ARMAX) model. The paper proves that the optimization of the proposed criterion can achieve GMV control. Numerical examples for two different model structures show the validity of the proposed method. In particular, the application to datasets obtained from a continuous stirred tank reactor (CSTR) demonstrates the efficiency of the proposed method.
更多查看译文
关键词
data-driven controller design,generalized minimum variance control,regulatory control
AI 理解论文
溯源树
样例
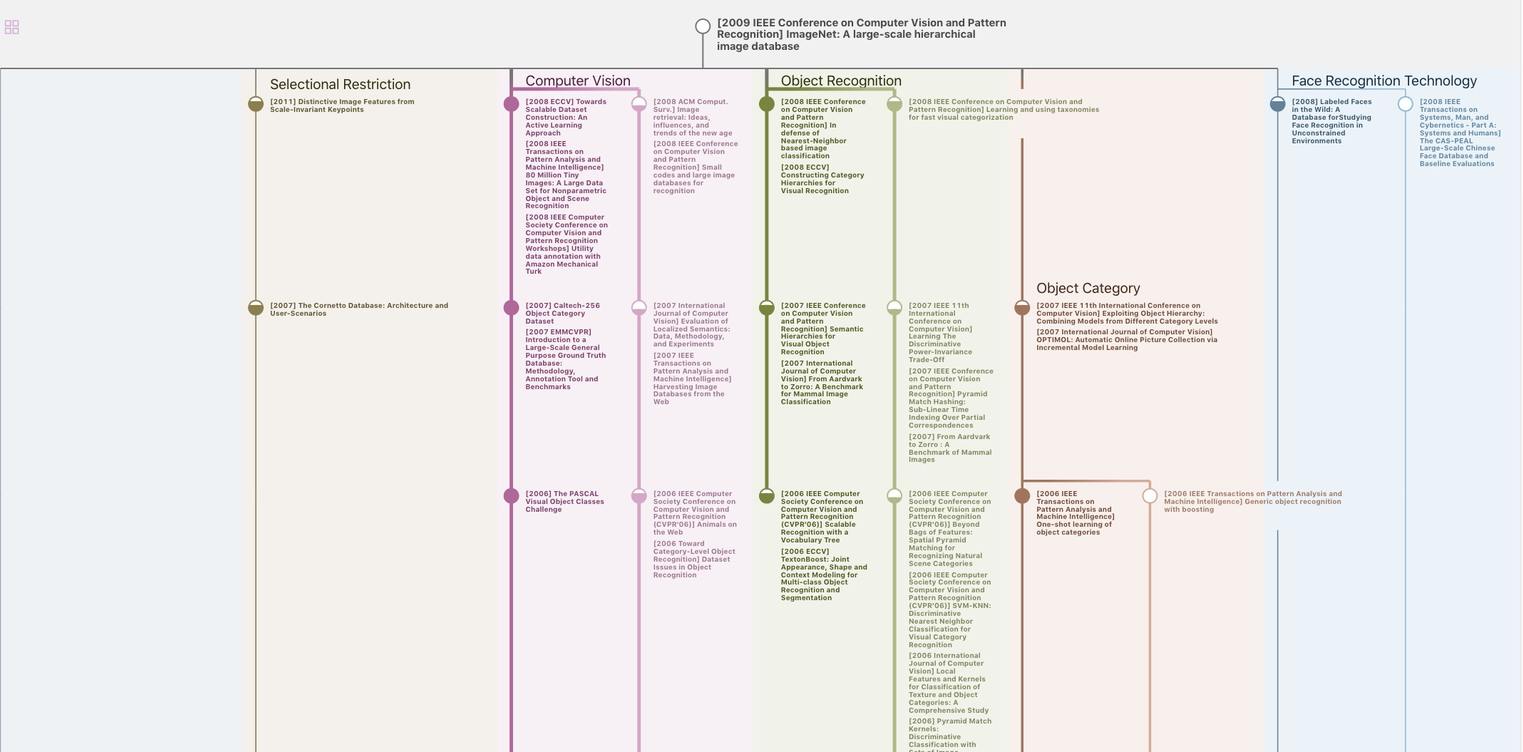
生成溯源树,研究论文发展脉络
Chat Paper
正在生成论文摘要