A probabilistic data analytics methodology based on Bayesian Belief network for predicting and understanding breast cancer survival
Knowledge-Based Systems(2022)
摘要
Understanding breast cancer survival has proven to be a challenging problem for practitioners and researchers. Identifying the factors affecting cancer progression, their interrelationships, and their influence on patients’ long-term survival helps make timely treatment decisions. The current study addresses this problem by proposing a Tree-Augmented Bayesian Belief Network (TAN)-based data analytics methodology comprising of four steps: data acquisition and preprocessing, variable selection via Genetic Algorithm (GA), data balancing with synthetic minority over-sampling and random under-sampling methods, and finally the development of the TAN model to determine the probabilistic inter-conditional dependency structure among breast cancer-related variables along with the posterior survival probabilities The proposed model is compared to well-known machine learning models. A what-if analysis has also been conducted to verify the associations among the variables in the TAN model. The relative importance of each variable has been investigated via sensitivity analysis. Finally, a decision support tool is developed to further explore the conditional dependency structure among the cancer-related factors. The results produced by the proposed methodology, namely the patient-specific posterior survival probabilities and the conditional relationships among the variables, can be used by healthcare professionals and physicians to improve the decision-making process in planning and managing breast cancer treatments. Our generic methodology can also accommodate other types of cancer and be applied to manage various medical procedures.
更多查看译文
关键词
Breast cancer,Data mining,Genetic Algorithm,Machine learning,Sensitivity Analysis
AI 理解论文
溯源树
样例
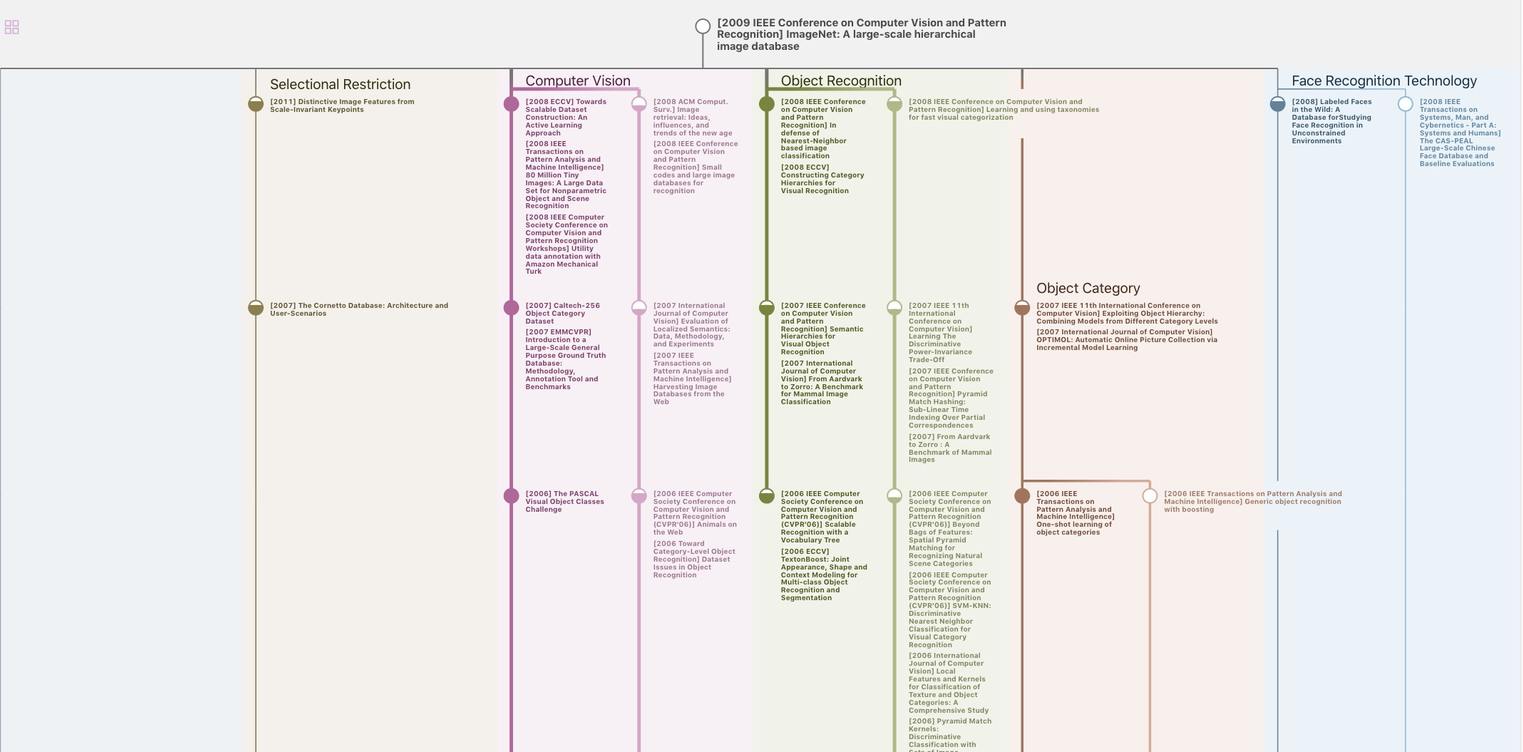
生成溯源树,研究论文发展脉络
Chat Paper
正在生成论文摘要