Assessing Feature Representations for Instance-Based Cross-Domain Anomaly Detection in Cloud Services Univariate Time Series Data
IoT(2022)
摘要
In this paper, we compare and assess the efficacy of a number of time-series instance feature representations for anomaly detection. To assess whether there are statistically significant differences between different feature representations for anomaly detection in a time series, we calculate and compare confidence intervals on the average performance of different feature sets across a number of different model types and cross-domain time-series datasets. Our results indicate that the catch22 time-series feature set augmented with features based on rolling mean and variance performs best on average, and that the difference in performance between this feature set and the next best feature set is statistically significant. Furthermore, our analysis of the features used by the most successful model indicates that features related to mean and variance are the most informative for anomaly detection. We also find that features based on model forecast errors are useful for anomaly detection for some but not all datasets.
更多查看译文
关键词
time series analysis,anomaly detection,data representation,cloud monitoring,AIOPS
AI 理解论文
溯源树
样例
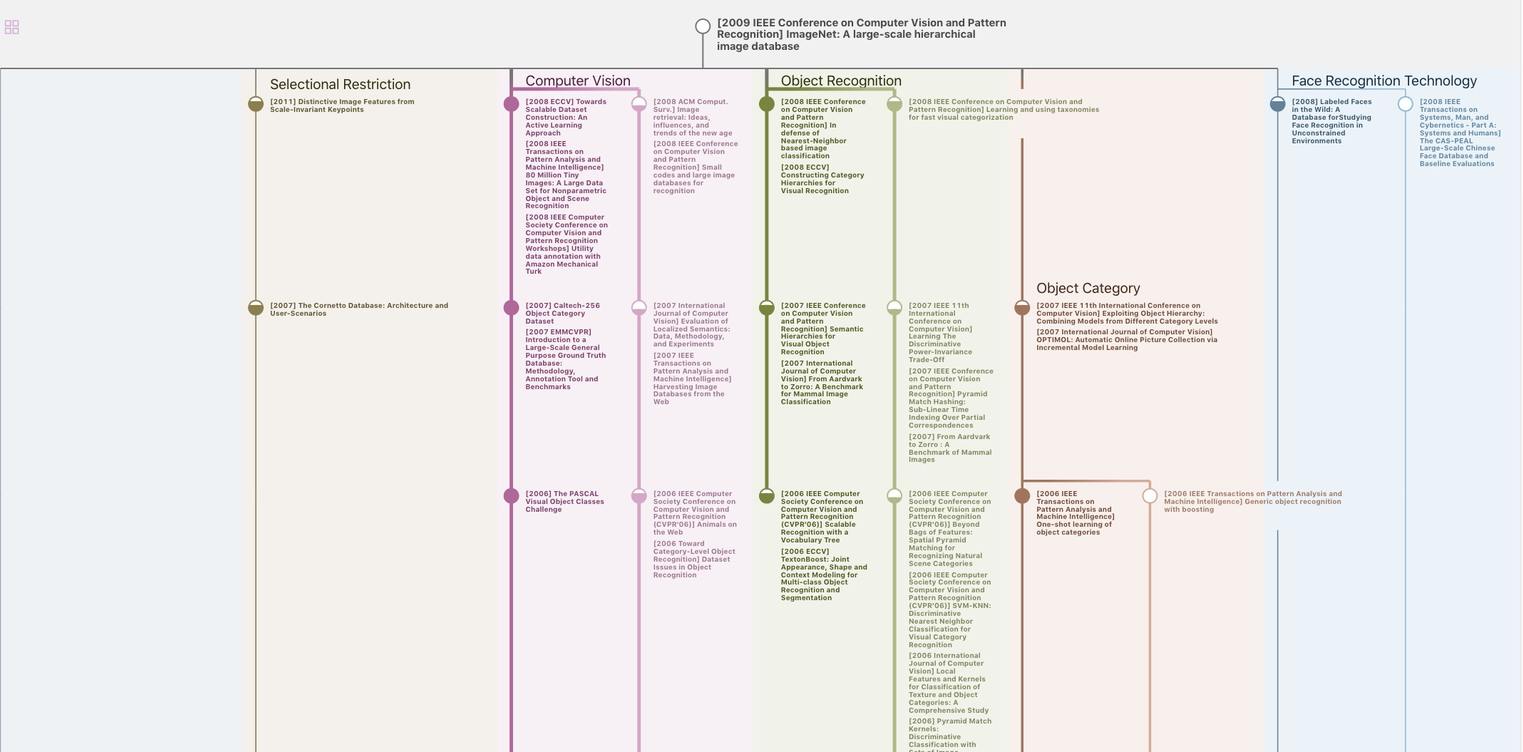
生成溯源树,研究论文发展脉络
Chat Paper
正在生成论文摘要